Projects Category: AI
- Home
- AI
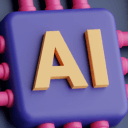
Cloud cost management has evolved from manual spreadsheet tracking to sophisticated AI-driven automation. As organizations increasingly adopt multi-cloud strategies, the complexity of cost optimization grows exponentially. This comprehensive guide explores building agentic AI systems that autonomously manage cloud costs, leveraging open-source models and cutting-edge AI frameworks.
Understanding Agentic AI Systems
Agentic AI systems represent a paradigm shift from traditional reactive AI to proactive, goal-oriented artificial intelligence. Unlike conventional AI models that respond to queries, agentic systems operate autonomously, making decisions and taking actions to achieve specific objectives.
Core Characteristics of Agentic AI
Autonomy: The system operates independently, making decisions without constant human intervention while respecting predefined boundaries and policies.
Goal-Oriented Behavior: Each agent has clear objectives, such as minimizing cloud costs while maintaining performance SLAs, and continuously works toward achieving these goals.
Environmental Awareness: Agents continuously monitor their environment, understanding cloud resource utilization, cost trends, and performance metrics in real-time.
Learning and Adaptation: The system learns from past decisions, improving its cost optimization strategies over time through reinforcement learning and feedback loops.
Multi-Agent Coordination: Different specialized agents collaborate, such as a cost monitoring agent working with a resource optimization agent and a compliance agent.
Architecture of Agentic AI for Cloud Cost Management
Multi-Agent System Design
The architecture consists of specialized agents, each responsible for specific aspects of cloud cost management:
Cost Monitoring Agent: Continuously collects and analyzes billing data from multiple cloud providers, detecting anomalies and trends in real-time.
Resource Optimization Agent: Evaluates resource utilization patterns and makes recommendations or autonomous decisions about rightsizing, scaling, and resource allocation.
Compliance Agent: Ensures all cost optimization actions comply with organizational policies, regulatory requirements, and client-specific constraints.
Forecasting Agent: Uses predictive models to anticipate future costs and resource needs, enabling proactive optimization strategies.
Notification Agent: Manages communication with stakeholders, sending alerts, reports, and recommendations through appropriate channels.
Action Execution Agent: Safely implements approved optimization actions across cloud environments, with rollback capabilities for safety.
Data Flow and Integration Layer
The system integrates with multiple data sources and APIs:
Cloud Provider APIs: Direct integration with AWS Cost Explorer, Azure Cost Management, Google Cloud Billing, and other cloud providers’ cost and usage APIs.
Infrastructure Monitoring: Integration with Prometheus, Grafana, Datadog, or New Relic for real-time performance metrics.
Configuration Management: Connection to Terraform, Ansible, or CloudFormation for infrastructure state management.
Business Systems: Integration with ERP, CRM, and project management systems for cost allocation and chargeback functionality.
Open Source AI Models and Frameworks
Large Language Models Integration
Ollama Integration: Ollama provides local deployment of open-source models, ensuring data privacy and reducing API costs. Key models include:
- Llama 2/3: Excellent for natural language processing tasks, generating cost optimization reports, and explaining complex cost patterns to stakeholders
- Code Llama: Specialized for generating and analyzing infrastructure code, automating Terraform configurations, and creating cost optimization scripts
- Mistral 7B/8x7B: Efficient models for real-time decision making and cost analysis with lower computational requirements
Anthropic Claude Integration: While not open-source, Claude’s API provides sophisticated reasoning capabilities for complex cost optimization scenarios and policy interpretation.
Mistral AI Models: Open-weight models offering excellent performance for cost analysis tasks:
- Mixtral 8x7B: Mixture of experts model providing efficient processing for multi-task cost optimization
- Mistral 7B: Compact model suitable for edge deployment and real-time decision making
Specialized AI Tools and Libraries
Machine Learning Frameworks:
- scikit-learn: For traditional ML tasks like anomaly detection, clustering cost patterns, and regression analysis
- XGBoost/LightGBM: Gradient boosting for accurate cost forecasting and resource usage prediction
- PyTorch/TensorFlow: Deep learning frameworks for complex pattern recognition in cost data
Time Series Analysis:
- Prophet: Facebook’s time series forecasting tool, excellent for predicting cloud costs with seasonal patterns
- ARIMA/SARIMA: Classical time series models for cost trend analysis
- Neural Prophet: Deep learning approach to time series forecasting
Reinforcement Learning:
- Stable Baselines3: Implementation of RL algorithms for autonomous cost optimization decisions
- Ray RLlib: Distributed reinforcement learning for complex multi-agent scenarios
Natural Language Processing:
- Transformers (Hugging Face): Pre-trained models for processing cost reports, policy documents, and generating explanations
- spaCy: Efficient NLP library for text processing and entity extraction from cost documentation
Technical Implementation Stack
Backend Infrastructure
Core Application Framework:
# FastAPI-based microservices architecture
from fastapi import FastAPI, BackgroundTasks
from pydantic import BaseModel
import asyncio
from typing import List, Dict
import httpx
app = FastAPI(title="Agentic Cloud Cost Management")
class CostAgent:
def __init__(self, model_endpoint: str):
self.model_endpoint = model_endpoint
self.client = httpx.AsyncClient()
async def analyze_costs(self, cost_data: Dict):
# Integration with Ollama or other model endpoints
pass
Message Queue and Orchestration:
- Apache Kafka: Real-time data streaming for cost events and optimization triggers
- Celery with Redis: Task queue for asynchronous cost optimization jobs
- Apache Airflow: Workflow orchestration for complex cost management pipelines
Database Architecture:
- InfluxDB: Time-series database for storing cost and usage metrics
- PostgreSQL: Relational database for client configurations, policies, and audit trails
- MongoDB: Document store for unstructured data like cost reports and optimization recommendations
- Redis: Caching layer for frequently accessed cost data and model predictions
AI Model Deployment and Management
Local Model Deployment with Ollama:
# Install and configure Ollama
curl -fsSL https://ollama.ai/install.sh | sh
# Pull required models
ollama pull llama3
ollama pull mistral
ollama pull codellama
# Create custom cost management model
ollama create cost-optimizer -f ./Modelfile
Model Serving Infrastructure:
- TorchServe: Production-ready serving for PyTorch models
- MLflow: Model versioning, experiment tracking, and deployment
- Kubeflow: Kubernetes-native ML workflows
- BentoML: Framework for building AI application APIs
Container Orchestration:
# Kubernetes deployment for AI agents
apiVersion: apps/v1
kind: Deployment
metadata:
name: cost-optimization-agent
spec:
replicas: 3
selector:
matchLabels:
app: cost-agent
template:
metadata:
labels:
app: cost-agent
spec:
containers:
- name: cost-agent
image: cost-optimizer:latest
resources:
requests:
memory: "2Gi"
cpu: "1000m"
limits:
memory: "4Gi"
cpu: "2000m"
Cloud Provider Integration
Multi-Cloud Cost Collection:
import boto3
from azure.mgmt.consumption import ConsumptionManagementClient
from google.cloud import billing
import asyncio
class MultiCloudCostCollector:
def __init__(self):
self.aws_client = boto3.client('ce') # Cost Explorer
self.azure_client = ConsumptionManagementClient(credential, subscription_id)
self.gcp_client = billing.CloudBillingClient()
async def collect_all_costs(self):
tasks = [
self.get_aws_costs(),
self.get_azure_costs(),
self.get_gcp_costs()
]
return await asyncio.gather(*tasks)
Infrastructure as Code Integration:
- Terraform Provider: Custom provider for cost-optimized resource provisioning
- Pulumi: Modern IaC with native programming language support
- CDK (Cloud Development Kit): Define cloud resources using familiar programming languages
Agent Communication and Coordination
Inter-Agent Communication Protocol
Message Passing System:
from dataclasses import dataclass
from enum import Enum
from typing import Any, Dict
class MessageType(Enum):
COST_ALERT = "cost_alert"
OPTIMIZATION_REQUEST = "optimization_request"
ACTION_APPROVAL = "action_approval"
STATUS_UPDATE = "status_update"
@dataclass
class AgentMessage:
sender: str
recipient: str
message_type: MessageType
payload: Dict[str, Any]
timestamp: float
priority: int = 1
class AgentCommunicationHub:
def __init__(self):
self.agents = {}
self.message_queue = asyncio.Queue()
async def route_message(self, message: AgentMessage):
if message.recipient in self.agents:
await self.agents[message.recipient].receive_message(message)
Consensus Mechanisms: Implement voting systems for critical decisions affecting multiple clients or significant cost impacts, ensuring no single agent can make potentially harmful decisions without consensus.
