Projects Category: NLP
- Home
- NLP
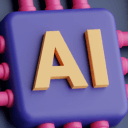
Cloud cost management has evolved from manual spreadsheet tracking to sophisticated AI-driven automation. As organizations increasingly adopt multi-cloud strategies, the complexity of cost optimization grows exponentially. This comprehensive guide explores building agentic AI systems that autonomously manage cloud costs, leveraging open-source models and cutting-edge AI frameworks.
Understanding Agentic AI Systems
Agentic AI systems represent a paradigm shift from traditional reactive AI to proactive, goal-oriented artificial intelligence. Unlike conventional AI models that respond to queries, agentic systems operate autonomously, making decisions and taking actions to achieve specific objectives.
Core Characteristics of Agentic AI
Autonomy: The system operates independently, making decisions without constant human intervention while respecting predefined boundaries and policies.
Goal-Oriented Behavior: Each agent has clear objectives, such as minimizing cloud costs while maintaining performance SLAs, and continuously works toward achieving these goals.
Environmental Awareness: Agents continuously monitor their environment, understanding cloud resource utilization, cost trends, and performance metrics in real-time.
Learning and Adaptation: The system learns from past decisions, improving its cost optimization strategies over time through reinforcement learning and feedback loops.
Multi-Agent Coordination: Different specialized agents collaborate, such as a cost monitoring agent working with a resource optimization agent and a compliance agent.
Architecture of Agentic AI for Cloud Cost Management
Multi-Agent System Design
The architecture consists of specialized agents, each responsible for specific aspects of cloud cost management:
Cost Monitoring Agent: Continuously collects and analyzes billing data from multiple cloud providers, detecting anomalies and trends in real-time.
Resource Optimization Agent: Evaluates resource utilization patterns and makes recommendations or autonomous decisions about rightsizing, scaling, and resource allocation.
Compliance Agent: Ensures all cost optimization actions comply with organizational policies, regulatory requirements, and client-specific constraints.
Forecasting Agent: Uses predictive models to anticipate future costs and resource needs, enabling proactive optimization strategies.
Notification Agent: Manages communication with stakeholders, sending alerts, reports, and recommendations through appropriate channels.
Action Execution Agent: Safely implements approved optimization actions across cloud environments, with rollback capabilities for safety.
Data Flow and Integration Layer
The system integrates with multiple data sources and APIs:
Cloud Provider APIs: Direct integration with AWS Cost Explorer, Azure Cost Management, Google Cloud Billing, and other cloud providers’ cost and usage APIs.
Infrastructure Monitoring: Integration with Prometheus, Grafana, Datadog, or New Relic for real-time performance metrics.
Configuration Management: Connection to Terraform, Ansible, or CloudFormation for infrastructure state management.
Business Systems: Integration with ERP, CRM, and project management systems for cost allocation and chargeback functionality.
Open Source AI Models and Frameworks
Large Language Models Integration
Ollama Integration: Ollama provides local deployment of open-source models, ensuring data privacy and reducing API costs. Key models include:
- Llama 2/3: Excellent for natural language processing tasks, generating cost optimization reports, and explaining complex cost patterns to stakeholders
- Code Llama: Specialized for generating and analyzing infrastructure code, automating Terraform configurations, and creating cost optimization scripts
- Mistral 7B/8x7B: Efficient models for real-time decision making and cost analysis with lower computational requirements
Anthropic Claude Integration: While not open-source, Claude’s API provides sophisticated reasoning capabilities for complex cost optimization scenarios and policy interpretation.
Mistral AI Models: Open-weight models offering excellent performance for cost analysis tasks:
- Mixtral 8x7B: Mixture of experts model providing efficient processing for multi-task cost optimization
- Mistral 7B: Compact model suitable for edge deployment and real-time decision making
Specialized AI Tools and Libraries
Machine Learning Frameworks:
- scikit-learn: For traditional ML tasks like anomaly detection, clustering cost patterns, and regression analysis
- XGBoost/LightGBM: Gradient boosting for accurate cost forecasting and resource usage prediction
- PyTorch/TensorFlow: Deep learning frameworks for complex pattern recognition in cost data
Time Series Analysis:
- Prophet: Facebook’s time series forecasting tool, excellent for predicting cloud costs with seasonal patterns
- ARIMA/SARIMA: Classical time series models for cost trend analysis
- Neural Prophet: Deep learning approach to time series forecasting
Reinforcement Learning:
- Stable Baselines3: Implementation of RL algorithms for autonomous cost optimization decisions
- Ray RLlib: Distributed reinforcement learning for complex multi-agent scenarios
Natural Language Processing:
- Transformers (Hugging Face): Pre-trained models for processing cost reports, policy documents, and generating explanations
- spaCy: Efficient NLP library for text processing and entity extraction from cost documentation
Technical Implementation Stack
Backend Infrastructure
Core Application Framework:
# FastAPI-based microservices architecture
from fastapi import FastAPI, BackgroundTasks
from pydantic import BaseModel
import asyncio
from typing import List, Dict
import httpx
app = FastAPI(title="Agentic Cloud Cost Management")
class CostAgent:
def __init__(self, model_endpoint: str):
self.model_endpoint = model_endpoint
self.client = httpx.AsyncClient()
async def analyze_costs(self, cost_data: Dict):
# Integration with Ollama or other model endpoints
pass
Message Queue and Orchestration:
- Apache Kafka: Real-time data streaming for cost events and optimization triggers
- Celery with Redis: Task queue for asynchronous cost optimization jobs
- Apache Airflow: Workflow orchestration for complex cost management pipelines
Database Architecture:
- InfluxDB: Time-series database for storing cost and usage metrics
- PostgreSQL: Relational database for client configurations, policies, and audit trails
- MongoDB: Document store for unstructured data like cost reports and optimization recommendations
- Redis: Caching layer for frequently accessed cost data and model predictions
AI Model Deployment and Management
Local Model Deployment with Ollama:
# Install and configure Ollama
curl -fsSL https://ollama.ai/install.sh | sh
# Pull required models
ollama pull llama3
ollama pull mistral
ollama pull codellama
# Create custom cost management model
ollama create cost-optimizer -f ./Modelfile
Model Serving Infrastructure:
- TorchServe: Production-ready serving for PyTorch models
- MLflow: Model versioning, experiment tracking, and deployment
- Kubeflow: Kubernetes-native ML workflows
- BentoML: Framework for building AI application APIs
Container Orchestration:
# Kubernetes deployment for AI agents
apiVersion: apps/v1
kind: Deployment
metadata:
name: cost-optimization-agent
spec:
replicas: 3
selector:
matchLabels:
app: cost-agent
template:
metadata:
labels:
app: cost-agent
spec:
containers:
- name: cost-agent
image: cost-optimizer:latest
resources:
requests:
memory: "2Gi"
cpu: "1000m"
limits:
memory: "4Gi"
cpu: "2000m"
Cloud Provider Integration
Multi-Cloud Cost Collection:
import boto3
from azure.mgmt.consumption import ConsumptionManagementClient
from google.cloud import billing
import asyncio
class MultiCloudCostCollector:
def __init__(self):
self.aws_client = boto3.client('ce') # Cost Explorer
self.azure_client = ConsumptionManagementClient(credential, subscription_id)
self.gcp_client = billing.CloudBillingClient()
async def collect_all_costs(self):
tasks = [
self.get_aws_costs(),
self.get_azure_costs(),
self.get_gcp_costs()
]
return await asyncio.gather(*tasks)
Infrastructure as Code Integration:
- Terraform Provider: Custom provider for cost-optimized resource provisioning
- Pulumi: Modern IaC with native programming language support
- CDK (Cloud Development Kit): Define cloud resources using familiar programming languages
Agent Communication and Coordination
Inter-Agent Communication Protocol
Message Passing System:
from dataclasses import dataclass
from enum import Enum
from typing import Any, Dict
class MessageType(Enum):
COST_ALERT = "cost_alert"
OPTIMIZATION_REQUEST = "optimization_request"
ACTION_APPROVAL = "action_approval"
STATUS_UPDATE = "status_update"
@dataclass
class AgentMessage:
sender: str
recipient: str
message_type: MessageType
payload: Dict[str, Any]
timestamp: float
priority: int = 1
class AgentCommunicationHub:
def __init__(self):
self.agents = {}
self.message_queue = asyncio.Queue()
async def route_message(self, message: AgentMessage):
if message.recipient in self.agents:
await self.agents[message.recipient].receive_message(message)
Consensus Mechanisms: Implement voting systems for critical decisions affecting multiple clients or significant cost impacts, ensuring no single agent can make potentially harmful decisions without consensus.