Event-Driven Architecture
Event Streaming with Kafka:
from kafka import KafkaProducer, KafkaConsumer
import json
class CostEventProducer:
def __init__(self):
self.producer = KafkaProducer(
bootstrap_servers=['localhost:9092'],
value_serializer=lambda x: json.dumps(x).encode('utf-8')
)
def emit_cost_event(self, event_type: str, data: Dict):
event = {
'event_type': event_type,
'timestamp': time.time(),
'data': data
}
self.producer.send('cost-events', event)
class OptimizationAgent:
def __init__(self):
self.consumer = KafkaConsumer(
'cost-events',
bootstrap_servers=['localhost:9092'],
auto_offset_reset='latest',
value_deserializer=lambda m: json.loads(m.decode('utf-8'))
)
async def process_events(self):
for message in self.consumer:
await self.handle_cost_event(message.value)
Implementing Intelligent Cost Optimization
Anomaly Detection System
Statistical Anomaly Detection:
import numpy as np
from sklearn.ensemble import IsolationForest
from sklearn.preprocessing import StandardScaler
class CostAnomalyDetector:
def __init__(self):
self.model = IsolationForest(contamination=0.1, random_state=42)
self.scaler = StandardScaler()
self.is_trained = False
def train(self, historical_costs: np.ndarray):
scaled_costs = self.scaler.fit_transform(historical_costs)
self.model.fit(scaled_costs)
self.is_trained = True
def detect_anomalies(self, current_costs: np.ndarray) -> List[bool]:
if not self.is_trained:
raise ValueError("Model must be trained first")
scaled_costs = self.scaler.transform(current_costs)
anomaly_scores = self.model.decision_function(scaled_costs)
return self.model.predict(scaled_costs) == -1
Deep Learning Anomaly Detection:
import torch
import torch.nn as nn
from torch.utils.data import DataLoader, TensorDataset
class CostAutoencoderAnomalyDetector(nn.Module):
def __init__(self, input_dim: int, hidden_dims: List[int]):
super().__init__()
# Encoder
encoder_layers = []
current_dim = input_dim
for hidden_dim in hidden_dims:
encoder_layers.extend([
nn.Linear(current_dim, hidden_dim),
nn.ReLU(),
nn.BatchNorm1d(hidden_dim)
])
current_dim = hidden_dim
# Decoder
decoder_layers = []
for i in range(len(hidden_dims) - 1, -1, -1):
decoder_layers.extend([
nn.Linear(current_dim, hidden_dims[i] if i > 0 else input_dim),
nn.ReLU() if i > 0 else nn.Sigmoid()
])
current_dim = hidden_dims[i] if i > 0 else input_dim
self.encoder = nn.Sequential(*encoder_layers)
self.decoder = nn.Sequential(*decoder_layers)
def forward(self, x):
encoded = self.encoder(x)
decoded = self.decoder(encoded)
return decoded
Predictive Cost Modeling
Time Series Forecasting with Neural Networks:
import pytorch_lightning as pl
from pytorch_forecasting import TimeSeriesDataSet, NBeats
import pandas as pd
class CostForecastingModel:
def __init__(self):
self.model = None
self.training_data = None
def prepare_data(self, cost_history: pd.DataFrame):
# Convert cost history to time series format
self.training_data = TimeSeriesDataSet(
cost_history,
time_idx="day",
target="cost",
group_ids=["client_id", "service"],
min_encoder_length=30,
max_encoder_length=90,
min_prediction_length=7,
max_prediction_length=30,
static_categoricals=["client_id", "service"],
time_varying_known_reals=["day_of_week", "month", "quarter"],
time_varying_unknown_reals=["cost"],
)
def train_model(self):
self.model = NBeats.from_dataset(
self.training_data,
learning_rate=3e-2,
weight_decay=1e-8,
widths=[32, 512],
backcast_loss_ratio=1.0,
)
trainer = pl.Trainer(max_epochs=50, gpus=1)
trainer.fit(self.model, self.training_data)
Reinforcement Learning for Cost Optimization
Deep Q-Network for Resource Allocation:
import gym
import torch
import torch.nn as nn
import numpy as np
from collections import deque
import random
class CloudCostEnvironment(gym.Env):
def __init__(self, client_config: Dict):
super().__init__()
self.client_config = client_config
self.action_space = gym.spaces.Discrete(5) # Scale up, down, maintain, stop, start
self.observation_space = gym.spaces.Box(
low=0, high=np.inf, shape=(10,), dtype=np.float32
)
self.current_cost = 0
self.performance_score = 1.0
def step(self, action):
# Simulate cost and performance changes based on action
cost_change, performance_change = self._simulate_action(action)
self.current_cost += cost_change
self.performance_score += performance_change
# Calculate reward (negative cost + performance bonus)
reward = -cost_change + (performance_change * 10)
# Check if episode is done
done = self.current_cost > self.client_config['max_budget']
obs = self._get_observation()
return obs, reward, done, {}
def _simulate_action(self, action):
# Implementation of cost and performance simulation
pass
class DQNAgent:
def __init__(self, state_size, action_size):
self.state_size = state_size
self.action_size = action_size
self.memory = deque(maxlen=2000)
self.epsilon = 1.0
self.epsilon_min = 0.01
self.epsilon_decay = 0.995
self.learning_rate = 0.001
self.q_network = self._build_model()
self.target_network = self._build_model()
def _build_model(self):
model = nn.Sequential(
nn.Linear(self.state_size, 64),
nn.ReLU(),
nn.Linear(64, 64),
nn.ReLU(),
nn.Linear(64, self.action_size)
)
return model
Multi-Tenant Architecture for Service Organizations
Client Isolation and Security
Tenant Management System:
from sqlalchemy import create_engine, Column, String, Integer, JSON, DateTime
from sqlalchemy.ext.declarative import declarative_base
from sqlalchemy.orm import sessionmaker
import hashlib
import secrets
Base = declarative_base()
class Tenant(Base):
__tablename__ = 'tenants'
id = Column(String, primary_key=True)
name = Column(String, nullable=False)
api_key_hash = Column(String, nullable=False)
config = Column(JSON, default={})
created_at = Column(DateTime)
def generate_api_key(self):
api_key = secrets.token_urlsafe(32)
self.api_key_hash = hashlib.sha256(api_key.encode()).hexdigest()
return api_key
class TenantManager:
def __init__(self, database_url: str):
self.engine = create_engine(database_url)
self.SessionLocal = sessionmaker(bind=self.engine)
def create_tenant(self, name: str, config: Dict) -> Tuple[str, str]:
tenant = Tenant(
id=secrets.token_urlsafe(16),
name=name,
config=config
)
api_key = tenant.generate_api_key()
with self.SessionLocal() as session:
session.add(tenant)
session.commit()
return tenant.id, api_key
Data Isolation with Row-Level Security:
-- PostgreSQL RLS for tenant data isolation
CREATE POLICY tenant_isolation ON cost_data
FOR ALL TO application_role
USING (tenant_id = current_setting('app.current_tenant'));
-- Function to set tenant context
CREATE OR REPLACE FUNCTION set_tenant_context(tenant_id TEXT)
RETURNS VOID AS $$
BEGIN
PERFORM set_config('app.current_tenant', tenant_id, true);
END;
$$ LANGUAGE plpgsql;
Scalable Agent Deployment
Kubernetes Operator for Agent Management:
apiVersion: apiextensions.k8s.io/v1
kind: CustomResourceDefinition
metadata:
name: costagents.ai.company.com
spec:
group: ai.company.com
versions:
- name: v1
served: true
storage: true
schema:
openAPIV3Schema:
type: object
properties:
spec:
type: object
properties:
tenantId:
type: string
agentType:
type: string
enum: ["cost-monitor", "optimizer", "forecaster"]
resources:
type: object
properties:
cpu:
type: string
memory:
type: string
modelConfig:
type: object
scope: Namespaced
names:
plural: costagents
singular: costagent
kind: CostAgent
Advanced AI Integration Patterns
Multi-Model Ensemble Approach
Model Router and Ensemble:
from typing import List, Dict, Any
import numpy as np
from sklearn.metrics import mean_squared_error
class ModelEnsemble:
def __init__(self):
self.models = {}
self.weights = {}
self.performance_history = {}
def add_model(self, name: str, model: Any, weight: float = 1.0):
self.models[name] = model
self.weights[name] = weight
self.performance_history[name] = deque(maxlen=100)
def predict(self, data: Any) -> Dict[str, Any]:
predictions = {}
for name, model in self.models.items():
try:
if name.startswith('ollama_'):
# Handle Ollama model inference
predictions[name] = self._query_ollama_model(model, data)
elif name.startswith('mistral_'):
# Handle Mistral model inference
predictions[name] = self._query_mistral_model(model, data)
else:
# Handle scikit-learn or other models
predictions[name] = model.predict(data)
except Exception as e:
print(f"Model {name} failed: {e}")
continue
# Weight predictions based on recent performance
final_prediction = self._weighted_ensemble(predictions)
return final_prediction
def _weighted_ensemble(self, predictions: Dict) -> Any:
# Implement weighted averaging based on model performance
pass
class IntelligentModelRouter:
def __init__(self):
self.routing_rules = {}
self.model_capabilities = {}
def route_request(self, request_type: str, complexity: float, latency_req: float):
# Route to appropriate model based on request characteristics
if request_type == "cost_analysis" and complexity < 0.5:
return "mistral_7b" # Fast, efficient model
elif request_type == "optimization" and latency_req > 10:
return "llama3_70b" # More capable model when time allows
else:
return "ensemble" # Use ensemble for complex decisions
Real-Time Streaming AI
Streaming Cost Analysis with Kafka Streams:
from kafka import KafkaConsumer, KafkaProducer
import json
import asyncio
from typing import AsyncGenerator
class StreamingCostAnalyzer:
def __init__(self, model_endpoint: str):
self.model_endpoint = model_endpoint
self.consumer = KafkaConsumer(
'cost-events',
bootstrap_servers=['localhost:9092'],
value_deserializer=lambda m: json.loads(m.decode('utf-8'))
)
self.producer = KafkaProducer(
bootstrap_servers=['localhost:9092'],
value_serializer=lambda x: json.dumps(x).encode('utf-8')
)
async def process_cost_stream(self):
async for message in self._async_consume():
# Real-time cost analysis
analysis_result = await self._analyze_cost_event(message.value)
# Emit results
await self._emit_analysis_result(analysis_result)
async def _analyze_cost_event(self, event: Dict) -> Dict:
# Use streaming-optimized model for real-time analysis
prompt = f"Analyze this cost event: {json.dumps(event)}"
response = await self._query_model(prompt)
return {
'event_id': event['id'],
'analysis': response,
'timestamp': time.time(),
'confidence': self._calculate_confidence(response)
}
Monitoring and Observability
Comprehensive Monitoring Stack
Agent Health Monitoring:
from prometheus_client import Counter, Histogram, Gauge, start_http_server
import time
import logging
class AgentMetrics:
def __init__(self):
self.decision_counter = Counter('agent_decisions_total', 'Total decisions made', ['agent_type', 'decision_type'])
self.response_time = Histogram('agent_response_time_seconds', 'Agent response time')
self.active_agents = Gauge('active_agents', 'Number of active agents', ['agent_type'])
self.cost_savings = Counter('cost_savings_total', 'Total cost savings achieved', ['client_id'])
def record_decision(self, agent_type: str, decision_type: str):
self.decision_counter.labels(agent_type=agent_type, decision_type=decision_type).inc()
def record_response_time(self, duration: float):
self.response_time.observe(duration)
# Distributed tracing with OpenTelemetry
from opentelemetry import trace
from opentelemetry.exporter.jaeger.thrift import JaegerExporter
from opentelemetry.sdk.trace import TracerProvider
from opentelemetry.sdk.trace.export import BatchSpanProcessor
tracer = trace.get_tracer(__name__)
class TracedCostAgent:
def __init__(self):
self.metrics = AgentMetrics()
async def make_cost_decision(self, request: Dict):
with tracer.start_as_current_span("cost_decision") as span:
span.set_attribute("client_id", request['client_id'])
span.set_attribute("request_type", request['type'])
start_time = time.time()
try:
result = await self._process_request(request)
span.set_attribute("decision", result['decision'])
return result
finally:
duration = time.time() - start_time
self.metrics.record_response_time(duration)
Automated Testing and Validation
Agent Behavior Testing:
import pytest
from unittest.mock import AsyncMock, patch
import asyncio
class TestCostOptimizationAgent:
@pytest.fixture
async def agent(self):
return CostOptimizationAgent(
model_endpoint="http://localhost:11434",
client_config={"max_cost": 1000, "min_performance": 0.8}
)
@pytest.mark.asyncio
async def test_cost_spike_detection(self, agent):
# Mock cost data with spike
cost_data = {
'current_cost': 1500,
'historical_average': 800,
'client_id': 'test_client'
}
with patch.object(agent, '_query_model') as mock_query:
mock_query.return_value = {
'anomaly_detected': True,
'recommended_action': 'scale_down',
'confidence': 0.95
}
result = await agent.analyze_cost_anomaly(cost_data)
assert result['anomaly_detected'] is True
assert result['recommended_action'] == 'scale_down'
@pytest.mark.asyncio
async def test_multi_agent_coordination(self):
# Test agent communication and coordination
pass
# Load testing for agent scalability
from locust import HttpUser, task, between
class AgentLoadTest(HttpUser):
wait_time = between(1, 3)
@task
def analyze_costs(self):
cost_data = {
'client_id': 'load_test_client',
'costs': [100, 150, 200, 180, 220],
'timestamp': time.time()
}
self.client.post("/api/v1/analyze-costs", json=cost_data)
@task
def get_recommendations(self):
self.client.get("/api/v1/recommendations/load_test_client")
Business Intelligence and Reporting
Automated Report Generation
AI-Powered Cost Reports:
from jinja2 import Template
import matplotlib.pyplot as plt
import pandas as pd
from datetime import datetime, timedelta
class IntelligentReporter:
def __init__(self, llm_client):
self.llm_client = llm_client
self.report_templates = {}
async def generate_executive_summary(self, cost_data: Dict, client_id: str) -> str:
# Use LLM to generate natural language insights
prompt = f"""
Based on the following cost data for client {client_id}, generate an executive summary:
Total Cost: ${cost_data['total_cost']:,.2f}
Month-over-Month Change: {cost_data['mom_change']:.1f}%
Top Cost Drivers: {', '.join(cost_data['top_drivers'])}
Optimization Opportunities: {cost_data['savings_potential']}
Generate a professional executive summary highlighting key insights and recommendations.