Event-Driven Architecture
Event Streaming with Kafka:
from kafka import KafkaProducer, KafkaConsumer
import json
class CostEventProducer:
def __init__(self):
self.producer = KafkaProducer(
bootstrap_servers=['localhost:9092'],
value_serializer=lambda x: json.dumps(x).encode('utf-8')
)
def emit_cost_event(self, event_type: str, data: Dict):
event = {
'event_type': event_type,
'timestamp': time.time(),
'data': data
}
self.producer.send('cost-events', event)
class OptimizationAgent:
def __init__(self):
self.consumer = KafkaConsumer(
'cost-events',
bootstrap_servers=['localhost:9092'],
auto_offset_reset='latest',
value_deserializer=lambda m: json.loads(m.decode('utf-8'))
)
async def process_events(self):
for message in self.consumer:
await self.handle_cost_event(message.value)
Implementing Intelligent Cost Optimization
Anomaly Detection System
Statistical Anomaly Detection:
import numpy as np
from sklearn.ensemble import IsolationForest
from sklearn.preprocessing import StandardScaler
class CostAnomalyDetector:
def __init__(self):
self.model = IsolationForest(contamination=0.1, random_state=42)
self.scaler = StandardScaler()
self.is_trained = False
def train(self, historical_costs: np.ndarray):
scaled_costs = self.scaler.fit_transform(historical_costs)
self.model.fit(scaled_costs)
self.is_trained = True
def detect_anomalies(self, current_costs: np.ndarray) -> List[bool]:
if not self.is_trained:
raise ValueError("Model must be trained first")
scaled_costs = self.scaler.transform(current_costs)
anomaly_scores = self.model.decision_function(scaled_costs)
return self.model.predict(scaled_costs) == -1
Deep Learning Anomaly Detection:
import torch
import torch.nn as nn
from torch.utils.data import DataLoader, TensorDataset
class CostAutoencoderAnomalyDetector(nn.Module):
def __init__(self, input_dim: int, hidden_dims: List[int]):
super().__init__()
# Encoder
encoder_layers = []
current_dim = input_dim
for hidden_dim in hidden_dims:
encoder_layers.extend([
nn.Linear(current_dim, hidden_dim),
nn.ReLU(),
nn.BatchNorm1d(hidden_dim)
])
current_dim = hidden_dim
# Decoder
decoder_layers = []
for i in range(len(hidden_dims) - 1, -1, -1):
decoder_layers.extend([
nn.Linear(current_dim, hidden_dims[i] if i > 0 else input_dim),
nn.ReLU() if i > 0 else nn.Sigmoid()
])
current_dim = hidden_dims[i] if i > 0 else input_dim
self.encoder = nn.Sequential(*encoder_layers)
self.decoder = nn.Sequential(*decoder_layers)
def forward(self, x):
encoded = self.encoder(x)
decoded = self.decoder(encoded)
return decoded
Predictive Cost Modeling
Time Series Forecasting with Neural Networks:
import pytorch_lightning as pl
from pytorch_forecasting import TimeSeriesDataSet, NBeats
import pandas as pd
class CostForecastingModel:
def __init__(self):
self.model = None
self.training_data = None
def prepare_data(self, cost_history: pd.DataFrame):
# Convert cost history to time series format
self.training_data = TimeSeriesDataSet(
cost_history,
time_idx="day",
target="cost",
group_ids=["client_id", "service"],
min_encoder_length=30,
max_encoder_length=90,
min_prediction_length=7,
max_prediction_length=30,
static_categoricals=["client_id", "service"],
time_varying_known_reals=["day_of_week", "month", "quarter"],
time_varying_unknown_reals=["cost"],
)
def train_model(self):
self.model = NBeats.from_dataset(
self.training_data,
learning_rate=3e-2,
weight_decay=1e-8,
widths=[32, 512],
backcast_loss_ratio=1.0,
)
trainer = pl.Trainer(max_epochs=50, gpus=1)
trainer.fit(self.model, self.training_data)
Reinforcement Learning for Cost Optimization
Deep Q-Network for Resource Allocation:
import gym
import torch
import torch.nn as nn
import numpy as np
from collections import deque
import random
class CloudCostEnvironment(gym.Env):
def __init__(self, client_config: Dict):
super().__init__()
self.client_config = client_config
self.action_space = gym.spaces.Discrete(5) # Scale up, down, maintain, stop, start
self.observation_space = gym.spaces.Box(
low=0, high=np.inf, shape=(10,), dtype=np.float32
)
self.current_cost = 0
self.performance_score = 1.0
def step(self, action):
# Simulate cost and performance changes based on action
cost_change, performance_change = self._simulate_action(action)
self.current_cost += cost_change
self.performance_score += performance_change
# Calculate reward (negative cost + performance bonus)
reward = -cost_change + (performance_change * 10)
# Check if episode is done
done = self.current_cost > self.client_config['max_budget']
obs = self._get_observation()
return obs, reward, done, {}
def _simulate_action(self, action):
# Implementation of cost and performance simulation
pass
class DQNAgent:
def __init__(self, state_size, action_size):
self.state_size = state_size
self.action_size = action_size
self.memory = deque(maxlen=2000)
self.epsilon = 1.0
self.epsilon_min = 0.01
self.epsilon_decay = 0.995
self.learning_rate = 0.001
self.q_network = self._build_model()
self.target_network = self._build_model()
def _build_model(self):
model = nn.Sequential(
nn.Linear(self.state_size, 64),
nn.ReLU(),
nn.Linear(64, 64),
nn.ReLU(),
nn.Linear(64, self.action_size)
)
return model
Multi-Tenant Architecture for Service Organizations
Client Isolation and Security
Tenant Management System:
from sqlalchemy import create_engine, Column, String, Integer, JSON, DateTime
from sqlalchemy.ext.declarative import declarative_base
from sqlalchemy.orm import sessionmaker
import hashlib
import secrets
Base = declarative_base()
class Tenant(Base):
__tablename__ = 'tenants'
id = Column(String, primary_key=True)
name = Column(String, nullable=False)
api_key_hash = Column(String, nullable=False)
config = Column(JSON, default={})
created_at = Column(DateTime)
def generate_api_key(self):
api_key = secrets.token_urlsafe(32)
self.api_key_hash = hashlib.sha256(api_key.encode()).hexdigest()
return api_key
class TenantManager:
def __init__(self, database_url: str):
self.engine = create_engine(database_url)
self.SessionLocal = sessionmaker(bind=self.engine)
def create_tenant(self, name: str, config: Dict) -> Tuple[str, str]:
tenant = Tenant(
id=secrets.token_urlsafe(16),
name=name,
config=config
)
api_key = tenant.generate_api_key()
with self.SessionLocal() as session:
session.add(tenant)
session.commit()
return tenant.id, api_key
Data Isolation with Row-Level Security:
-- PostgreSQL RLS for tenant data isolation
CREATE POLICY tenant_isolation ON cost_data
FOR ALL TO application_role
USING (tenant_id = current_setting('app.current_tenant'));
-- Function to set tenant context
CREATE OR REPLACE FUNCTION set_tenant_context(tenant_id TEXT)
RETURNS VOID AS $$
BEGIN
PERFORM set_config('app.current_tenant', tenant_id, true);
END;
$$ LANGUAGE plpgsql;
Scalable Agent Deployment
Kubernetes Operator for Agent Management:
apiVersion: apiextensions.k8s.io/v1
kind: CustomResourceDefinition
metadata:
name: costagents.ai.company.com
spec:
group: ai.company.com
versions:
- name: v1
served: true
storage: true
schema:
openAPIV3Schema:
type: object
properties:
spec:
type: object
properties:
tenantId:
type: string
agentType:
type: string
enum: ["cost-monitor", "optimizer", "forecaster"]
resources:
type: object
properties:
cpu:
type: string
memory:
type: string
modelConfig:
type: object
scope: Namespaced
names:
plural: costagents
singular: costagent
kind: CostAgent
Advanced AI Integration Patterns
Multi-Model Ensemble Approach
Model Router and Ensemble:
from typing import List, Dict, Any
import numpy as np
from sklearn.metrics import mean_squared_error
class ModelEnsemble:
def __init__(self):
self.models = {}
self.weights = {}
self.performance_history = {}
def add_model(self, name: str, model: Any, weight: float = 1.0):
self.models[name] = model
self.weights[name] = weight
self.performance_history[name] = deque(maxlen=100)
def predict(self, data: Any) -> Dict[str, Any]:
predictions = {}
for name, model in self.models.items():
try:
if name.startswith('ollama_'):
# Handle Ollama model inference
predictions[name] = self._query_ollama_model(model, data)
elif name.startswith('mistral_'):
# Handle Mistral model inference
predictions[name] = self._query_mistral_model(model, data)
else:
# Handle scikit-learn or other models
predictions[name] = model.predict(data)
except Exception as e:
print(f"Model {name} failed: {e}")
continue
# Weight predictions based on recent performance
final_prediction = self._weighted_ensemble(predictions)
return final_prediction
def _weighted_ensemble(self, predictions: Dict) -> Any:
# Implement weighted averaging based on model performance
pass
class IntelligentModelRouter:
def __init__(self):
self.routing_rules = {}
self.model_capabilities = {}
def route_request(self, request_type: str, complexity: float, latency_req: float):
# Route to appropriate model based on request characteristics
if request_type == "cost_analysis" and complexity < 0.5:
return "mistral_7b" # Fast, efficient model
elif request_type == "optimization" and latency_req > 10:
return "llama3_70b" # More capable model when time allows
else:
return "ensemble" # Use ensemble for complex decisions
Real-Time Streaming AI
Streaming Cost Analysis with Kafka Streams:
from kafka import KafkaConsumer, KafkaProducer
import json
import asyncio
from typing import AsyncGenerator
class StreamingCostAnalyzer:
def __init__(self, model_endpoint: str):
self.model_endpoint = model_endpoint
self.consumer = KafkaConsumer(
'cost-events',
bootstrap_servers=['localhost:9092'],
value_deserializer=lambda m: json.loads(m.decode('utf-8'))
)
self.producer = KafkaProducer(
bootstrap_servers=['localhost:9092'],
value_serializer=lambda x: json.dumps(x).encode('utf-8')
)
async def process_cost_stream(self):
async for message in self._async_consume():
# Real-time cost analysis
analysis_result = await self._analyze_cost_event(message.value)
# Emit results
await self._emit_analysis_result(analysis_result)
async def _analyze_cost_event(self, event: Dict) -> Dict:
# Use streaming-optimized model for real-time analysis
prompt = f"Analyze this cost event: {json.dumps(event)}"
response = await self._query_model(prompt)
return {
'event_id': event['id'],
'analysis': response,
'timestamp': time.time(),
'confidence': self._calculate_confidence(response)
}
Monitoring and Observability
Comprehensive Monitoring Stack
Agent Health Monitoring:
from prometheus_client import Counter, Histogram, Gauge, start_http_server
import time
import logging
class AgentMetrics:
def __init__(self):
self.decision_counter = Counter('agent_decisions_total', 'Total decisions made', ['agent_type', 'decision_type'])
self.response_time = Histogram('agent_response_time_seconds', 'Agent response time')
self.active_agents = Gauge('active_agents', 'Number of active agents', ['agent_type'])
self.cost_savings = Counter('cost_savings_total', 'Total cost savings achieved', ['client_id'])
def record_decision(self, agent_type: str, decision_type: str):
self.decision_counter.labels(agent_type=agent_type, decision_type=decision_type).inc()
def record_response_time(self, duration: float):
self.response_time.observe(duration)
# Distributed tracing with OpenTelemetry
from opentelemetry import trace
from opentelemetry.exporter.jaeger.thrift import JaegerExporter
from opentelemetry.sdk.trace import TracerProvider
from opentelemetry.sdk.trace.export import BatchSpanProcessor
tracer = trace.get_tracer(__name__)
class TracedCostAgent:
def __init__(self):
self.metrics = AgentMetrics()
async def make_cost_decision(self, request: Dict):
with tracer.start_as_current_span("cost_decision") as span:
span.set_attribute("client_id", request['client_id'])
span.set_attribute("request_type", request['type'])
start_time = time.time()
try:
result = await self._process_request(request)
span.set_attribute("decision", result['decision'])
return result
finally:
duration = time.time() - start_time
self.metrics.record_response_time(duration)
Automated Testing and Validation
Agent Behavior Testing:
import pytest
from unittest.mock import AsyncMock, patch
import asyncio
class TestCostOptimizationAgent:
@pytest.fixture
async def agent(self):
return CostOptimizationAgent(
model_endpoint="http://localhost:11434",
client_config={"max_cost": 1000, "min_performance": 0.8}
)
@pytest.mark.asyncio
async def test_cost_spike_detection(self, agent):
# Mock cost data with spike
cost_data = {
'current_cost': 1500,
'historical_average': 800,
'client_id': 'test_client'
}
with patch.object(agent, '_query_model') as mock_query:
mock_query.return_value = {
'anomaly_detected': True,
'recommended_action': 'scale_down',
'confidence': 0.95
}
result = await agent.analyze_cost_anomaly(cost_data)
assert result['anomaly_detected'] is True
assert result['recommended_action'] == 'scale_down'
@pytest.mark.asyncio
async def test_multi_agent_coordination(self):
# Test agent communication and coordination
pass
# Load testing for agent scalability
from locust import HttpUser, task, between
class AgentLoadTest(HttpUser):
wait_time = between(1, 3)
@task
def analyze_costs(self):
cost_data = {
'client_id': 'load_test_client',
'costs': [100, 150, 200, 180, 220],
'timestamp': time.time()
}
self.client.post("/api/v1/analyze-costs", json=cost_data)
@task
def get_recommendations(self):
self.client.get("/api/v1/recommendations/load_test_client")
Business Intelligence and Reporting
Automated Report Generation
AI-Powered Cost Reports:
from jinja2 import Template
import matplotlib.pyplot as plt
import pandas as pd
from datetime import datetime, timedelta
class IntelligentReporter:
def __init__(self, llm_client):
self.llm_client = llm_client
self.report_templates = {}
async def generate_executive_summary(self, cost_data: Dict, client_id: str) -> str:
# Use LLM to generate natural language insights
prompt = f"""
Based on the following cost data for client {client_id}, generate an executive summary:
Total Cost: ${cost_data['total_cost']:,.2f}
Month-over-Month Change: {cost_data['mom_change']:.1f}%
Top Cost Drivers: {', '.join(cost_data['top_drivers'])}
Optimization Opportunities: {cost_data['savings_potential']}
Generate a professional executive summary highlighting key insights and recommendations.
"""
response = await self.llm_client.generate(prompt)
return response
async def create_comprehensive_report(self, client_id: str, period: str) -> Dict:
# Gather data from multiple sources
cost_data = await self._fetch_cost_data(client_id, period)
savings_data = await self._fetch_savings_data(client_id, period)
forecast_data = await self._generate_forecast(client_id)
# Generate visualizations
charts = await self._create_charts(cost_data)
# Generate AI insights
executive_summary = await self.generate_executive_summary(cost_data, client_id)
recommendations = await self._generate_recommendations(cost_data, savings_data)
return {
'client_id': client_id,
'period': period,
'executive_summary': executive_summary,
'cost_breakdown': cost_data,
'savings_achieved': savings_data,
'forecast': forecast_data,
'recommendations': recommendations,
'charts': charts,
'generated_at': datetime.now().isoformat()
}
Security and Compliance Framework
AI Model Security
Model Input Validation and Sanitization:
import re
from typing import Any, Dict
import bleach
class SecureModelInterface:
def __init__(self):
self.input_validators = {
'cost_analysis': self._validate_cost_input,
'optimization': self._validate_optimization_input
}
self.max_input_length = 10000
self.allowed_tags = []
def sanitize_input(self, input_data: Any) -> Any:
if isinstance(input_data, str):
# Remove potentially harmful content
sanitized = bleach.clean(input_data, tags=self.allowed_tags, strip=True)
# Validate length
if len(sanitized) > self.max_input_length:
raise ValueError("Input too long")
# Check for injection patterns
if self._detect_injection_patterns(sanitized):
raise ValueError("Potentially malicious input detected")
return sanitized
elif isinstance(input_data, dict):
return {k: self.sanitize_input(v) for k, v in input_data.items()}
return input_data
def _detect_injection_patterns(self, text: str) -> bool:
# Check for common injection patterns
patterns = [
r'<script.*?>.*?</script>',
r'javascript:',
r'data:text/html',
r'vbscript:',
r'onload\s*=',
r'onerror\s*='
]
for pattern in patterns:
if re.search(pattern, text, re.IGNORECASE | re.DOTALL):
return True
return False
# Audit logging for all AI decisions
class AuditLogger:
def __init__(self, log_level: str = "INFO"):
self.logger = logging.getLogger("agent_audit")
self.logger.setLevel(getattr(logging, log_level))
handler = logging.StreamHandler()
formatter = logging.Formatter(
'%(asctime)s - %(name)s - %(levelname)s - %(message)s'
)
handler.setFormatter(formatter)
self.logger.addHandler(handler)
def log_decision(self, agent_id: str, client_id: str, decision: Dict, reasoning: str):
audit_entry = {
'timestamp': datetime.now().isoformat(),
'agent_id': agent_id,
'client_id': client_id,
'decision': decision,
'reasoning': reasoning,
'model_version': self._get_model_version(),
'confidence_score': decision.get('confidence', 0.0)
}
self.logger.info(f"DECISION: {json.dumps(audit_entry)}")
def log_action_taken(self, agent_id: str, client_id: str, action: Dict, result: Dict):
audit_entry = {
'timestamp': datetime.now().isoformat(),
'agent_id': agent_id,
'client_id': client_id,
'action': action,
'result': result,
'success': result.get('success', False)
}
self.logger.info(f"ACTION: {json.dumps(audit_entry)}")
Compliance and Governance
Policy Engine for Cost Management:
from dataclasses import dataclass
from typing import List, Callable, Any
from enum import Enum
class PolicyType(Enum):
COST_LIMIT = "cost_limit"
RESOURCE_CONSTRAINT = "resource_constraint"
APPROVAL_REQUIRED = "approval_required"
COMPLIANCE_RULE = "compliance_rule"
@dataclass
class Policy:
id: str
name: str
policy_type: PolicyType
conditions: Dict[str, Any]
actions: List[str]
priority: int
active: bool = True
class PolicyEngine:
def __init__(self):
self.policies: Dict[str, Policy] = {}
self.rule_evaluators: Dict[PolicyType, Callable] = {
PolicyType.COST_LIMIT: self._evaluate_cost_limit,
PolicyType.RESOURCE_CONSTRAINT: self._evaluate_resource_constraint,
PolicyType.APPROVAL_REQUIRED: self._evaluate_approval_requirement,
PolicyType.COMPLIANCE_RULE: self._evaluate_compliance_rule
}
def add_policy(self, policy: Policy):
self.policies[policy.id] = policy
async def evaluate_action(self, action: Dict, context: Dict) -> Dict:
"""Evaluate if an action is allowed based on active policies"""
evaluation_results = []
# Sort policies by priority
sorted_policies = sorted(
[p for p in self.policies.values() if p.active],
key=lambda x: x.priority,
reverse=True
)
for policy in sorted_policies:
evaluator = self.rule_evaluators.get(policy.policy_type)
if evaluator:
result = await evaluator(policy, action, context)
evaluation_results.append({
'policy_id': policy.id,
'policy_name': policy.name,
'allowed': result['allowed'],
'reason': result['reason'],
'required_approvals': result.get('required_approvals', [])
})
# Determine final decision
allowed = all(result['allowed'] for result in evaluation_results)
required_approvals = []
for result in evaluation_results:
required_approvals.extend(result.get('required_approvals', []))
return {
'allowed': allowed,
'policy_evaluations': evaluation_results,
'required_approvals': list(set(required_approvals))
}
async def _evaluate_cost_limit(self, policy: Policy, action: Dict, context: Dict) -> Dict:
current_cost = context.get('current_monthly_cost', 0)
projected_cost = current_cost + action.get('cost_impact', 0)
cost_limit = policy.conditions.get('monthly_limit', float('inf'))
if projected_cost > cost_limit:
return {
'allowed': False,
'reason': f"Action would exceed monthly cost limit of ${cost_limit:,.2f}"
}
return {'allowed': True, 'reason': 'Within cost limits'}
async def _evaluate_resource_constraint(self, policy: Policy, action: Dict, context: Dict) -> Dict:
# Implement resource constraint evaluation
return {'allowed': True, 'reason': 'Resource constraints satisfied'}
async def _evaluate_approval_requirement(self, policy: Policy, action: Dict, context: Dict) -> Dict:
cost_impact = action.get('cost_impact', 0)
approval_threshold = policy.conditions.get('cost_threshold', 1000)
if abs(cost_impact) > approval_threshold:
return {
'allowed': False,
'reason': f"Action requires approval (cost impact: ${cost_impact:,.2f})",
'required_approvals': policy.conditions.get('approvers', ['manager'])
}
return {'allowed': True, 'reason': 'No approval required'}
async def _evaluate_compliance_rule(self, policy: Policy, action: Dict, context: Dict) -> Dict:
# Implement compliance rule evaluation (PCI, HIPAA, SOX, etc.)
compliance_tags = context.get('compliance_tags', [])
required_tags = policy.conditions.get('required_tags', [])
if not all(tag in compliance_tags for tag in required_tags):
return {
'allowed': False,
'reason': f"Missing required compliance tags: {set(required_tags) - set(compliance_tags)}"
}
return {'allowed': True, 'reason': 'Compliance requirements met'}
Advanced Model Integration Techniques
Hybrid Local-Cloud Model Architecture
Intelligent Model Selection:
class HybridModelOrchestrator:
def __init__(self):
self.local_models = {
'ollama_llama3': 'http://localhost:11434/api/generate',
'ollama_mistral': 'http://localhost:11434/api/generate',
'ollama_codellama': 'http://localhost:11434/api/generate'
}
self.cloud_models = {
'claude': 'https://api.anthropic.com/v1/messages',
'mistral_large': 'https://api.mistral.ai/v1/chat/completions'
}
self.model_characteristics = {
'ollama_llama3': {'latency': 'low', 'cost': 'free', 'privacy': 'high', 'capability': 'medium'},
'ollama_mistral': {'latency': 'low', 'cost': 'free', 'privacy': 'high', 'capability': 'medium'},
'claude': {'latency': 'medium', 'cost': 'medium', 'privacy': 'medium', 'capability': 'high'},
'mistral_large': {'latency': 'medium', 'cost': 'low', 'privacy': 'medium', 'capability': 'high'}
}
async def select_optimal_model(self, task_type: str, requirements: Dict) -> str:
"""Select the best model based on task requirements"""
# Define selection criteria
if requirements.get('privacy_critical', False):
# Use only local models for sensitive data
candidates = list(self.local_models.keys())
else:
candidates = list(self.local_models.keys()) + list(self.cloud_models.keys())
# Score models based on requirements
scored_models = []
for model in candidates:
score = self._calculate_model_score(model, task_type, requirements)
scored_models.append((model, score))
# Return best scoring model
return max(scored_models, key=lambda x: x[1])[0]
def _calculate_model_score(self, model: str, task_type: str, requirements: Dict) -> float:
characteristics = self.model_characteristics[model]
score = 0.0
# Latency scoring
if requirements.get('max_latency', 10) < 2:
score += 3 if characteristics['latency'] == 'low' else 0
# Cost scoring
if requirements.get('cost_sensitive', False):
score += 2 if characteristics['cost'] == 'free' else 0
# Privacy scoring
if requirements.get('privacy_critical', False):
score += 4 if characteristics['privacy'] == 'high' else 0
# Capability scoring for complex tasks
if task_type in ['complex_analysis', 'strategic_planning']:
score += 3 if characteristics['capability'] == 'high' else 1
return score
async def execute_with_fallback(self, prompt: str, task_type: str, requirements: Dict) -> Dict:
"""Execute request with automatic fallback to alternative models"""
primary_model = await self.select_optimal_model(task_type, requirements)
try:
return await self._query_model(primary_model, prompt)
except Exception as e:
# Fallback strategy
fallback_models = [m for m in self.model_characteristics.keys() if m != primary_model]
for fallback_model in fallback_models:
try:
result = await self._query_model(fallback_model, prompt)
# Log fallback usage
self._log_fallback(primary_model, fallback_model, str(e))
return result
except Exception:
continue
raise Exception("All models failed")
async def _query_model(self, model_name: str, prompt: str) -> Dict:
if model_name in self.local_models:
return await self._query_ollama(model_name, prompt)
else:
return await self._query_cloud_model(model_name, prompt)
async def _query_ollama(self, model_name: str, prompt: str) -> Dict:
model_key = model_name.split('_')[1] # Extract model name (llama3, mistral, etc.)