"""
response = await self.llm_client.generate(prompt)
return response
async def create_comprehensive_report(self, client_id: str, period: str) -> Dict:
# Gather data from multiple sources
cost_data = await self._fetch_cost_data(client_id, period)
savings_data = await self._fetch_savings_data(client_id, period)
forecast_data = await self._generate_forecast(client_id)
# Generate visualizations
charts = await self._create_charts(cost_data)
# Generate AI insights
executive_summary = await self.generate_executive_summary(cost_data, client_id)
recommendations = await self._generate_recommendations(cost_data, savings_data)
return {
'client_id': client_id,
'period': period,
'executive_summary': executive_summary,
'cost_breakdown': cost_data,
'savings_achieved': savings_data,
'forecast': forecast_data,
'recommendations': recommendations,
'charts': charts,
'generated_at': datetime.now().isoformat()
}
Security and Compliance Framework
AI Model Security
Model Input Validation and Sanitization:
import re
from typing import Any, Dict
import bleach
class SecureModelInterface:
def __init__(self):
self.input_validators = {
'cost_analysis': self._validate_cost_input,
'optimization': self._validate_optimization_input
}
self.max_input_length = 10000
self.allowed_tags = []
def sanitize_input(self, input_data: Any) -> Any:
if isinstance(input_data, str):
# Remove potentially harmful content
sanitized = bleach.clean(input_data, tags=self.allowed_tags, strip=True)
# Validate length
if len(sanitized) > self.max_input_length:
raise ValueError("Input too long")
# Check for injection patterns
if self._detect_injection_patterns(sanitized):
raise ValueError("Potentially malicious input detected")
return sanitized
elif isinstance(input_data, dict):
return {k: self.sanitize_input(v) for k, v in input_data.items()}
return input_data
def _detect_injection_patterns(self, text: str) -> bool:
# Check for common injection patterns
patterns = [
r'<script.*?>.*?</script>',
r'javascript:',
r'data:text/html',
r'vbscript:',
r'onload\s*=',
r'onerror\s*='
]
for pattern in patterns:
if re.search(pattern, text, re.IGNORECASE | re.DOTALL):
return True
return False
# Audit logging for all AI decisions
class AuditLogger:
def __init__(self, log_level: str = "INFO"):
self.logger = logging.getLogger("agent_audit")
self.logger.setLevel(getattr(logging, log_level))
handler = logging.StreamHandler()
formatter = logging.Formatter(
'%(asctime)s - %(name)s - %(levelname)s - %(message)s'
)
handler.setFormatter(formatter)
self.logger.addHandler(handler)
def log_decision(self, agent_id: str, client_id: str, decision: Dict, reasoning: str):
audit_entry = {
'timestamp': datetime.now().isoformat(),
'agent_id': agent_id,
'client_id': client_id,
'decision': decision,
'reasoning': reasoning,
'model_version': self._get_model_version(),
'confidence_score': decision.get('confidence', 0.0)
}
self.logger.info(f"DECISION: {json.dumps(audit_entry)}")
def log_action_taken(self, agent_id: str, client_id: str, action: Dict, result: Dict):
audit_entry = {
'timestamp': datetime.now().isoformat(),
'agent_id': agent_id,
'client_id': client_id,
'action': action,
'result': result,
'success': result.get('success', False)
}
self.logger.info(f"ACTION: {json.dumps(audit_entry)}")
Compliance and Governance
Policy Engine for Cost Management:
from dataclasses import dataclass
from typing import List, Callable, Any
from enum import Enum
class PolicyType(Enum):
COST_LIMIT = "cost_limit"
RESOURCE_CONSTRAINT = "resource_constraint"
APPROVAL_REQUIRED = "approval_required"
COMPLIANCE_RULE = "compliance_rule"
@dataclass
class Policy:
id: str
name: str
policy_type: PolicyType
conditions: Dict[str, Any]
actions: List[str]
priority: int
active: bool = True
class PolicyEngine:
def __init__(self):
self.policies: Dict[str, Policy] = {}
self.rule_evaluators: Dict[PolicyType, Callable] = {
PolicyType.COST_LIMIT: self._evaluate_cost_limit,
PolicyType.RESOURCE_CONSTRAINT: self._evaluate_resource_constraint,
PolicyType.APPROVAL_REQUIRED: self._evaluate_approval_requirement,
PolicyType.COMPLIANCE_RULE: self._evaluate_compliance_rule
}
def add_policy(self, policy: Policy):
self.policies[policy.id] = policy
async def evaluate_action(self, action: Dict, context: Dict) -> Dict:
"""Evaluate if an action is allowed based on active policies"""
evaluation_results = []
# Sort policies by priority
sorted_policies = sorted(
[p for p in self.policies.values() if p.active],
key=lambda x: x.priority,
reverse=True
)
for policy in sorted_policies:
evaluator = self.rule_evaluators.get(policy.policy_type)
if evaluator:
result = await evaluator(policy, action, context)
evaluation_results.append({
'policy_id': policy.id,
'policy_name': policy.name,
'allowed': result['allowed'],
'reason': result['reason'],
'required_approvals': result.get('required_approvals', [])
})
# Determine final decision
allowed = all(result['allowed'] for result in evaluation_results)
required_approvals = []
for result in evaluation_results:
required_approvals.extend(result.get('required_approvals', []))
return {
'allowed': allowed,
'policy_evaluations': evaluation_results,
'required_approvals': list(set(required_approvals))
}
async def _evaluate_cost_limit(self, policy: Policy, action: Dict, context: Dict) -> Dict:
current_cost = context.get('current_monthly_cost', 0)
projected_cost = current_cost + action.get('cost_impact', 0)
cost_limit = policy.conditions.get('monthly_limit', float('inf'))
if projected_cost > cost_limit:
return {
'allowed': False,
'reason': f"Action would exceed monthly cost limit of ${cost_limit:,.2f}"
}
return {'allowed': True, 'reason': 'Within cost limits'}
async def _evaluate_resource_constraint(self, policy: Policy, action: Dict, context: Dict) -> Dict:
# Implement resource constraint evaluation
return {'allowed': True, 'reason': 'Resource constraints satisfied'}
async def _evaluate_approval_requirement(self, policy: Policy, action: Dict, context: Dict) -> Dict:
cost_impact = action.get('cost_impact', 0)
approval_threshold = policy.conditions.get('cost_threshold', 1000)
if abs(cost_impact) > approval_threshold:
return {
'allowed': False,
'reason': f"Action requires approval (cost impact: ${cost_impact:,.2f})",
'required_approvals': policy.conditions.get('approvers', ['manager'])
}
return {'allowed': True, 'reason': 'No approval required'}
async def _evaluate_compliance_rule(self, policy: Policy, action: Dict, context: Dict) -> Dict:
# Implement compliance rule evaluation (PCI, HIPAA, SOX, etc.)
compliance_tags = context.get('compliance_tags', [])
required_tags = policy.conditions.get('required_tags', [])
if not all(tag in compliance_tags for tag in required_tags):
return {
'allowed': False,
'reason': f"Missing required compliance tags: {set(required_tags) - set(compliance_tags)}"
}
return {'allowed': True, 'reason': 'Compliance requirements met'}
Advanced Model Integration Techniques
Hybrid Local-Cloud Model Architecture
Intelligent Model Selection:
class HybridModelOrchestrator:
def __init__(self):
self.local_models = {
'ollama_llama3': 'http://localhost:11434/api/generate',
'ollama_mistral': 'http://localhost:11434/api/generate',
'ollama_codellama': 'http://localhost:11434/api/generate'
}
self.cloud_models = {
'claude': 'https://api.anthropic.com/v1/messages',
'mistral_large': 'https://api.mistral.ai/v1/chat/completions'
}
self.model_characteristics = {
'ollama_llama3': {'latency': 'low', 'cost': 'free', 'privacy': 'high', 'capability': 'medium'},
'ollama_mistral': {'latency': 'low', 'cost': 'free', 'privacy': 'high', 'capability': 'medium'},
'claude': {'latency': 'medium', 'cost': 'medium', 'privacy': 'medium', 'capability': 'high'},
'mistral_large': {'latency': 'medium', 'cost': 'low', 'privacy': 'medium', 'capability': 'high'}
}
async def select_optimal_model(self, task_type: str, requirements: Dict) -> str:
"""Select the best model based on task requirements"""
# Define selection criteria
if requirements.get('privacy_critical', False):
# Use only local models for sensitive data
candidates = list(self.local_models.keys())
else:
candidates = list(self.local_models.keys()) + list(self.cloud_models.keys())
# Score models based on requirements
scored_models = []
for model in candidates:
score = self._calculate_model_score(model, task_type, requirements)
scored_models.append((model, score))
# Return best scoring model
return max(scored_models, key=lambda x: x[1])[0]
def _calculate_model_score(self, model: str, task_type: str, requirements: Dict) -> float:
characteristics = self.model_characteristics[model]
score = 0.0
# Latency scoring
if requirements.get('max_latency', 10) < 2:
score += 3 if characteristics['latency'] == 'low' else 0
# Cost scoring
if requirements.get('cost_sensitive', False):
score += 2 if characteristics['cost'] == 'free' else 0
# Privacy scoring
if requirements.get('privacy_critical', False):
score += 4 if characteristics['privacy'] == 'high' else 0
# Capability scoring for complex tasks
if task_type in ['complex_analysis', 'strategic_planning']:
score += 3 if characteristics['capability'] == 'high' else 1
return score
async def execute_with_fallback(self, prompt: str, task_type: str, requirements: Dict) -> Dict:
"""Execute request with automatic fallback to alternative models"""
primary_model = await self.select_optimal_model(task_type, requirements)
try:
return await self._query_model(primary_model, prompt)
except Exception as e:
# Fallback strategy
fallback_models = [m for m in self.model_characteristics.keys() if m != primary_model]
for fallback_model in fallback_models:
try:
result = await self._query_model(fallback_model, prompt)
# Log fallback usage
self._log_fallback(primary_model, fallback_model, str(e))
return result
except Exception:
continue
raise Exception("All models failed")
async def _query_model(self, model_name: str, prompt: str) -> Dict:
if model_name in self.local_models:
return await self._query_ollama(model_name, prompt)
else:
return await self._query_cloud_model(model_name, prompt)
async def _query_ollama(self, model_name: str, prompt: str) -> Dict:
model_key = model_name.split('_')[1] # Extract model name (llama3, mistral, etc.)
payload = {
"model": model_key,
"prompt": prompt,
"stream": False,
"options": {
"temperature": 0.1,
"top_p": 0.9,
"num_predict": 512
}
}
async with httpx.AsyncClient() as client:
response = await client.post(
self.local_models[model_name],
json=payload,
timeout=30.0
)
response.raise_for_status()
return response.json()
Advanced Prompt Engineering for Cost Management
Domain-Specific Prompt Templates:
class CostManagementPrompts:
def __init__(self):
self.templates = {
'anomaly_analysis': """
You are a cloud cost optimization expert. Analyze the following cost data and identify anomalies:
Cost Data:
{cost_data}
Context:
- Historical average: ${historical_avg}
- Current cost: ${current_cost}
- Time period: {time_period}
- Services involved: {services}
Please provide:
1. Anomaly detection (Yes/No) with confidence score
2. Root cause analysis
3. Recommended immediate actions
4. Potential cost impact if not addressed
Format your response as JSON with the following structure:
{{
"anomaly_detected": boolean,
"confidence_score": float,
"root_cause": "string",
"immediate_actions": ["action1", "action2"],
"cost_impact": float,
"reasoning": "detailed explanation"
}}
""",
'optimization_recommendation': """
You are an expert cloud architect focused on cost optimization. Given the following resource utilization data, provide optimization recommendations:
Resource Data:
{resource_data}
Current Configuration:
{current_config}
Performance Requirements:
{performance_requirements}
Budget Constraints:
{budget_constraints}
Provide specific, actionable recommendations that:
1. Reduce costs while maintaining or improving performance
2. Consider business requirements and constraints
3. Include estimated cost savings
4. Prioritize recommendations by impact and effort
Format as JSON:
{{
"recommendations": [
{{
"action": "string",
"estimated_savings": float,
"effort_level": "low|medium|high",
"risk_level": "low|medium|high",
"implementation_steps": ["step1", "step2"],
"expected_timeline": "string"
}}
],
"total_potential_savings": float,
"implementation_priority": ["rec1", "rec2", "rec3"]
}}
""",
'executive_summary': """
Create an executive summary for cloud cost management based on the following data:
Financial Summary:
- Total monthly cost: ${total_cost}
- Month-over-month change: {mom_change}%
- Year-over-year change: {yoy_change}%
- Budget utilization: {budget_utilization}%
Key Metrics:
{key_metrics}
Optimization Actions Taken:
{actions_taken}
Upcoming Initiatives:
{upcoming_initiatives}
Write a professional executive summary that:
1. Highlights key financial performance
2. Explains significant changes
3. Summarizes optimization impact
4. Outlines strategic recommendations
5. Uses business-friendly language (avoid technical jargon)
Keep it concise (200-300 words) and focus on business value.
"""
}
def get_prompt(self, template_name: str, **kwargs) -> str:
if template_name not in self.templates:
raise ValueError(f"Template {template_name} not found")
return self.templates[template_name].format(**kwargs)
def create_chain_of_thought_prompt(self, base_prompt: str, thinking_steps: List[str]) -> str:
"""Create a chain-of-thought prompt for complex reasoning"""
cot_prefix = """
Before providing your final answer, think through this step by step:
"""
for i, step in enumerate(thinking_steps, 1):
cot_prefix += f"{i}. {step}\n"
cot_prefix += "\nNow, work through each step and provide your final answer:\n\n"
return cot_prefix + base_prompt
Multi-Modal AI Integration
Integration with Vision Models for Infrastructure Diagrams:
class MultiModalCostAnalyzer:
def __init__(self):
self.vision_models = {
'diagram_analysis': 'llava:latest', # Ollama vision model
'chart_interpretation': 'bakllava:latest'
}
async def analyze_architecture_diagram(self, image_path: str, cost_context: Dict) -> Dict:
"""Analyze infrastructure diagrams to identify cost optimization opportunities"""
# Read image
with open(image_path, 'rb') as img_file:
image_data = base64.b64encode(img_file.read()).decode()
prompt = f"""
Analyze this cloud architecture diagram and identify potential cost optimization opportunities.
Consider the following cost context:
- Current monthly spend: ${cost_context['monthly_spend']}
- Top cost drivers: {', '.join(cost_context['top_drivers'])}
- Performance requirements: {cost_context['performance_requirements']}
Look for:
1. Over-provisioned resources
2. Unnecessary redundancy
3. Inefficient data flow patterns
4. Missing cost optimization services
5. Opportunities for serverless migration
Provide specific, actionable recommendations with estimated cost impact.
"""
# Query vision model through Ollama
response = await self._query_vision_model('llava:latest', prompt, image_data)
return {
'diagram_analysis': response,
'cost_optimization_score': self._calculate_optimization_score(response),
'recommended_actions': self._extract_actions(response)
}
async def interpret_cost_charts(self, chart_images: List[str]) -> Dict:
"""Interpret cost trend charts and graphs"""
interpretations = []
for chart_path in chart_images:
with open(chart_path, 'rb') as img_file:
image_data = base64.b64encode(img_file.read()).decode()
prompt = """
Analyze this cost chart/graph and provide insights:
1. Identify trends and patterns
2. Spot anomalies or unusual spikes
3. Determine seasonality effects
4. Suggest areas for investigation
5. Provide forecasting insights
Be specific about time periods, cost values, and percentage changes you observe.