payload = {
"model": model_key,
"prompt": prompt,
"stream": False,
"options": {
"temperature": 0.1,
"top_p": 0.9,
"num_predict": 512
}
}
async with httpx.AsyncClient() as client:
response = await client.post(
self.local_models[model_name],
json=payload,
timeout=30.0
)
response.raise_for_status()
return response.json()
Advanced Prompt Engineering for Cost Management
Domain-Specific Prompt Templates:
class CostManagementPrompts:
def __init__(self):
self.templates = {
'anomaly_analysis': """
You are a cloud cost optimization expert. Analyze the following cost data and identify anomalies:
Cost Data:
{cost_data}
Context:
- Historical average: ${historical_avg}
- Current cost: ${current_cost}
- Time period: {time_period}
- Services involved: {services}
Please provide:
1. Anomaly detection (Yes/No) with confidence score
2. Root cause analysis
3. Recommended immediate actions
4. Potential cost impact if not addressed
Format your response as JSON with the following structure:
{{
"anomaly_detected": boolean,
"confidence_score": float,
"root_cause": "string",
"immediate_actions": ["action1", "action2"],
"cost_impact": float,
"reasoning": "detailed explanation"
}}
""",
'optimization_recommendation': """
You are an expert cloud architect focused on cost optimization. Given the following resource utilization data, provide optimization recommendations:
Resource Data:
{resource_data}
Current Configuration:
{current_config}
Performance Requirements:
{performance_requirements}
Budget Constraints:
{budget_constraints}
Provide specific, actionable recommendations that:
1. Reduce costs while maintaining or improving performance
2. Consider business requirements and constraints
3. Include estimated cost savings
4. Prioritize recommendations by impact and effort
Format as JSON:
{{
"recommendations": [
{{
"action": "string",
"estimated_savings": float,
"effort_level": "low|medium|high",
"risk_level": "low|medium|high",
"implementation_steps": ["step1", "step2"],
"expected_timeline": "string"
}}
],
"total_potential_savings": float,
"implementation_priority": ["rec1", "rec2", "rec3"]
}}
""",
'executive_summary': """
Create an executive summary for cloud cost management based on the following data:
Financial Summary:
- Total monthly cost: ${total_cost}
- Month-over-month change: {mom_change}%
- Year-over-year change: {yoy_change}%
- Budget utilization: {budget_utilization}%
Key Metrics:
{key_metrics}
Optimization Actions Taken:
{actions_taken}
Upcoming Initiatives:
{upcoming_initiatives}
Write a professional executive summary that:
1. Highlights key financial performance
2. Explains significant changes
3. Summarizes optimization impact
4. Outlines strategic recommendations
5. Uses business-friendly language (avoid technical jargon)
Keep it concise (200-300 words) and focus on business value.
"""
}
def get_prompt(self, template_name: str, **kwargs) -> str:
if template_name not in self.templates:
raise ValueError(f"Template {template_name} not found")
return self.templates[template_name].format(**kwargs)
def create_chain_of_thought_prompt(self, base_prompt: str, thinking_steps: List[str]) -> str:
"""Create a chain-of-thought prompt for complex reasoning"""
cot_prefix = """
Before providing your final answer, think through this step by step:
"""
for i, step in enumerate(thinking_steps, 1):
cot_prefix += f"{i}. {step}\n"
cot_prefix += "\nNow, work through each step and provide your final answer:\n\n"
return cot_prefix + base_prompt
Multi-Modal AI Integration
Integration with Vision Models for Infrastructure Diagrams:
class MultiModalCostAnalyzer:
def __init__(self):
self.vision_models = {
'diagram_analysis': 'llava:latest', # Ollama vision model
'chart_interpretation': 'bakllava:latest'
}
async def analyze_architecture_diagram(self, image_path: str, cost_context: Dict) -> Dict:
"""Analyze infrastructure diagrams to identify cost optimization opportunities"""
# Read image
with open(image_path, 'rb') as img_file:
image_data = base64.b64encode(img_file.read()).decode()
prompt = f"""
Analyze this cloud architecture diagram and identify potential cost optimization opportunities.
Consider the following cost context:
- Current monthly spend: ${cost_context['monthly_spend']}
- Top cost drivers: {', '.join(cost_context['top_drivers'])}
- Performance requirements: {cost_context['performance_requirements']}
Look for:
1. Over-provisioned resources
2. Unnecessary redundancy
3. Inefficient data flow patterns
4. Missing cost optimization services
5. Opportunities for serverless migration
Provide specific, actionable recommendations with estimated cost impact.
"""
# Query vision model through Ollama
response = await self._query_vision_model('llava:latest', prompt, image_data)
return {
'diagram_analysis': response,
'cost_optimization_score': self._calculate_optimization_score(response),
'recommended_actions': self._extract_actions(response)
}
async def interpret_cost_charts(self, chart_images: List[str]) -> Dict:
"""Interpret cost trend charts and graphs"""
interpretations = []
for chart_path in chart_images:
with open(chart_path, 'rb') as img_file:
image_data = base64.b64encode(img_file.read()).decode()
prompt = """
Analyze this cost chart/graph and provide insights:
1. Identify trends and patterns
2. Spot anomalies or unusual spikes
3. Determine seasonality effects
4. Suggest areas for investigation
5. Provide forecasting insights
Be specific about time periods, cost values, and percentage changes you observe.
"""
interpretation = await self._query_vision_model('bakllava:latest', prompt, image_data)
interpretations.append(interpretation)
return {
'chart_interpretations': interpretations,
'combined_insights': await self._synthesize_chart_insights(interpretations)
}
async def _query_vision_model(self, model: str, prompt: str, image_data: str) -> str:
payload = {
"model": model,
"prompt": prompt,
"images": [image_data],
"stream": False
}
async with httpx.AsyncClient() as client:
response = await client.post(
"http://localhost:11434/api/generate",
json=payload,
timeout=60.0
)
response.raise_for_status()
return response.json()['response']
Performance Optimization and Scaling
Distributed Agent Architecture
Agent Cluster Management:
import asyncio
import aioredis
from typing import List, Dict, Any
from dataclasses import dataclass, asdict
from datetime import datetime, timedelta
@dataclass
class AgentNode:
node_id: str
agent_type: str
status: str
last_heartbeat: datetime
current_load: float
max_capacity: int
client_assignments: List[str]
class DistributedAgentManager:
def __init__(self, redis_url: str):
self.redis_url = redis_url
self.redis = None
self.local_agents: Dict[str, Any] = {}
self.node_id = secrets.token_urlsafe(8)
async def initialize(self):
self.redis = await aioredis.from_url(self.redis_url)
await self.register_node()
# Start background tasks
asyncio.create_task(self.heartbeat_loop())
asyncio.create_task(self.load_balancer_loop())
async def register_node(self):
"""Register this node in the distributed cluster"""
node_info = AgentNode(
node_id=self.node_id,
agent_type="multi_purpose",
status="active",
last_heartbeat=datetime.now(),
current_load=0.0,
max_capacity=100,
client_assignments=[]
)
await self.redis.hset(
"agent_nodes",
self.node_id,
json.dumps(asdict(node_info), default=str)
)
async def heartbeat_loop(self):
"""Send periodic heartbeats to maintain cluster membership"""
while True:
try:
await self.redis.hset(
"agent_nodes",
self.node_id,
json.dumps({
"node_id": self.node_id,
"status": "active",
"last_heartbeat": datetime.now().isoformat(),
"current_load": self.calculate_current_load(),
"client_assignments": list(self.local_agents.keys())
})
)
await asyncio.sleep(30) # Heartbeat every 30 seconds
except Exception as e:
print(f"Heartbeat error: {e}")
await asyncio.sleep(5)
async def distribute_work(self, task: Dict) -> str:
"""Distribute work to the most appropriate agent node"""
# Get all active nodes
nodes = await self.get_active_nodes()
# Select best node based on load and capabilities
best_node = self.select_optimal_node(nodes, task)
if best_node == self.node_id:
# Execute locally
return await self.execute_local_task(task)
else:
# Send to remote node
return await self.send_remote_task(best_node, task)
def select_optimal_node(self, nodes: List[Dict], task: Dict) -> str:
"""Select the optimal node for task execution"""
# Simple load-based selection (can be enhanced with ML)
available_nodes = [
node for node in nodes
if node['current_load'] < 0.8 and node['status'] == 'active'
]
if not available_nodes:
return self.node_id # Fallback to local execution
# Select node with lowest load
best_node = min(available_nodes, key=lambda x: x['current_load'])
return best_node['node_id']
async def get_active_nodes(self) -> List[Dict]:
"""Get list of all active agent nodes"""
node_data = await self.redis.hgetall("agent_nodes")
nodes = []
for node_id, data in node_data.items():
node_info = json.loads(data)
# Check if node is still alive (heartbeat within last 2 minutes)
last_heartbeat = datetime.fromisoformat(node_info['last_heartbeat'])
if datetime.now() - last_heartbeat < timedelta(minutes=2):
nodes.append(node_info)
return nodes
class AdaptiveLoadBalancer:
def __init__(self):
self.load_history = deque(maxlen=100)
self.response_times = deque(maxlen=100)
self.error_rates = deque(maxlen=100)
def calculate_node_score(self, node: Dict, task_type: str) -> float:
"""Calculate a score for node selection based on multiple factors"""
# Base score from current load (lower is better)
load_score = 1.0 - node['current_load']
# Historical performance score
performance_score = self.get_historical_performance(node['node_id'])
# Task affinity score (some nodes might be better for certain tasks)
affinity_score = self.get_task_affinity(node['node_id'], task_type)
# Weighted combination
total_score = (load_score * 0.4 + performance_score * 0.4 + affinity_score * 0.2)
return total_score
def get_historical_performance(self, node_id: str) -> float:
# Implementation would track historical performance metrics
return 0.8 # Placeholder
def get_task_affinity(self, node_id: str, task_type: str) -> float:
# Some nodes might be optimized for specific task types
return 0.5 # Placeholder
Caching and Optimization Strategies
Intelligent Caching for AI Responses:
import hashlib
from typing import Optional, Tuple
import pickle
import asyncio
class IntelligentCache:
def __init__(self, redis_client, ttl_seconds: int = 3600):
self.redis = redis_client
self.ttl = ttl_seconds
self.hit_rate_window = deque(maxlen=1000)
async def get_or_compute(self,
key_data: Dict,
compute_func: Callable,
cache_strategy: str = 'standard') -> Tuple[Any, bool]:
"""Get cached result or compute new one with intelligent caching strategies"""
cache_key = self._generate_cache_key(key_data)
# Try to get from cache
cached_result = await self._get_cached_result(cache_key)
if cached_result is not None:
self.hit_rate_window.append(1) # Cache hit
return cached_result, True
# Cache miss - compute result
self.hit_rate_window.append(0) # Cache miss
result = await compute_func()
# Apply caching strategy
if cache_strategy == 'standard':
await self._cache_result(cache_key, result, self.ttl)
elif cache_strategy == 'adaptive':
ttl = await self._calculate_adaptive_ttl(key_data, result)
await self._cache_result(cache_key, result, ttl)
elif cache_strategy == 'predictive':
await self._predictive_cache(key_data, result)
return result, False
def _generate_cache_key(self, key_data: Dict) -> str:
"""Generate deterministic cache key from input data"""
# Sort keys to ensure consistent ordering
sorted_data = json.dumps(key_data, sort_keys=True)
# Create hash
return hashlib.sha256(sorted_data.encode()).hexdigest()
async def _get_cached_result(self, cache_key: str) -> Optional[Any]:
"""Retrieve result from cache"""
try:
cached_data = await self.redis.get(f"cache:{cache_key}")
if cached_data:
return pickle.loads(cached_data)
except Exception as e:
print(f"Cache retrieval error: {e}")
return None
async def _cache_result(self, cache_key: str, result: Any, ttl: int):
"""Store result in cache"""
try:
serialized_result = pickle.dumps(result)
await self.redis.setex(f"cache:{cache_key}", ttl, serialized_result)
except Exception as e:
print(f"Cache storage error: {e}")
async def _calculate_adaptive_ttl(self, key_data: Dict, result: Any) -> int:
"""Calculate adaptive TTL based on data characteristics"""
base_ttl = self.ttl
# Adjust TTL based on result confidence
if isinstance(result, dict) and 'confidence' in result:
confidence = result['confidence']
# Higher confidence = longer TTL
ttl_multiplier = 0.5 + (confidence * 1.5)
base_ttl = int(base_ttl * ttl_multiplier)
# Adjust based on data volatility
if 'real_time' in key_data and key_data['real_time']:
base_ttl = min(base_ttl, 300) # Max 5 minutes for real-time data
# Adjust based on cost of computation
computation_cost = key_data.get('computation_cost', 'medium')
if computation_cost == 'high':
base_ttl *= 2 # Cache longer for expensive computations
elif computation_cost == 'low':
base_ttl = int(base_ttl * 0.5) # Shorter cache for cheap computations
return max(60, min(base_ttl, 86400)) # Between 1 minute and 1 day
async def _predictive_cache(self, key_data: Dict, result: Any):
"""Implement predictive caching for likely future requests"""
# Analyze patterns to predict future cache needs
similar_keys = await self._find_similar_cache_patterns(key_data)
for similar_key in similar_keys:
# Pre-warm cache for similar requests
asyncio.create_task(self._precompute_similar_request(similar_key))
def get_cache_stats(self) -> Dict:
"""Get cache performance statistics"""
if not self.hit_rate_window:
return {'hit_rate': 0.0, 'total_requests': 0}
hit_rate = sum(self.hit_rate_window) / len(self.hit_rate_window)
return {
'hit_rate': hit_rate,
'total_requests': len(self.hit_rate_window),
'cache_hits': sum(self.hit_rate_window),
'cache_misses': len(self.hit_rate_window) - sum(self.hit_rate_window)
}
Conclusion and Future Directions
Building agentic AI systems for cloud cost management represents a significant evolution in how organizations approach cost optimization. By leveraging open-source models through platforms like Ollama, combined with cloud-based AI services from Anthropic and Mistral, organizations can create sophisticated, autonomous systems that continuously optimize cloud spending while maintaining performance and compliance requirements.