"""
interpretation = await self._query_vision_model('bakllava:latest', prompt, image_data)
interpretations.append(interpretation)
return {
'chart_interpretations': interpretations,
'combined_insights': await self._synthesize_chart_insights(interpretations)
}
async def _query_vision_model(self, model: str, prompt: str, image_data: str) -> str:
payload = {
"model": model,
"prompt": prompt,
"images": [image_data],
"stream": False
}
async with httpx.AsyncClient() as client:
response = await client.post(
"http://localhost:11434/api/generate",
json=payload,
timeout=60.0
)
response.raise_for_status()
return response.json()['response']
Performance Optimization and Scaling
Distributed Agent Architecture
Agent Cluster Management:
import asyncio
import aioredis
from typing import List, Dict, Any
from dataclasses import dataclass, asdict
from datetime import datetime, timedelta
@dataclass
class AgentNode:
node_id: str
agent_type: str
status: str
last_heartbeat: datetime
current_load: float
max_capacity: int
client_assignments: List[str]
class DistributedAgentManager:
def __init__(self, redis_url: str):
self.redis_url = redis_url
self.redis = None
self.local_agents: Dict[str, Any] = {}
self.node_id = secrets.token_urlsafe(8)
async def initialize(self):
self.redis = await aioredis.from_url(self.redis_url)
await self.register_node()
# Start background tasks
asyncio.create_task(self.heartbeat_loop())
asyncio.create_task(self.load_balancer_loop())
async def register_node(self):
"""Register this node in the distributed cluster"""
node_info = AgentNode(
node_id=self.node_id,
agent_type="multi_purpose",
status="active",
last_heartbeat=datetime.now(),
current_load=0.0,
max_capacity=100,
client_assignments=[]
)
await self.redis.hset(
"agent_nodes",
self.node_id,
json.dumps(asdict(node_info), default=str)
)
async def heartbeat_loop(self):
"""Send periodic heartbeats to maintain cluster membership"""
while True:
try:
await self.redis.hset(
"agent_nodes",
self.node_id,
json.dumps({
"node_id": self.node_id,
"status": "active",
"last_heartbeat": datetime.now().isoformat(),
"current_load": self.calculate_current_load(),
"client_assignments": list(self.local_agents.keys())
})
)
await asyncio.sleep(30) # Heartbeat every 30 seconds
except Exception as e:
print(f"Heartbeat error: {e}")
await asyncio.sleep(5)
async def distribute_work(self, task: Dict) -> str:
"""Distribute work to the most appropriate agent node"""
# Get all active nodes
nodes = await self.get_active_nodes()
# Select best node based on load and capabilities
best_node = self.select_optimal_node(nodes, task)
if best_node == self.node_id:
# Execute locally
return await self.execute_local_task(task)
else:
# Send to remote node
return await self.send_remote_task(best_node, task)
def select_optimal_node(self, nodes: List[Dict], task: Dict) -> str:
"""Select the optimal node for task execution"""
# Simple load-based selection (can be enhanced with ML)
available_nodes = [
node for node in nodes
if node['current_load'] < 0.8 and node['status'] == 'active'
]
if not available_nodes:
return self.node_id # Fallback to local execution
# Select node with lowest load
best_node = min(available_nodes, key=lambda x: x['current_load'])
return best_node['node_id']
async def get_active_nodes(self) -> List[Dict]:
"""Get list of all active agent nodes"""
node_data = await self.redis.hgetall("agent_nodes")
nodes = []
for node_id, data in node_data.items():
node_info = json.loads(data)
# Check if node is still alive (heartbeat within last 2 minutes)
last_heartbeat = datetime.fromisoformat(node_info['last_heartbeat'])
if datetime.now() - last_heartbeat < timedelta(minutes=2):
nodes.append(node_info)
return nodes
class AdaptiveLoadBalancer:
def __init__(self):
self.load_history = deque(maxlen=100)
self.response_times = deque(maxlen=100)
self.error_rates = deque(maxlen=100)
def calculate_node_score(self, node: Dict, task_type: str) -> float:
"""Calculate a score for node selection based on multiple factors"""
# Base score from current load (lower is better)
load_score = 1.0 - node['current_load']
# Historical performance score
performance_score = self.get_historical_performance(node['node_id'])
# Task affinity score (some nodes might be better for certain tasks)
affinity_score = self.get_task_affinity(node['node_id'], task_type)
# Weighted combination
total_score = (load_score * 0.4 + performance_score * 0.4 + affinity_score * 0.2)
return total_score
def get_historical_performance(self, node_id: str) -> float:
# Implementation would track historical performance metrics
return 0.8 # Placeholder
def get_task_affinity(self, node_id: str, task_type: str) -> float:
# Some nodes might be optimized for specific task types
return 0.5 # Placeholder
Caching and Optimization Strategies
Intelligent Caching for AI Responses:
import hashlib
from typing import Optional, Tuple
import pickle
import asyncio
class IntelligentCache:
def __init__(self, redis_client, ttl_seconds: int = 3600):
self.redis = redis_client
self.ttl = ttl_seconds
self.hit_rate_window = deque(maxlen=1000)
async def get_or_compute(self,
key_data: Dict,
compute_func: Callable,
cache_strategy: str = 'standard') -> Tuple[Any, bool]:
"""Get cached result or compute new one with intelligent caching strategies"""
cache_key = self._generate_cache_key(key_data)
# Try to get from cache
cached_result = await self._get_cached_result(cache_key)
if cached_result is not None:
self.hit_rate_window.append(1) # Cache hit
return cached_result, True
# Cache miss - compute result
self.hit_rate_window.append(0) # Cache miss
result = await compute_func()
# Apply caching strategy
if cache_strategy == 'standard':
await self._cache_result(cache_key, result, self.ttl)
elif cache_strategy == 'adaptive':
ttl = await self._calculate_adaptive_ttl(key_data, result)
await self._cache_result(cache_key, result, ttl)
elif cache_strategy == 'predictive':
await self._predictive_cache(key_data, result)
return result, False
def _generate_cache_key(self, key_data: Dict) -> str:
"""Generate deterministic cache key from input data"""
# Sort keys to ensure consistent ordering
sorted_data = json.dumps(key_data, sort_keys=True)
# Create hash
return hashlib.sha256(sorted_data.encode()).hexdigest()
async def _get_cached_result(self, cache_key: str) -> Optional[Any]:
"""Retrieve result from cache"""
try:
cached_data = await self.redis.get(f"cache:{cache_key}")
if cached_data:
return pickle.loads(cached_data)
except Exception as e:
print(f"Cache retrieval error: {e}")
return None
async def _cache_result(self, cache_key: str, result: Any, ttl: int):
"""Store result in cache"""
try:
serialized_result = pickle.dumps(result)
await self.redis.setex(f"cache:{cache_key}", ttl, serialized_result)
except Exception as e:
print(f"Cache storage error: {e}")
async def _calculate_adaptive_ttl(self, key_data: Dict, result: Any) -> int:
"""Calculate adaptive TTL based on data characteristics"""
base_ttl = self.ttl
# Adjust TTL based on result confidence
if isinstance(result, dict) and 'confidence' in result:
confidence = result['confidence']
# Higher confidence = longer TTL
ttl_multiplier = 0.5 + (confidence * 1.5)
base_ttl = int(base_ttl * ttl_multiplier)
# Adjust based on data volatility
if 'real_time' in key_data and key_data['real_time']:
base_ttl = min(base_ttl, 300) # Max 5 minutes for real-time data
# Adjust based on cost of computation
computation_cost = key_data.get('computation_cost', 'medium')
if computation_cost == 'high':
base_ttl *= 2 # Cache longer for expensive computations
elif computation_cost == 'low':
base_ttl = int(base_ttl * 0.5) # Shorter cache for cheap computations
return max(60, min(base_ttl, 86400)) # Between 1 minute and 1 day
async def _predictive_cache(self, key_data: Dict, result: Any):
"""Implement predictive caching for likely future requests"""
# Analyze patterns to predict future cache needs
similar_keys = await self._find_similar_cache_patterns(key_data)
for similar_key in similar_keys:
# Pre-warm cache for similar requests
asyncio.create_task(self._precompute_similar_request(similar_key))
def get_cache_stats(self) -> Dict:
"""Get cache performance statistics"""
if not self.hit_rate_window:
return {'hit_rate': 0.0, 'total_requests': 0}
hit_rate = sum(self.hit_rate_window) / len(self.hit_rate_window)
return {
'hit_rate': hit_rate,
'total_requests': len(self.hit_rate_window),
'cache_hits': sum(self.hit_rate_window),
'cache_misses': len(self.hit_rate_window) - sum(self.hit_rate_window)
}
Conclusion and Future Directions
Building agentic AI systems for cloud cost management represents a significant evolution in how organizations approach cost optimization. By leveraging open-source models through platforms like Ollama, combined with cloud-based AI services from Anthropic and Mistral, organizations can create sophisticated, autonomous systems that continuously optimize cloud spending while maintaining performance and compliance requirements.
The key to success lies in creating a robust, scalable architecture that can adapt to changing requirements and learn from experience. The multi-agent approach allows for specialization and coordination, while the use of both local and cloud-based models provides flexibility in balancing cost, privacy, and capability requirements.
Future Enhancements
Advanced AI Capabilities:
- Integration of multimodal AI for processing infrastructure diagrams, dashboards, and documentation
- Implementation of federated learning for cross-client insights while maintaining privacy
- Development of domain-specific fine-tuned models for cloud cost optimization
Enhanced Automation:
- Autonomous contract negotiation with cloud providers
- Predictive scaling based on business events and seasonal patterns
- Integration with business intelligence systems for holistic cost optimization
Improved Decision Making:
- Causal inference models to understand the true impact of optimization actions
- Game-theoretic approaches for multi-cloud optimization
- Integration of sustainability metrics alongside cost optimization
The future of cloud cost management lies in intelligent, autonomous systems that can understand business context, predict future needs, and take proactive actions to optimize costs while ensuring performance and compliance. By implementing the architecture and techniques outlined in this guide, organizations can build powerful agentic AI systems that transform cloud cost management from a reactive discipline to a proactive, strategic advantage.
As the field continues to evolve, staying current with advances in AI models, cloud technologies, and optimization techniques will be crucial for maintaining competitive advantage in the rapidly changing landscape of cloud computing and artificial intelligence.
Sentiment Analysis with RNN End to End Project: A Technical Exploration
In today’s digital landscape, understanding sentiment from text data has become a crucial component for businesses and researchers alike. This blog post explores an end-to-end implementation of a sentiment analysis system using Recurrent Neural Networks (RNNs), with a detailed examination of the underlying code, architecture decisions, and deployment strategy.
Try the Sentiment WebApp: model Accuracy > 90%
IMDB Sentiment Analysis Webapp
Analyze the sentiment of any IMDB review using our Sentiment Analysis Tool
Launch ApplicationIntroduction to the Project
The Sentiment Analysis RNN project by Tejas K provides a comprehensive implementation of sentiment analysis that takes raw text as input and classifies it into positive, negative, or neutral categories. What makes this project stand out is its careful attention to the entire machine learning pipeline from data preprocessing to deployment.
Let’s delve into the technical aspects of this implementation.