The key to success lies in creating a robust, scalable architecture that can adapt to changing requirements and learn from experience. The multi-agent approach allows for specialization and coordination, while the use of both local and cloud-based models provides flexibility in balancing cost, privacy, and capability requirements.
Future Enhancements
Advanced AI Capabilities:
- Integration of multimodal AI for processing infrastructure diagrams, dashboards, and documentation
- Implementation of federated learning for cross-client insights while maintaining privacy
- Development of domain-specific fine-tuned models for cloud cost optimization
Enhanced Automation:
- Autonomous contract negotiation with cloud providers
- Predictive scaling based on business events and seasonal patterns
- Integration with business intelligence systems for holistic cost optimization
Improved Decision Making:
- Causal inference models to understand the true impact of optimization actions
- Game-theoretic approaches for multi-cloud optimization
- Integration of sustainability metrics alongside cost optimization
The future of cloud cost management lies in intelligent, autonomous systems that can understand business context, predict future needs, and take proactive actions to optimize costs while ensuring performance and compliance. By implementing the architecture and techniques outlined in this guide, organizations can build powerful agentic AI systems that transform cloud cost management from a reactive discipline to a proactive, strategic advantage.
As the field continues to evolve, staying current with advances in AI models, cloud technologies, and optimization techniques will be crucial for maintaining competitive advantage in the rapidly changing landscape of cloud computing and artificial intelligence.
Sentiment Analysis with RNN End to End Project: A Technical Exploration
In today’s digital landscape, understanding sentiment from text data has become a crucial component for businesses and researchers alike. This blog post explores an end-to-end implementation of a sentiment analysis system using Recurrent Neural Networks (RNNs), with a detailed examination of the underlying code, architecture decisions, and deployment strategy.
Try the Sentiment WebApp: model Accuracy > 90%
IMDB Sentiment Analysis Webapp
Analyze the sentiment of any IMDB review using our Sentiment Analysis Tool
Launch ApplicationIntroduction to the Project
The Sentiment Analysis RNN project by Tejas K provides a comprehensive implementation of sentiment analysis that takes raw text as input and classifies it into positive, negative, or neutral categories. What makes this project stand out is its careful attention to the entire machine learning pipeline from data preprocessing to deployment.
Let’s delve into the technical aspects of this implementation.
Data Preprocessing: The Foundation
The quality of any NLP model heavily depends on how well the text data is preprocessed. The project implements several crucial preprocessing steps:
def preprocess_text(text):
# Convert to lowercase
text = text.lower()
# Remove HTML tags
text = re.sub(r'<.*?>', '', text)
# Remove special characters and numbers
text = re.sub(r'[^a-zA-Z\s]', '', text)
# Tokenize
tokens = word_tokenize(text)
# Remove stopwords
stop_words = set(stopwords.words('english'))
tokens = [word for word in tokens if word not in stop_words]
# Lemmatization
lemmatizer = WordNetLemmatizer()
tokens = [lemmatizer.lemmatize(word) for word in tokens]
return ' '.join(tokens)
This preprocessing function performs several important operations:
- Converting text to lowercase to ensure consistent processing
- Removing HTML tags that might be present in web-scraped data
- Filtering out special characters and numbers to focus on alphabetic content
- Tokenizing the text into individual words
- Removing stopwords (common words like “the”, “and”, etc.) that typically don’t carry sentiment
- Lemmatizing words to reduce them to their base form
Building the Vocabulary: Tokenization and Embedding
Before feeding text to an RNN, we need to convert words into numerical vectors. The project implements a vocabulary builder and embedding mechanism:
class Vocabulary:
def __init__(self, max_size=None):
self.word2idx = {"<PAD>": 0, "<UNK>": 1}
self.idx2word = {0: "<PAD>", 1: "<UNK>"}
self.word_count = {}
self.max_size = max_size
def add_word(self, word):
if word not in self.word_count:
self.word_count[word] = 1
else:
self.word_count[word] += 1
def build_vocab(self):
# Sort words by frequency
sorted_words = sorted(self.word_count.items(), key=lambda x: x[1], reverse=True)
# Take only max_size most common words if specified
if self.max_size:
sorted_words = sorted_words[:self.max_size-2] # -2 for <PAD> and <UNK>
# Add words to dictionaries
for word, _ in sorted_words:
idx = len(self.word2idx)
self.word2idx[word] = idx
self.idx2word[idx] = word
def text_to_indices(self, text, max_length=None):
words = text.split()
indices = [self.word2idx.get(word, self.word2idx["<UNK>"]) for word in words]
if max_length:
if len(indices) > max_length:
indices = indices[:max_length]
else:
indices += [self.word2idx["<PAD>"]] * (max_length - len(indices))
return indices
This vocabulary class:
- Maintains mappings between words and their numerical indices
- Counts word frequencies to build a vocabulary of the most common words
- Handles unknown words with a special
<UNK>
token - Pads sequences to a consistent length with a
<PAD>
token - Converts text to sequences of indices for model processing
The Core: RNN Model Architecture
The heart of the project is the RNN model architecture. The implementation uses PyTorch to build a flexible model that can be configured with different RNN cell types (LSTM or GRU) and embedding dimensions:
class SentimentRNN(nn.Module):
def __init__(self, vocab_size, embedding_dim, hidden_dim, output_dim, n_layers,
bidirectional, dropout, pad_idx, cell_type='lstm'):
super().__init__()
self.embedding = nn.Embedding(vocab_size, embedding_dim, padding_idx=pad_idx)
if cell_type.lower() == 'lstm':
self.rnn = nn.LSTM(embedding_dim,
hidden_dim,
num_layers=n_layers,
bidirectional=bidirectional,
dropout=dropout if n_layers > 1 else 0,
batch_first=True)
elif cell_type.lower() == 'gru':
self.rnn = nn.GRU(embedding_dim,
hidden_dim,
num_layers=n_layers,
bidirectional=bidirectional,
dropout=dropout if n_layers > 1 else 0,
batch_first=True)
else:
raise ValueError("cell_type must be 'lstm' or 'gru'")
self.fc = nn.Linear(hidden_dim * 2 if bidirectional else hidden_dim, output_dim)
self.dropout = nn.Dropout(dropout)
def forward(self, text, text_lengths):
# text = [batch size, seq length]
embedded = self.dropout(self.embedding(text))
# embedded = [batch size, seq length, embedding dim]
# Pack sequence for RNN efficiency
packed_embedded = nn.utils.rnn.pack_padded_sequence(embedded, text_lengths.cpu(),
batch_first=True, enforce_sorted=False)
if isinstance(self.rnn, nn.LSTM):
packed_output, (hidden, _) = self.rnn(packed_embedded)
else: # GRU
packed_output, hidden = self.rnn(packed_embedded)
# hidden = [n layers * n directions, batch size, hidden dim]
# If bidirectional, concatenate the final forward and backward hidden states
if self.rnn.bidirectional:
hidden = self.dropout(torch.cat((hidden[-2,:,:], hidden[-1,:,:]), dim=1))
else:
hidden = self.dropout(hidden[-1,:,:])
# hidden = [batch size, hidden dim * n directions]
return self.fc(hidden)
This model includes several key components:
- An embedding layer that converts word indices to dense vectors
- A configurable RNN layer (either LSTM or GRU) that processes the sequence
- Support for bidirectional processing to capture context from both directions
- Dropout for regularization to prevent overfitting
- A final fully connected layer for classification
- Efficient sequence packing to handle variable-length inputs
Training the Model: The Learning Process
The training loop implements several best practices for deep learning:
def train_model(model, train_iterator, optimizer, criterion):
model.train()
epoch_loss = 0
epoch_acc = 0
for batch in train_iterator:
optimizer.zero_grad()
text, text_lengths = batch.text
predictions = model(text, text_lengths)
loss = criterion(predictions, batch.label)
acc = calculate_accuracy(predictions, batch.label)
loss.backward()
nn.utils.clip_grad_norm_(model.parameters(), max_norm=5)
optimizer.step()
epoch_loss += loss.item()
epoch_acc += acc.item()
return epoch_loss / len(train_iterator), epoch_acc / len(train_iterator)
Notable aspects include:
- Setting the model to training mode with
model.train()
- Zeroing gradients before each batch to prevent accumulation
- Computing loss and accuracy for monitoring training progress
- Implementing gradient clipping to prevent exploding gradients
- Updating model weights with the optimizer
- Tracking and returning average loss and accuracy
Evaluation and Testing: Measuring Performance
The evaluation function follows a similar structure but disables certain training-specific components:
def evaluate_model(model, iterator, criterion):
model.eval()
epoch_loss = 0
epoch_acc = 0
with torch.no_grad():
for batch in iterator:
text, text_lengths = batch.text
predictions = model(text, text_lengths)
loss = criterion(predictions, batch.label)
acc = calculate_accuracy(predictions, batch.label)
epoch_loss += loss.item()
epoch_acc += acc.item()
return epoch_loss / len(iterator), epoch_acc / len(iterator)
Key differences from the training function:
- Setting the model to evaluation mode with
model.eval()
- Using
torch.no_grad()
to disable gradient calculation for efficiency - Not performing backward passes or optimizer steps
Model Deployment: From PyTorch to Streamlit
The project’s deployment strategy involves exporting the trained PyTorch model to TorchScript for production use:
def export_model(model, vocab):
model.eval()
# Create a script module from the PyTorch model
example_text = torch.randint(0, len(vocab), (1, 10))
example_lengths = torch.tensor([10])
traced_model = torch.jit.trace(model, (example_text, example_lengths))
# Save the scripted model
torch.jit.save(traced_model, "sentiment_model.pt")
# Save the vocabulary
with open("vocab.json", "w") as f:
json.dump({
"word2idx": vocab.word2idx,
"idx2word": {int(k): v for k, v in vocab.idx2word.items()}
}, f)
The exported model is then integrated into a Streamlit application for easy access:
def load_model():
# Load the TorchScript model
model = torch.jit.load("sentiment_model.pt")
# Load vocabulary
with open("vocab.json", "r") as f:
vocab_data = json.load(f)
# Recreate vocabulary object
vocab = Vocabulary()
vocab.word2idx = vocab_data["word2idx"]
vocab.idx2word = {int(k): v for k, v in vocab_data["idx2word"].items()}
return model, vocab
def predict_sentiment(model, vocab, text):
# Preprocess text
processed_text = preprocess_text(text)
# Convert to indices
indices = vocab.text_to_indices(processed_text, max_length=100)
tensor = torch.LongTensor(indices).unsqueeze(0) # Add batch dimension
length = torch.tensor([len(indices)])
# Make prediction
model.eval()
with torch.no_grad():
prediction = model(tensor, length)
# Get probability using softmax
probabilities = F.softmax(prediction, dim=1)
# Get predicted class
predicted_class = torch.argmax(prediction, dim=1).item()
# Map to sentiment
sentiment_map = {0: "Negative", 1: "Neutral", 2: "Positive"}
return {
"sentiment": sentiment_map[predicted_class],
"confidence": probabilities[0][predicted_class].item(),
"probabilities": {
sentiment_map[i]: prob.item() for i, prob in enumerate(probabilities[0])
}
}
The Streamlit application code brings everything together in a user-friendly interface:
def main():
st.title("Sentiment Analysis with RNN")
model, vocab = load_model()
st.write("Enter text to analyze its sentiment:")
user_input = st.text_area("Text input", "")
if st.button("Analyze Sentiment"):
if user_input:
with st.spinner("Analyzing..."):
result = predict_sentiment(model, vocab, user_input)
st.write(f"**Sentiment:** {result['sentiment']}")
st.write(f"**Confidence:** {result['confidence']*100:.2f}%")
# Display probabilities
st.write("### Probability Distribution")
for sentiment, prob in result['probabilities'].items():
st.write(f"{sentiment}: {prob*100:.2f}%")
st.progress(prob)
else:
st.warning("Please enter some text to analyze.")
if __name__ == "__main__":
main()
The iframe parameters and styling ensure:
- The dark theme specified with
embed_options=dark_theme
- Responsive design that works on different screen sizes
- Clean integration with the WordPress site’s aesthetics
- Proper sizing to accommodate the application’s interface
Performance Optimization and Model Improvements
The project implements several performance optimizations:
- Batch processing during training to improve GPU utilization:
def create_iterators(train_data, valid_data, test_data, batch_size=64):
train_iterator, valid_iterator, test_iterator = data.BucketIterator.splits(
(train_data, valid_data, test_data),
batch_size=batch_size,
sort_key=lambda x: len(x.text),
sort_within_batch=True,
device=device)
return train_iterator, valid_iterator, test_iterator
- Early stopping to prevent overfitting:
def train_with_early_stopping(model, train_iterator, valid_iterator,
optimizer, criterion, patience=5):
best_valid_loss = float('inf')
epochs_without_improvement = 0
for epoch in range(max_epochs):
train_loss, train_acc = train_model(model, train_iterator, optimizer, criterion)
valid_loss, valid_acc = evaluate_model(model, valid_iterator, criterion)
if valid_loss < best_valid_loss:
best_valid_loss = valid_loss
torch.save(model.state_dict(), 'best-model.pt')
epochs_without_improvement = 0
else:
epochs_without_improvement += 1
print(f'Epoch: {epoch+1}')
print(f'\tTrain Loss: {train_loss:.3f} | Train Acc: {train_acc*100:.2f}%')
print(f'\tVal. Loss: {valid_loss:.3f} | Val. Acc: {valid_acc*100:.2f}%')
if epochs_without_improvement >= patience:
print(f'Early stopping after {epoch+1} epochs')
break
# Load the best model
model.load_state_dict(torch.load('best-model.pt'))
return model
- Learning rate scheduling for better convergence:
optimizer = optim.Adam(model.parameters(), lr=2e-4)
scheduler = optim.lr_scheduler.ReduceLROnPlateau(optimizer, mode='min',
factor=0.5, patience=2)
# In training loop
scheduler.step(valid_loss)
Conclusion: Putting It All Together
The Sentiment Analysis RNN project demonstrates how to build a complete NLP system from data preprocessing to web deployment. Key technical takeaways include:
- Effective text preprocessing is crucial for good model performance
- RNNs (particularly LSTMs and GRUs) excel at capturing sequential dependencies in text
- Proper training techniques like early stopping and learning rate scheduling improve model quality
- Model export and deployment bridges the gap between development and production
- Web integration makes the model accessible to end-users without technical knowledge
By embedding the Streamlit application in a WordPress site, this technical solution becomes accessible to a wider audience, showcasing how advanced NLP techniques can be applied to practical problems.
The combination of robust model architecture, efficient training procedures, and user-friendly deployment makes this project an excellent case study in applied deep learning for natural language processing.
You can explore the full implementation on GitHub or try the live demo at Streamlit App.
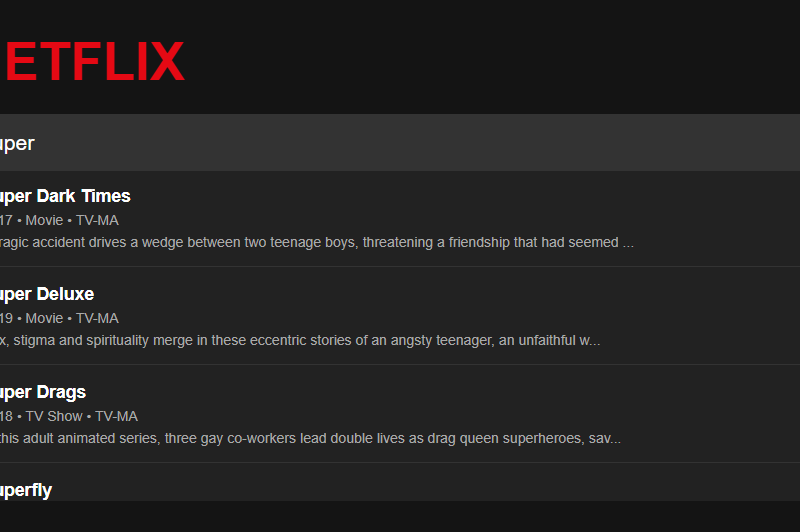
Netflix Autosuggest Search Engine
By Tejas Kamble – AI/ML Developer & Researcher | tejaskamble.com
Introduction
Have you ever used the Netflix search bar and instantly seen suggestions that seem to know exactly what you’re looking for—even before you finish typing? Inspired by this, I created a Netflix Search Engine using NLP Text Suggestions — a project that bridges the power of natural language processing (NLP) with real-time search functionalities.
In this post, I’ll walk you through the codebase hosted on my GitHub: Netflix_Search_Engine_NLP_Text_suggestion, breaking down each important part, from data loading and text preprocessing to building the suggestion logic and deploying it using Flask.
📂 Project Structure
Netflix_Search_Engine_NLP_Text_suggestion/
├── app.py ← Flask Web App
├── netflix_titles.csv ← Dataset of Netflix shows/movies
├── templates/
│ ├── index.html ← Frontend UI
├── static/
│ └── style.css ← Custom styling
├── requirements.txt ← Python dependencies
└── README.md ← Project overview
Dataset Overview
I used a dataset of Netflix titles (from Kaggle). It includes:
- Title: Name of the show/movie
- Description: Synopsis of the content
- Cast: Actors involved
- Genres, Date Added, Duration and more…
This dataset is essential for understanding user intent when making text suggestions.
Step-by-Step Breakdown of the Code
Loading the Dataset
df = pd.read_csv("netflix_titles.csv")
df.dropna(subset=['title'], inplace=True)
We load the dataset and ensure there are no missing values in the title
column since that’s our search anchor.
Text Vectorization using TF-IDF
from sklearn.feature_extraction.text import TfidfVectorizer
vectorizer = TfidfVectorizer(stop_words='english')
tfidf_matrix = vectorizer.fit_transform(df['title'])
- TF-IDF (Term Frequency-Inverse Document Frequency) is used to convert titles into numerical vectors.
- This helps quantify the importance of each word in the context of the entire dataset.
Cosine Similarity Search
from sklearn.metrics.pairwise import cosine_similarity
def get_recommendations(input_text):
input_vec = vectorizer.transform([input_text])
similarity = cosine_similarity(input_vec, tfidf_matrix)
indices = similarity.argsort()[0][-5:][::-1]
return df['title'].iloc[indices]
Here’s where the magic happens:
- The user input is vectorized.
- We compute cosine similarity with all titles.
- The top 5 most similar titles are returned as recommendations.
Flask Web Application
The search engine is hosted using a lightweight Flask backend.
@app.route("/", methods=["GET", "POST"])
def index():
if request.method == "POST":
user_input = request.form["title"]
suggestions = get_recommendations(user_input)
return render_template("index.html", suggestions=suggestions, query=user_input)
return render_template("index.html")
- Accepts user input from the HTML form
- Processes it through
get_recommendations()
- Displays top matching titles
Frontend – index.html
A simple yet effective UI allows users to interact with the engine.
<form method="POST">
<input type="text" name="title" placeholder="Search for Netflix titles...">
<button type="submit">Search</button>
</form>
If suggestions are found, they’re shown dynamically below the form.
🌐 Deployment
To run this app locally:
git clone https://github.com/tejask0512/Netflix_Search_Engine_NLP_Text_suggestion
cd Netflix_Search_Engine_NLP_Text_suggestion
pip install -r requirements.txt
python app.py
Then open http://127.0.0.1:5000
in your browser!
Key Takeaways
- TF-IDF is powerful for information retrieval tasks.
- Even a simple cosine similarity search can replicate sophisticated autocomplete behavior.
- Flask makes it easy to bring machine learning to the web.
What’s Next?
Here are a few ways I plan to extend this project:
- Use BERT or Sentence Transformers for semantic similarity.
- Add spell correction and synonym support.
- Deploy it on Render, Heroku, or HuggingFace Spaces.
- Add a recommendation engine using genres, cast similarity, or collaborative filtering.
🧑💻 About Me
I’m Tejas Kamble, an AI/ML Developer & Researcher passionate about building intelligent, ethical, and multilingual human-computer interaction systems. I focus on:
- AI-driven trading strategies
- NLP-based behavioral analysis
- Real-time blockchain sentiment analysis
- Deep learning for crop disease detection
Check out more of my work on my GitHub @tejask0512
🌐 Website: tejaskamble.com
💬 Feedback & Collaboration
I’d love to hear your thoughts or collaborate on cool projects!