Data Preprocessing: The Foundation
The quality of any NLP model heavily depends on how well the text data is preprocessed. The project implements several crucial preprocessing steps:
def preprocess_text(text):
# Convert to lowercase
text = text.lower()
# Remove HTML tags
text = re.sub(r'<.*?>', '', text)
# Remove special characters and numbers
text = re.sub(r'[^a-zA-Z\s]', '', text)
# Tokenize
tokens = word_tokenize(text)
# Remove stopwords
stop_words = set(stopwords.words('english'))
tokens = [word for word in tokens if word not in stop_words]
# Lemmatization
lemmatizer = WordNetLemmatizer()
tokens = [lemmatizer.lemmatize(word) for word in tokens]
return ' '.join(tokens)
This preprocessing function performs several important operations:
- Converting text to lowercase to ensure consistent processing
- Removing HTML tags that might be present in web-scraped data
- Filtering out special characters and numbers to focus on alphabetic content
- Tokenizing the text into individual words
- Removing stopwords (common words like “the”, “and”, etc.) that typically don’t carry sentiment
- Lemmatizing words to reduce them to their base form
Building the Vocabulary: Tokenization and Embedding
Before feeding text to an RNN, we need to convert words into numerical vectors. The project implements a vocabulary builder and embedding mechanism:
class Vocabulary:
def __init__(self, max_size=None):
self.word2idx = {"<PAD>": 0, "<UNK>": 1}
self.idx2word = {0: "<PAD>", 1: "<UNK>"}
self.word_count = {}
self.max_size = max_size
def add_word(self, word):
if word not in self.word_count:
self.word_count[word] = 1
else:
self.word_count[word] += 1
def build_vocab(self):
# Sort words by frequency
sorted_words = sorted(self.word_count.items(), key=lambda x: x[1], reverse=True)
# Take only max_size most common words if specified
if self.max_size:
sorted_words = sorted_words[:self.max_size-2] # -2 for <PAD> and <UNK>
# Add words to dictionaries
for word, _ in sorted_words:
idx = len(self.word2idx)
self.word2idx[word] = idx
self.idx2word[idx] = word
def text_to_indices(self, text, max_length=None):
words = text.split()
indices = [self.word2idx.get(word, self.word2idx["<UNK>"]) for word in words]
if max_length:
if len(indices) > max_length:
indices = indices[:max_length]
else:
indices += [self.word2idx["<PAD>"]] * (max_length - len(indices))
return indices
This vocabulary class:
- Maintains mappings between words and their numerical indices
- Counts word frequencies to build a vocabulary of the most common words
- Handles unknown words with a special
<UNK>
token - Pads sequences to a consistent length with a
<PAD>
token - Converts text to sequences of indices for model processing
The Core: RNN Model Architecture
The heart of the project is the RNN model architecture. The implementation uses PyTorch to build a flexible model that can be configured with different RNN cell types (LSTM or GRU) and embedding dimensions:
class SentimentRNN(nn.Module):
def __init__(self, vocab_size, embedding_dim, hidden_dim, output_dim, n_layers,
bidirectional, dropout, pad_idx, cell_type='lstm'):
super().__init__()
self.embedding = nn.Embedding(vocab_size, embedding_dim, padding_idx=pad_idx)
if cell_type.lower() == 'lstm':
self.rnn = nn.LSTM(embedding_dim,
hidden_dim,
num_layers=n_layers,
bidirectional=bidirectional,
dropout=dropout if n_layers > 1 else 0,
batch_first=True)
elif cell_type.lower() == 'gru':
self.rnn = nn.GRU(embedding_dim,
hidden_dim,
num_layers=n_layers,
bidirectional=bidirectional,
dropout=dropout if n_layers > 1 else 0,
batch_first=True)
else:
raise ValueError("cell_type must be 'lstm' or 'gru'")
self.fc = nn.Linear(hidden_dim * 2 if bidirectional else hidden_dim, output_dim)
self.dropout = nn.Dropout(dropout)
def forward(self, text, text_lengths):
# text = [batch size, seq length]
embedded = self.dropout(self.embedding(text))
# embedded = [batch size, seq length, embedding dim]
# Pack sequence for RNN efficiency
packed_embedded = nn.utils.rnn.pack_padded_sequence(embedded, text_lengths.cpu(),
batch_first=True, enforce_sorted=False)
if isinstance(self.rnn, nn.LSTM):
packed_output, (hidden, _) = self.rnn(packed_embedded)
else: # GRU
packed_output, hidden = self.rnn(packed_embedded)
# hidden = [n layers * n directions, batch size, hidden dim]
# If bidirectional, concatenate the final forward and backward hidden states
if self.rnn.bidirectional:
hidden = self.dropout(torch.cat((hidden[-2,:,:], hidden[-1,:,:]), dim=1))
else:
hidden = self.dropout(hidden[-1,:,:])
# hidden = [batch size, hidden dim * n directions]
return self.fc(hidden)
This model includes several key components:
- An embedding layer that converts word indices to dense vectors
- A configurable RNN layer (either LSTM or GRU) that processes the sequence
- Support for bidirectional processing to capture context from both directions
- Dropout for regularization to prevent overfitting
- A final fully connected layer for classification
- Efficient sequence packing to handle variable-length inputs
Training the Model: The Learning Process
The training loop implements several best practices for deep learning:
def train_model(model, train_iterator, optimizer, criterion):
model.train()
epoch_loss = 0
epoch_acc = 0
for batch in train_iterator:
optimizer.zero_grad()
text, text_lengths = batch.text
predictions = model(text, text_lengths)
loss = criterion(predictions, batch.label)
acc = calculate_accuracy(predictions, batch.label)
loss.backward()
nn.utils.clip_grad_norm_(model.parameters(), max_norm=5)
optimizer.step()
epoch_loss += loss.item()
epoch_acc += acc.item()
return epoch_loss / len(train_iterator), epoch_acc / len(train_iterator)
Notable aspects include:
- Setting the model to training mode with
model.train()
- Zeroing gradients before each batch to prevent accumulation
- Computing loss and accuracy for monitoring training progress
- Implementing gradient clipping to prevent exploding gradients
- Updating model weights with the optimizer
- Tracking and returning average loss and accuracy
Evaluation and Testing: Measuring Performance
The evaluation function follows a similar structure but disables certain training-specific components:
def evaluate_model(model, iterator, criterion):
model.eval()
epoch_loss = 0
epoch_acc = 0
with torch.no_grad():
for batch in iterator:
text, text_lengths = batch.text
predictions = model(text, text_lengths)
loss = criterion(predictions, batch.label)
acc = calculate_accuracy(predictions, batch.label)
epoch_loss += loss.item()
epoch_acc += acc.item()
return epoch_loss / len(iterator), epoch_acc / len(iterator)
Key differences from the training function:
- Setting the model to evaluation mode with
model.eval()
- Using
torch.no_grad()
to disable gradient calculation for efficiency - Not performing backward passes or optimizer steps
Model Deployment: From PyTorch to Streamlit
The project’s deployment strategy involves exporting the trained PyTorch model to TorchScript for production use:
def export_model(model, vocab):
model.eval()
# Create a script module from the PyTorch model
example_text = torch.randint(0, len(vocab), (1, 10))
example_lengths = torch.tensor([10])
traced_model = torch.jit.trace(model, (example_text, example_lengths))
# Save the scripted model
torch.jit.save(traced_model, "sentiment_model.pt")
# Save the vocabulary
with open("vocab.json", "w") as f:
json.dump({
"word2idx": vocab.word2idx,
"idx2word": {int(k): v for k, v in vocab.idx2word.items()}
}, f)
The exported model is then integrated into a Streamlit application for easy access:
def load_model():
# Load the TorchScript model
model = torch.jit.load("sentiment_model.pt")
# Load vocabulary
with open("vocab.json", "r") as f:
vocab_data = json.load(f)
# Recreate vocabulary object
vocab = Vocabulary()
vocab.word2idx = vocab_data["word2idx"]
vocab.idx2word = {int(k): v for k, v in vocab_data["idx2word"].items()}
return model, vocab
def predict_sentiment(model, vocab, text):
# Preprocess text
processed_text = preprocess_text(text)
# Convert to indices
indices = vocab.text_to_indices(processed_text, max_length=100)
tensor = torch.LongTensor(indices).unsqueeze(0) # Add batch dimension
length = torch.tensor([len(indices)])
# Make prediction
model.eval()
with torch.no_grad():
prediction = model(tensor, length)
# Get probability using softmax
probabilities = F.softmax(prediction, dim=1)
# Get predicted class
predicted_class = torch.argmax(prediction, dim=1).item()
# Map to sentiment
sentiment_map = {0: "Negative", 1: "Neutral", 2: "Positive"}
return {
"sentiment": sentiment_map[predicted_class],
"confidence": probabilities[0][predicted_class].item(),
"probabilities": {
sentiment_map[i]: prob.item() for i, prob in enumerate(probabilities[0])
}
}
The Streamlit application code brings everything together in a user-friendly interface:
def main():
st.title("Sentiment Analysis with RNN")
model, vocab = load_model()
st.write("Enter text to analyze its sentiment:")
user_input = st.text_area("Text input", "")
if st.button("Analyze Sentiment"):
if user_input:
with st.spinner("Analyzing..."):
result = predict_sentiment(model, vocab, user_input)
st.write(f"**Sentiment:** {result['sentiment']}")
st.write(f"**Confidence:** {result['confidence']*100:.2f}%")
# Display probabilities
st.write("### Probability Distribution")
for sentiment, prob in result['probabilities'].items():
st.write(f"{sentiment}: {prob*100:.2f}%")
st.progress(prob)
else:
st.warning("Please enter some text to analyze.")
if __name__ == "__main__":
main()
The iframe parameters and styling ensure:
- The dark theme specified with
embed_options=dark_theme
- Responsive design that works on different screen sizes
- Clean integration with the WordPress site’s aesthetics
- Proper sizing to accommodate the application’s interface
Performance Optimization and Model Improvements
The project implements several performance optimizations:
- Batch processing during training to improve GPU utilization:
def create_iterators(train_data, valid_data, test_data, batch_size=64):
train_iterator, valid_iterator, test_iterator = data.BucketIterator.splits(
(train_data, valid_data, test_data),
batch_size=batch_size,
sort_key=lambda x: len(x.text),
sort_within_batch=True,
device=device)
return train_iterator, valid_iterator, test_iterator
- Early stopping to prevent overfitting:
def train_with_early_stopping(model, train_iterator, valid_iterator,
optimizer, criterion, patience=5):
best_valid_loss = float('inf')
epochs_without_improvement = 0
for epoch in range(max_epochs):
train_loss, train_acc = train_model(model, train_iterator, optimizer, criterion)
valid_loss, valid_acc = evaluate_model(model, valid_iterator, criterion)
if valid_loss < best_valid_loss:
best_valid_loss = valid_loss
torch.save(model.state_dict(), 'best-model.pt')
epochs_without_improvement = 0
else:
epochs_without_improvement += 1
print(f'Epoch: {epoch+1}')
print(f'\tTrain Loss: {train_loss:.3f} | Train Acc: {train_acc*100:.2f}%')
print(f'\tVal. Loss: {valid_loss:.3f} | Val. Acc: {valid_acc*100:.2f}%')
if epochs_without_improvement >= patience:
print(f'Early stopping after {epoch+1} epochs')
break
# Load the best model
model.load_state_dict(torch.load('best-model.pt'))
return model
- Learning rate scheduling for better convergence:
optimizer = optim.Adam(model.parameters(), lr=2e-4)
scheduler = optim.lr_scheduler.ReduceLROnPlateau(optimizer, mode='min',
factor=0.5, patience=2)
# In training loop
scheduler.step(valid_loss)
Conclusion: Putting It All Together
The Sentiment Analysis RNN project demonstrates how to build a complete NLP system from data preprocessing to web deployment. Key technical takeaways include:
- Effective text preprocessing is crucial for good model performance
- RNNs (particularly LSTMs and GRUs) excel at capturing sequential dependencies in text
- Proper training techniques like early stopping and learning rate scheduling improve model quality
- Model export and deployment bridges the gap between development and production
- Web integration makes the model accessible to end-users without technical knowledge
By embedding the Streamlit application in a WordPress site, this technical solution becomes accessible to a wider audience, showcasing how advanced NLP techniques can be applied to practical problems.
The combination of robust model architecture, efficient training procedures, and user-friendly deployment makes this project an excellent case study in applied deep learning for natural language processing.