Let’s connect: tejaskamble.com/contact
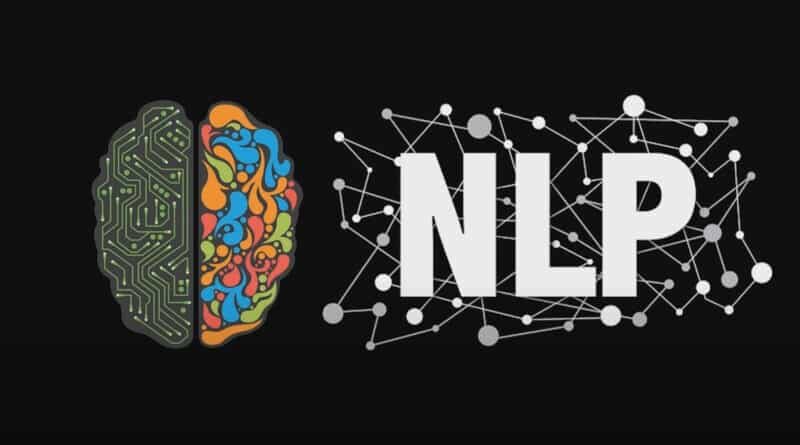
Leveraging Word2Vec: Practical Applications of Google’s 3 Billion Word Pre-trained Model
In the ever-evolving field of Natural Language Processing (NLP), word embeddings have revolutionized how machines understand human language. Among these technologies, Word2Vec stands as a foundational approach that transforms words into meaningful vector representations. This blog explores the practical implementation of Word2Vec using Google’s massive pre-trained model trained on approximately 3 billion words and phrases, demonstrating its versatility through diverse use cases.
Understanding Word2Vec: Beyond Simple Word Representation
Word2Vec, developed by researchers at Google, transforms words into numerical vectors where semantic relationships between words are preserved in vector space. Unlike traditional one-hot encoding methods, Word2Vec captures the contextual meaning of words, allowing machines to understand language nuances previously beyond their grasp.
Key Advantages of Word2Vec
- Semantic Relationships: Word2Vec captures semantic similarities between words, placing related concepts closer in vector space.
- Dimensionality Efficiency: While maintaining rich semantic information, Word2Vec typically uses only 300 dimensions per word (compared to vocabulary-sized vectors in one-hot encoding).
- Arithmetic Operations on Words: Perhaps most fascinating is Word2Vec’s ability to perform meaningful arithmetic with words. The classic example
king - man + woman ≈ queen
demonstrates how these vectors encode gender, royalty, and other semantic concepts. - Transfer Learning Capability: Pre-trained embeddings allow models to benefit from knowledge learned on massive text corpora without requiring extensive training data.
- Language Agnosticism: The core techniques work across languages, making it valuable for multilingual applications.
- Handling Out-of-Vocabulary Words: With techniques like subword embeddings, Word2Vec approaches can handle previously unseen words.
Exploring the Practical Implementation
Looking at the implementation repository, we can see how Google’s pre-trained model is leveraged through the gensim
library. Let’s explore some of the practical applications and extend them further.
Word Similarity and Relationships
The repository demonstrates finding similar words—a fundamental application of Word2Vec. For example, finding words most similar to “intelligent” reveals words like “smart,” “brilliant,” and “clever.” This capability forms the foundation for many downstream applications, from recommendation systems to semantic search.
model.most_similar("intelligent")
Analogical Reasoning
Word2Vec’s ability to perform word arithmetic allows for solving analogies:
model.most_similar(positive=['woman', 'king'], negative=['man'])
This returns “queen” as the top result, demonstrating the model’s understanding of gender relationships combined with royal status.
Advanced Use Cases for Google’s Pre-trained Model
Let’s explore additional applications beyond those covered in the repository, leveraging the power of Google’s 3-billion-word pre-trained embeddings:
1. Document Classification and Clustering
By averaging Word2Vec vectors for all words in a document, we can create document vectors for classification or clustering:
def document_vector(doc):
words = doc.lower().split()
word_vectors = [model[word] for word in words if word in model]
return np.mean(word_vectors, axis=0) if word_vectors else np.zeros(model.vector_size)
# Example documents
documents = [
"Artificial intelligence is transforming healthcare systems globally",
"Machine learning algorithms help diagnose diseases early",
"The stock market fluctuated significantly last quarter",
"Investors are concerned about economic indicators"
]
# Create document vectors
doc_vectors = [document_vector(doc) for doc in documents]
# Cluster documents
from sklearn.cluster import KMeans
kmeans = KMeans(n_clusters=2)
clusters = kmeans.fit_predict(doc_vectors)
This approach can group documents by topic without explicit topic modeling.
2. Sentiment Analysis Enhancement
Word2Vec can improve sentiment analysis by accounting for semantic relationships:
def sentiment_score(text, positive_words, negative_words):
words = text.lower().split()
score = 0
for word in words:
if word in model:
# Calculate similarity to positive and negative word sets
pos_similarity = np.mean([model.similarity(word, pos) for pos in positive_words if pos in model])
neg_similarity = np.mean([model.similarity(word, neg) for neg in negative_words if neg in model])
score += (pos_similarity - neg_similarity)
return score / max(len(words), 1) # Normalize by text length
# Example usage
positive_words = ["excellent", "amazing", "wonderful", "great"]
negative_words = ["terrible", "awful", "horrible", "bad"]
texts = [
"The product exceeded my expectations and works flawlessly.",
"This was a complete waste of money and time."
]
for text in texts:
print(f"Text: {text}")
print(f"Sentiment score: {sentiment_score(text, positive_words, negative_words):.4f}")
This method can detect sentiment in texts containing words not explicitly in our sentiment lexicons.
3. Named Entity Recognition Support
Word2Vec embeddings can enhance named entity recognition by providing semantic context:
def is_likely_organization(word, context_words):
org_indicators = ["company", "corporation", "organization", "enterprise"]
if word not in model:
return False
# Check similarity to organization indicators
org_similarity = np.mean([model.similarity(word, org) for org in org_indicators if org in model])
# Check if context suggests an organization
context_similarity = 0
if context_words:
context_vectors = [model[w] for w in context_words if w in model]
if context_vectors:
context_vector = np.mean(context_vectors, axis=0)
for org in org_indicators:
if org in model:
context_similarity += cosine_similarity([model[org]], [context_vector])[0][0]
context_similarity /= len(org_indicators)
return (org_similarity > 0.3) or (context_similarity > 0.4)
4. Concept Expansion and Exploration
Word2Vec can help expand topic-related terms for content creation or research:
def explore_concept(seed_terms, depth=2, breadth=5):
"""Explore related concepts starting from seed terms."""
all_terms = set(seed_terms)
current_terms = seed_terms
for d in range(depth):
next_level = []
for term in current_terms:
if term in model:
similar_terms = [word for word, _ in model.most_similar(term, topn=breadth)]
next_level.extend(similar_terms)
next_level = list(set(next_level) - all_terms) # Remove duplicates
all_terms.update(next_level)
current_terms = next_level
return all_terms
# Example: Explore AI-related concepts
ai_concepts = explore_concept(["artificial_intelligence", "machine_learning"], depth=2, breadth=7)
This function can help researchers explore interconnected concepts or content creators develop comprehensive topic coverage.
5. Translation Assistance
While not a complete translation system, Word2Vec can help with cross-language word mapping:
def find_translation_candidates(word, source_model, target_model, bridge_words):
"""Find possible translations using bridge words known in both languages."""
if word not in source_model:
return []
candidates = {}
for bridge in bridge_words:
if bridge in source_model and bridge in target_model:
# Find words similar to our word in source language
source_similar = [w for w, _ in source_model.most_similar(word, topn=10)]
# For each similar word, find corresponding words in target language
for s_word in source_similar:
if s_word in source_model:
# Use the bridge word to find target language equivalents
target_similar = [w for w, _ in target_model.most_similar(bridge, topn=20)]
for t_word in target_similar:
candidates[t_word] = candidates.get(t_word, 0) + 1
# Return candidates sorted by frequency
return sorted(candidates.items(), key=lambda x: x[1], reverse=True)
Research Implications and Future Directions
The Google pre-trained Word2Vec model’s 3 billion word training corpus offers several research advantages:
- Robust Representation: The massive training corpus ensures stable, noise-resistant word representations capturing subtle semantic relationships.
- Knowledge Transfer: Pre-trained embeddings transfer knowledge from vast text collections to specialized domains with limited training data.
- Cross-domain Applications: Word2Vec’s language agnosticism allows transferring knowledge across domains—using knowledge from general corpora for specialized applications like medical text analysis.
- Foundation for Advanced Architectures: While newer models like BERT and GPT have emerged, Word2Vec remains relevant as a lightweight alternative and serves as the conceptual foundation for these more complex architectures.
- Interpretability: Unlike black-box transformers, Word2Vec representations are more interpretable through techniques like principal component analysis of word vectors.
Challenges and Limitations
Despite its advantages, researchers should be aware of Word2Vec’s limitations:
- Context Insensitivity: Each word has exactly one vector, regardless of context (unlike BERT’s contextual embeddings).
- Training Corpus Bias: Embeddings inherit biases present in the training corpus, potentially perpetuating stereotypes.
- Rare Word Problem: Words appearing infrequently in the training corpus have less reliable representations.
- Computational Requirements: While more efficient than newer transformer models, loading Google’s pre-trained vectors still requires significant memory.
Conclusion
Google’s pre-trained Word2Vec model trained on 3 billion words offers a powerful foundation for numerous NLP applications. From semantic search to document classification, sentiment analysis to concept exploration, these word embeddings continue to provide value despite newer architectures.
The practical implementations explored in this blog demonstrate how a single pre-trained model can address diverse language understanding challenges without extensive additional training. As NLP research advances, Word2Vec remains relevant as both a standalone solution for many applications and a conceptual building block for understanding more complex embedding approaches.
For researchers and practitioners working with limited computational resources or seeking interpretable word representations, Google’s pre-trained Word2Vec model remains an invaluable tool in the NLP toolkit.
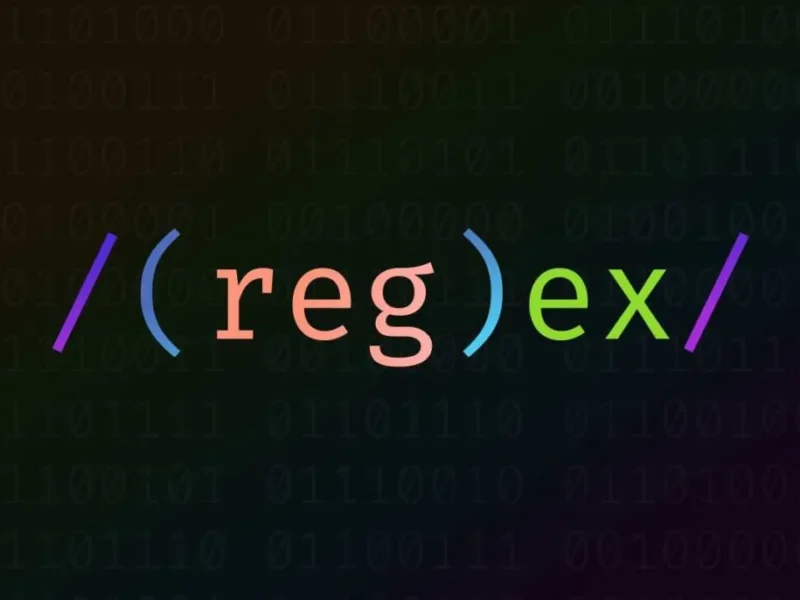
RegEx Mastery: Unlocking Structured Data From Unstructured Text
A comprehensive guide to advanced regular expressions for data mining and extraction
Introduction
In today’s data-driven world, the ability to efficiently extract structured information from unstructured text is invaluable. While many sophisticated NLP and machine learning tools exist for this purpose, regular expressions (regex) remain one of the most powerful and flexible tools in a data scientist’s toolkit. This blog explores advanced regex techniques implemented in the “Advance-Regex-For-Data-Mining-Extraction” project by Tejas K., demonstrating how carefully crafted patterns can transform raw text into actionable insights.
What Makes Regex Essential for Text Mining?
Regular expressions provide a concise, pattern-based approach to text processing that is:
- Language-agnostic: Works across programming languages and text processing tools
- Highly efficient: Once optimized, regex patterns can process large volumes of text quickly
- Precisely targeted: Allows extraction of exactly the information you need
- Flexible: Can be adapted to handle variations in text structure and format
Core Advanced Regex Techniques
Lookahead and Lookbehind Assertions
Lookahead (?=
) and lookbehind (?<=
) assertions are powerful techniques that allow matching patterns based on context without including that context in the match itself.
(?<=Price: \$)\d+\.\d{2}
This pattern matches a price value but only if it’s preceded by “Price: $”, without including “Price: $” in the match.
Non-Capturing Groups
When you need to group parts of a pattern but don’t need to extract that specific group:
(?:https?|ftp):\/\/[\w\.-]+\.[\w\.-]+
The ?:
tells the regex engine not to store the protocol match (http, https, or ftp), improving performance.
Named Capture Groups
Named capture groups make your regex more readable and the extracted data more easily accessible:
(?<date>\d{2}-\d{2}-\d{4}).*?(?<email>[a-zA-Z0-9._%+-]+@[a-zA-Z0-9.-]+\.[a-zA-Z]{2,})
Instead of working with numbered groups, you can now reference the extractions by name: date
and email
.
Balancing Groups for Nested Structures
The project implements sophisticated balancing groups for parsing nested structures like JSON or HTML:
\{(?<open>\{)|(?<-open>\})|[^{}]*\}(?(open)(?!))
This pattern matches properly nested curly braces, essential for parsing structured data formats.
Real-World Applications in the Project
1. Extracting Structured Information from Resumes
The project demonstrates how to parse unstructured resume text to extract:
Education: (?<education>(?:(?!Experience|Skills).)+)
Experience: (?<experience>(?:(?!Education|Skills).)+)
Skills: (?<skills>.+)
This pattern breaks a resume into logical sections, making it possible to analyze each component separately.
2. Mining Financial Data from Reports
Annual reports and financial statements contain valuable data that can be extracted with patterns like:
Revenue of \$(?<revenue>[\d,]+(?:\.\d+)?) million in (?<year>\d{4})
This extracts both the revenue figure and the corresponding year in a single operation.
3. Processing Log Files
The project includes patterns for parsing common log formats:
(?<ip>\d{1,3}\.\d{1,3}\.\d{1,3}\.\d{1,3}) - - \[(?<datetime>[^\]]+)\] "(?<request>[^"]*)" (?<status>\d+) (?<size>\d+)
This extracts IP addresses, timestamps, request details, status codes, and response sizes from standard HTTP logs.
Performance Optimization Techniques
1. Catastrophic Backtracking Prevention
The project implements strategies to avoid catastrophic backtracking, which can cause regex operations to hang:
# Instead of this (vulnerable to backtracking)
(\w+\s+){1,5}
# Use this (prevents backtracking issues)
(?:\w+\s+){1,5}?
2. Atomic Grouping
Atomic groups improve performance by preventing unnecessary backtracking:
(?>https?://[\w-]+(\.[\w-]+)+)
Once the atomic group matches, the regex engine doesn’t try alternative ways to match it.
3. Strategic Anchoring
Using anchors strategically improves performance by limiting where the regex engine needs to look:
^Subject: (.+)$
By anchoring to line start/end, the engine only attempts matches at line boundaries.
Implementation in Python
The project primarily uses Python’s re
module for implementation:
import re
def extract_structured_data(text):
pattern = r'Name: (?P<name>[\w\s]+)\s+Email: (?P<email>[^\s]+)\s+Phone: (?P<phone>[\d\-\(\)\s]+)'
match = re.search(pattern, text, re.MULTILINE)
if match:
return match.groupdict()
return None
For more complex operations, the project leverages the more powerful regex
module which supports advanced features like recursive patterns:
import regex
def extract_nested_structures(text):
pattern = r'\((?:[^()]++|(?R))*+\)' # Recursive pattern for nested parentheses
matches = regex.findall(pattern, text)
return matches
Case Study: Extracting Product Information from E-commerce Text
One compelling example from the project is extracting product details from unstructured e-commerce descriptions:
Product: Premium Bluetooth Headphones XC-400
SKU: BT-400-BLK
Price: $149.99
Available Colors: Black, Silver, Blue
Features: Noise Cancellation, 30-hour Battery, Water Resistant
Using this regex pattern:
Product: (?<product>.+?)[\r\n]+
SKU: (?<sku>[A-Z0-9\-]+)[\r\n]+
Price: \$(?<price>\d+\.\d{2})[\r\n]+
Available Colors: (?<colors>.+?)[\r\n]+
Features: (?<features>.+)
The code extracts a structured object:
{
"product": "Premium Bluetooth Headphones XC-400",
"sku": "BT-400-BLK",
"price": "149.99",
"colors": "Black, Silver, Blue",
"features": "Noise Cancellation, 30-hour Battery, Water Resistant"
}
Best Practices and Lessons Learned
The project emphasizes several best practices for regex-based data extraction:
- Test with diverse data: Ensure your patterns work with various text formats and edge cases
- Document complex patterns: Add comments explaining the logic behind complex regex
- Break complex patterns into components: Build and test incrementally
- Balance precision and flexibility: Overly specific patterns may break with slight text variations
- Consider preprocessing: Sometimes cleaning text before applying regex yields better results
Future Directions
The “Advance-Regex-For-Data-Mining-Extraction” project continues to evolve with plans to:
- Implement more domain-specific extraction patterns for legal, medical, and technical texts
- Create a pattern library organized by text type and extraction target
- Develop a visual pattern builder to make complex regex more accessible
- Benchmark performance against machine learning approaches for similar extraction tasks
Conclusion
Regular expressions remain a remarkably powerful tool for text mining and data extraction. The techniques demonstrated in this project show how advanced regex can transform unstructured text into structured, analyzable data with precision and efficiency. While newer technologies like NLP models and machine learning techniques offer alternative approaches, the flexibility, speed, and precision of well-crafted regex patterns ensure they’ll remain relevant for data mining tasks well into the future.
By mastering the advanced techniques outlined in this blog post, you’ll be well-equipped to tackle complex text mining challenges and extract meaningful insights from the vast sea of unstructured text data that surrounds us.
This blog post explores the techniques implemented in the Advance-Regex-For-Data-Mining-Extraction project by Tejas K.
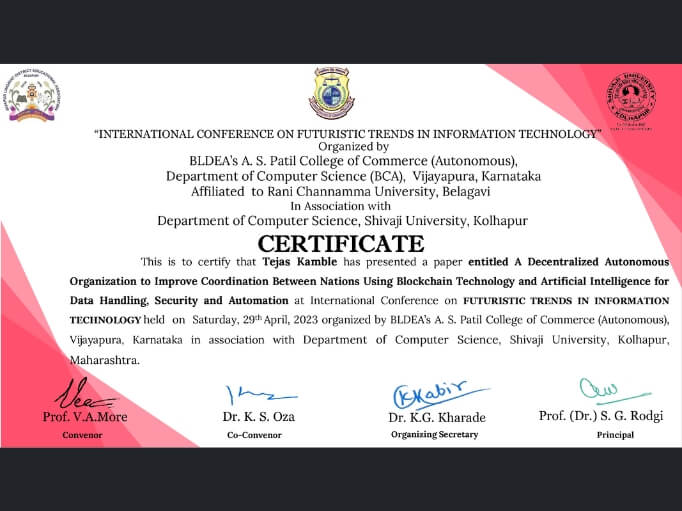
AI and Blockchain Convergence & Transformers based Sentiment Analysis on Live Global News Data.
A Decentralized Autonomous Organization to Improve Coordination Between Nations Using Blockchain Technology, Artificial Intelligence and Natural Language Processing for Sentiment analysis on News Data.
- Client Tejas Kamble
- Date 29 April 2023
- Services AI & Blockchain Technology
Abstract
This paper is about Establishing a Decentralized organization with the Different Countries as members where all the countries will be considered as the node of the blockchain. All the countries in the organization will be treated equally there will not be any superpower amongst them. Therefore, The Organization will gather huge amount of the data from the different countries from all the sectors like health, education, economy, technology, culture, and agriculture which represents the overall development of the countries. All this gathered data will be further analyzed for their positive and negative impacts on all the mentioned sectors. This will give brief idea about situation of an individual country in different areas on that basis, members of the Organizations or we can say all the member countries will decide the reward or penalty case for the respective country. Blockchains have the potential to enhance systems by getting rid of middlemen. Artificial Intelligence will play a major role in this organization as dealing with massive amount of data will be in the frame and to deal with this data, we need AI to improve data integrity of the result which will be used by Smart-Contract for decision making purpose, automating, and optimizing the smart contract. AI promises to remove oversight and increase the objectivity of our systems. This organization offers a framework for participants to work together to create a dataset and host a model that is continuously updated using smart contracts. As data is growing rapidly. AI will manage that data efficiently with less energy consumption
Acceptance Letter 2
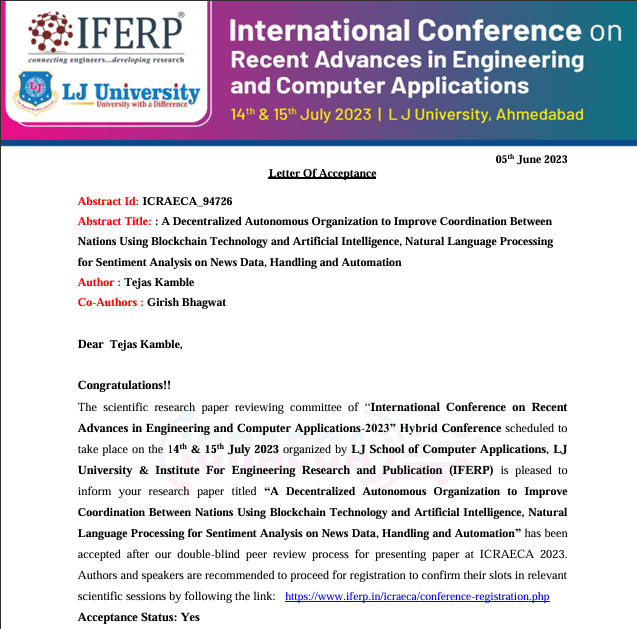