You can explore the full implementation on GitHub or try the live demo at Streamlit App.
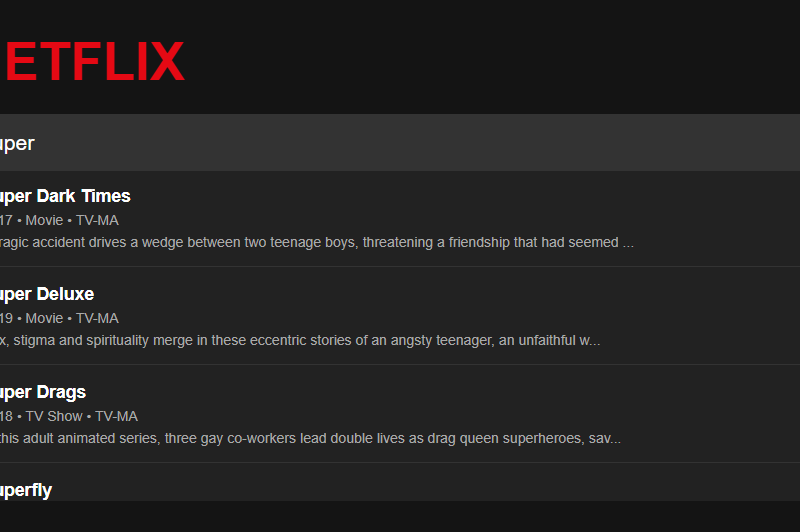
Netflix Autosuggest Search Engine
By Tejas Kamble – AI/ML Developer & Researcher | tejaskamble.com
Introduction
Have you ever used the Netflix search bar and instantly seen suggestions that seem to know exactly what you’re looking for—even before you finish typing? Inspired by this, I created a Netflix Search Engine using NLP Text Suggestions — a project that bridges the power of natural language processing (NLP) with real-time search functionalities.
In this post, I’ll walk you through the codebase hosted on my GitHub: Netflix_Search_Engine_NLP_Text_suggestion, breaking down each important part, from data loading and text preprocessing to building the suggestion logic and deploying it using Flask.
📂 Project Structure
Netflix_Search_Engine_NLP_Text_suggestion/
├── app.py ← Flask Web App
├── netflix_titles.csv ← Dataset of Netflix shows/movies
├── templates/
│ ├── index.html ← Frontend UI
├── static/
│ └── style.css ← Custom styling
├── requirements.txt ← Python dependencies
└── README.md ← Project overview
Dataset Overview
I used a dataset of Netflix titles (from Kaggle). It includes:
- Title: Name of the show/movie
- Description: Synopsis of the content
- Cast: Actors involved
- Genres, Date Added, Duration and more…
This dataset is essential for understanding user intent when making text suggestions.
Step-by-Step Breakdown of the Code
Loading the Dataset
df = pd.read_csv("netflix_titles.csv")
df.dropna(subset=['title'], inplace=True)
We load the dataset and ensure there are no missing values in the title
column since that’s our search anchor.
Text Vectorization using TF-IDF
from sklearn.feature_extraction.text import TfidfVectorizer
vectorizer = TfidfVectorizer(stop_words='english')
tfidf_matrix = vectorizer.fit_transform(df['title'])
- TF-IDF (Term Frequency-Inverse Document Frequency) is used to convert titles into numerical vectors.
- This helps quantify the importance of each word in the context of the entire dataset.
Cosine Similarity Search
from sklearn.metrics.pairwise import cosine_similarity
def get_recommendations(input_text):
input_vec = vectorizer.transform([input_text])
similarity = cosine_similarity(input_vec, tfidf_matrix)
indices = similarity.argsort()[0][-5:][::-1]
return df['title'].iloc[indices]
Here’s where the magic happens:
- The user input is vectorized.
- We compute cosine similarity with all titles.
- The top 5 most similar titles are returned as recommendations.
Flask Web Application
The search engine is hosted using a lightweight Flask backend.
@app.route("/", methods=["GET", "POST"])
def index():
if request.method == "POST":
user_input = request.form["title"]
suggestions = get_recommendations(user_input)
return render_template("index.html", suggestions=suggestions, query=user_input)
return render_template("index.html")
- Accepts user input from the HTML form
- Processes it through
get_recommendations()
- Displays top matching titles
Frontend – index.html
A simple yet effective UI allows users to interact with the engine.
<form method="POST">
<input type="text" name="title" placeholder="Search for Netflix titles...">
<button type="submit">Search</button>
</form>
If suggestions are found, they’re shown dynamically below the form.
🌐 Deployment
To run this app locally:
git clone https://github.com/tejask0512/Netflix_Search_Engine_NLP_Text_suggestion
cd Netflix_Search_Engine_NLP_Text_suggestion
pip install -r requirements.txt
python app.py
Then open http://127.0.0.1:5000
in your browser!
Key Takeaways
- TF-IDF is powerful for information retrieval tasks.
- Even a simple cosine similarity search can replicate sophisticated autocomplete behavior.
- Flask makes it easy to bring machine learning to the web.
What’s Next?
Here are a few ways I plan to extend this project:
- Use BERT or Sentence Transformers for semantic similarity.
- Add spell correction and synonym support.
- Deploy it on Render, Heroku, or HuggingFace Spaces.
- Add a recommendation engine using genres, cast similarity, or collaborative filtering.
🧑💻 About Me
I’m Tejas Kamble, an AI/ML Developer & Researcher passionate about building intelligent, ethical, and multilingual human-computer interaction systems. I focus on:
- AI-driven trading strategies
- NLP-based behavioral analysis
- Real-time blockchain sentiment analysis
- Deep learning for crop disease detection
Check out more of my work on my GitHub @tejask0512
🌐 Website: tejaskamble.com
💬 Feedback & Collaboration
I’d love to hear your thoughts or collaborate on cool projects!
Let’s connect: tejaskamble.com/contact
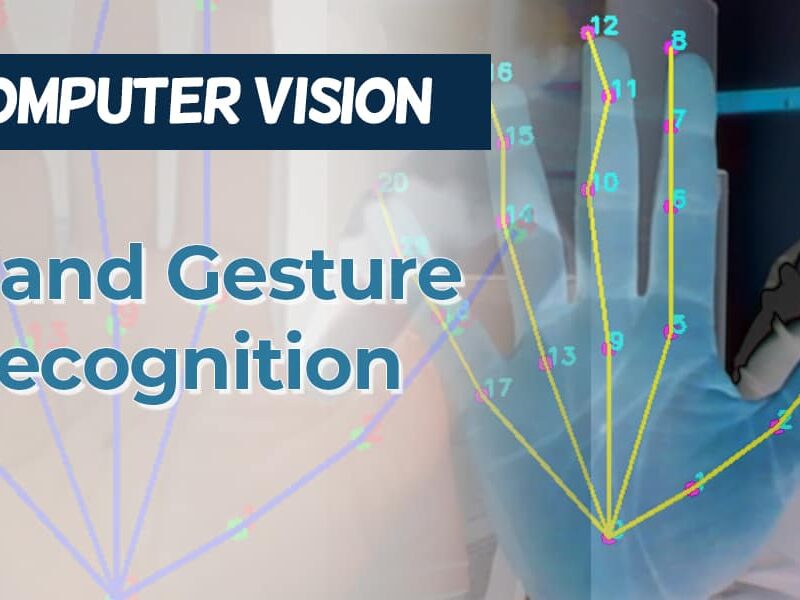
Computer Vision for Gesture Control: Building a Hand-Controlled Mouse
Introduction
In today’s digital era, the way we interact with computers continues to evolve. Beyond the traditional keyboard and mouse, gesture recognition represents one of the most intuitive forms of human-computer interaction. By leveraging computer vision techniques and machine learning, we can create systems that interpret hand movements and translate them into computer commands.
This blog explores the development of a gesture-controlled mouse system that allows users to control their cursor and perform clicks using only hand movements captured by a webcam. We’ll dive deep into the underlying computer vision technologies, implementation details, and practical considerations for building such a system.
The Science Behind Gesture Recognition
Computer Vision Fundamentals
Computer vision is the field that enables computers to derive meaningful information from digital images or videos. At its core, it involves:
- Image Acquisition: Capturing visual data through cameras or sensors
- Image Processing: Manipulating images to enhance features or reduce noise
- Feature Detection: Identifying points of interest within an image
- Pattern Recognition: Classifying patterns or objects within the visual data
For gesture control systems, we need reliable methods to detect hands, identify their landmarks (key points), and interpret their movements.
Hand Detection and Tracking
Modern hand tracking systems typically follow a two-stage approach:
- Hand Detection: Locating the hand within the frame
- Landmark Detection: Identifying specific points on the hand (fingertips, joints, palm center)
Historically, approaches included:
- Color-based segmentation: Isolating hand regions based on skin color
- Background subtraction: Identifying moving objects against a static background
- Feature-based methods: Using handcrafted features like Haar cascades or HOG
Today’s state-of-the-art systems leverage deep learning, specifically convolutional neural networks (CNNs), for both detection and landmark identification.
MediaPipe Hands
Google’s MediaPipe Hands is currently one of the most accessible and accurate hand tracking solutions available. It provides:
- Real-time hand detection
- 21 3D landmarks per hand
- Support for multiple hands
- Cross-platform compatibility
MediaPipe uses a pipeline approach:
- A palm detector that locates hand regions
- A hand landmark model that identifies 21 key points
- A gesture recognition system built on these landmarks
Each landmark corresponds to a specific anatomical feature of the hand:
- Wrist point
- Thumb (4 points)
- Index finger (4 points)
- Middle finger (4 points)
- Ring finger (4 points)
- Pinky finger (4 points)
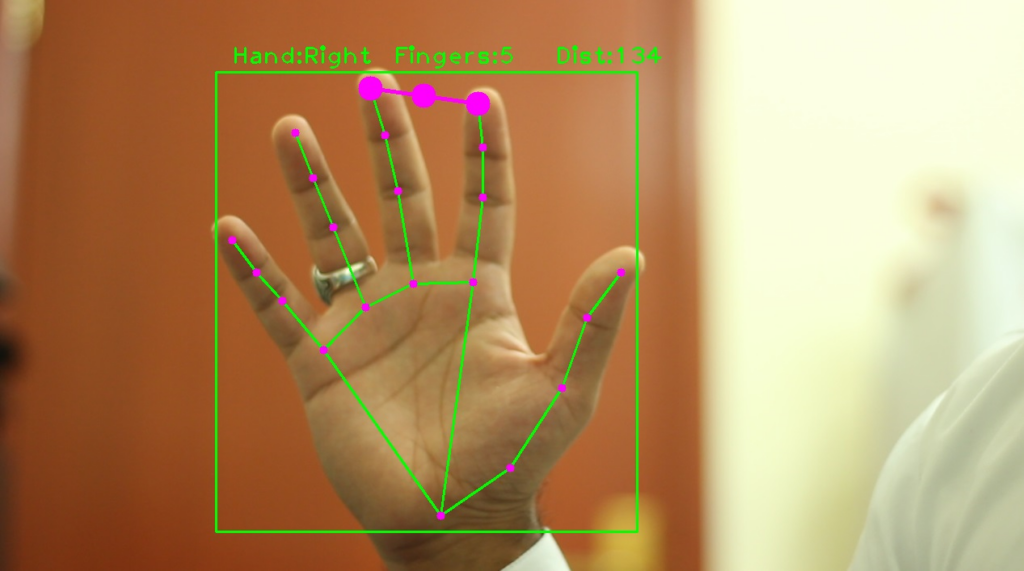
Sample Code
import cvzone
import cv2
cap = cv2.VideoCapture(0)
cap.set(3, 1280)
cap.set(4, 720)
detector = cvzone.HandDetector(detectionCon=0.5, maxHands=1)
while True:
# Get image frame
success, img = cap.read()
# Find the hand and its landmarks
img = detector.findHands(img)
lmList, bbox = detector.findPosition(img)
# Display
cv2.imshow("Image", img)
cv2.waitKey(1)
Building a Gesture-Controlled Mouse
System Architecture
Our gesture mouse system consists of several interconnected components:
- Input Processing: Captures and processes webcam input
- Hand Detection: Identifies hands in the frame
- Landmark Extraction: Locates the 21 key points on each hand
- Gesture Recognition: Interprets specific hand configurations as commands
- Command Execution: Translates gestures into mouse actions
Required Technologies and Libraries
To implement this system, we’ll use:
- OpenCV: For webcam capture and image processing
- MediaPipe: For hand detection and landmark tracking
- PyAutoGUI: For programmatically controlling the mouse
- NumPy: For efficient numerical operations
Implementation Details
Let’s explore the core functionality of our gesture-controlled mouse system:
1. Setting Up the Environment
First, we initialize the necessary libraries and configure MediaPipe for hand tracking:
import cv2
import mediapipe as mp
import pyautogui
import numpy as np
import time
# Initialize MediaPipe Hand solution
mp_hands = mp.solutions.hands
hands = mp_hands.Hands(
static_image_mode=False,
max_num_hands=1,
min_detection_confidence=0.7,
min_tracking_confidence=0.5
)
mp_drawing = mp.solutions.drawing_utils
# Get screen dimensions for mapping hand position to screen coordinates
screen_width, screen_height = pyautogui.size()
The MediaPipe configuration includes several important parameters:
static_image_mode=False
: Optimizes for video sequence trackingmax_num_hands=1
: Limits detection to one hand for simplicitymin_detection_confidence=0.7
: Sets the threshold for hand detectionmin_tracking_confidence=0.5
: Sets the threshold for tracking continuation
2. Capturing and Processing Video
Next, we set up the webcam capture and create the main processing loop:
# Get webcam
cap = cv2.VideoCapture(0)
while cap.isOpened():
success, image = cap.read()
if not success:
print("Failed to capture image from webcam.")
continue
# Flip the image horizontally for a more intuitive mirror view
image = cv2.flip(image, 1)
# Convert BGR image to RGB for MediaPipe
rgb_image = cv2.cvtColor(image, cv2.COLOR_BGR2RGB)
# Process the image and detect hands
results = hands.process(rgb_image)
The horizontal flip creates a mirror-like experience, making the interaction more intuitive for users.
3. Hand Landmark Detection
Once we have processed the image, we extract and visualize the hand landmarks:
# Draw hand landmarks if detected
if results.multi_hand_landmarks:
for hand_landmarks in results.multi_hand_landmarks:
mp_drawing.draw_landmarks(
image, hand_landmarks, mp_hands.HAND_CONNECTIONS)
# Get the landmarks as a list
landmarks = hand_landmarks.landmark
# Process landmarks for mouse control...
Each detected hand provides 21 landmarks with normalized coordinates:
- x, y: Normalized to [0.0, 1.0] within the image
- z: Represents depth with the wrist as origin (negative values are toward the camera)
4. Implementing Mouse Movement
To control mouse movement, we map hand position to screen coordinates:
# Smoothing factors
smoothing = 5
prev_x, prev_y = 0, 0
# Inside the main loop:
# Using wrist position for mouse control
wrist = landmarks[mp_hands.HandLandmark.WRIST]
x = int(wrist.x * screen_width)
y = int(wrist.y * screen_height)
# Apply smoothing for more stable cursor movement
prev_x = prev_x + (x - prev_x) / smoothing
prev_y = prev_y + (y - prev_y) / smoothing
# Move the mouse
pyautogui.moveTo(prev_x, prev_y)
The smoothing factor reduces jitter by creating a weighted average between the current and previous positions, resulting in more fluid cursor movement.
5. Gesture Recognition for Mouse Clicks
For click actions, we detect finger tap gestures:
def detect_finger_tap(landmarks, finger_tip_idx, finger_pip_idx):
"""Detect if a finger is tapped (tip close to palm)"""
tip = landmarks[finger_tip_idx]
pip = landmarks[finger_pip_idx]
# Calculate vertical distance between tip and pip
distance = abs(tip.y - pip.y)
# If tip is below pip and close enough, it's a tap
return tip.y > pip.y and distance < tap_threshold
# In the main loop:
# Detect index finger tap for left click
if detect_finger_tap(landmarks, mp_hands.HandLandmark.INDEX_FINGER_TIP, mp_hands.HandLandmark.INDEX_FINGER_PIP):
current_time = time.time()
if current_time - last_index_tap_time > tap_cooldown:
print("Left click")
pyautogui.click()
last_index_tap_time = current_time
# Detect middle finger tap for right click
if detect_finger_tap(landmarks, mp_hands.HandLandmark.MIDDLE_FINGER_TIP, mp_hands.HandLandmark.MIDDLE_FINGER_PIP):
current_time = time.time()
if current_time - last_middle_tap_time > tap_cooldown:
print("Right click")
pyautogui.rightClick()
last_middle_tap_time = current_time
The tap detection works by:
- Measuring the vertical distance between a fingertip and its corresponding PIP joint
- Identifying a tap when the fingertip moves below the joint and within a certain distance threshold
- Implementing a cooldown period to prevent accidental multiple clicks
Implementing Scrolling Functionality
Scrolling is an essential feature for navigating documents and webpages. Let’s implement smooth scrolling control using hand gestures.
1. Pinch-to-Scroll Implementation
One of the most intuitive ways to implement scrolling is through a pinch gesture between the thumb and ring finger, followed by vertical movement:
# Global variables for tracking scroll state
scroll_active = False
scroll_start_y = 0
last_scroll_time = 0
scroll_cooldown = 0.05 # Seconds between scroll actions
scroll_sensitivity = 1.0 # Adjustable scroll sensitivity
def detect_scroll_gesture(landmarks):
"""Detect thumb and ring finger pinch for scrolling"""
thumb_tip = landmarks[mp_hands.HandLandmark.THUMB_TIP]
ring_tip = landmarks[mp_hands.HandLandmark.RING_FINGER_TIP]
# Calculate distance between thumb and ring finger
distance = np.sqrt((thumb_tip.x - ring_tip.x)**2 + (thumb_tip.y - ring_tip.y)**2)
# If thumb and ring finger are close enough, it's a pinch
return distance < 0.07 # Threshold value may need adjustment
# In the main loop:
if results.multi_hand_landmarks:
landmarks = results.multi_hand_landmarks[0].landmark
# Check for scroll gesture
is_scroll_gesture = detect_scroll_gesture(landmarks)
# Get middle point between thumb and ring finger for tracking
if is_scroll_gesture:
thumb_tip = landmarks[mp_hands.HandLandmark.THUMB_TIP]
ring_tip = landmarks[mp_hands.HandLandmark.RING_FINGER_TIP]
current_y = (thumb_tip.y + ring_tip.y) / 2
# Initialize scroll if just started pinching
if not scroll_active:
scroll_active = True
scroll_start_y = current_y
else:
# Calculate scroll distance
current_time = time.time()
if current_time - last_scroll_time > scroll_cooldown:
# Convert movement to scroll amount
scroll_amount = int((current_y - scroll_start_y) * 20 * scroll_sensitivity)
if abs(scroll_amount) > 0:
# Scroll up or down
pyautogui.scroll(-scroll_amount) # Negative because screen coordinates are inverted
scroll_start_y = current_y # Reset start position
last_scroll_time = current_time
else:
scroll_active = False
This implementation:
- Detects a pinch between the thumb and ring finger
- Tracks the vertical movement of the pinch
- Converts the movement to scrolling actions
- Uses a cooldown mechanism to prevent too many scroll events
- Applies sensitivity settings to adjust scroll speed
2. Alternative: Two-Finger Scroll Gesture
For users who might find the pinch gesture challenging, we can implement an alternative two-finger scroll method:
def detect_two_finger_scroll(landmarks):
"""Detect index and middle finger extended for scrolling"""
index_tip = landmarks[mp_hands.HandLandmark.INDEX_FINGER_TIP]
index_pip = landmarks[mp_hands.HandLandmark.INDEX_FINGER_PIP]
middle_tip = landmarks[mp_hands.HandLandmark.MIDDLE_FINGER_TIP]
middle_pip = landmarks[mp_hands.HandLandmark.MIDDLE_FINGER_PIP]
# Check if both fingers are extended (tips above pips)
index_extended = index_tip.y < index_pip.y
middle_extended = middle_tip.y < middle_pip.y
# Check if other fingers are curled
ring_tip = landmarks[mp_hands.HandLandmark.RING_FINGER_TIP]
ring_pip = landmarks[mp_hands.HandLandmark.RING_FINGER_PIP]
pinky_tip = landmarks[mp_hands.HandLandmark.PINKY_TIP]
pinky_pip = landmarks[mp_hands.HandLandmark.PINKY_PIP]
ring_curled = ring_tip.y > ring_pip.y
pinky_curled = pinky_tip.y > pinky_pip.y
# Return true if index and middle extended, others curled
return index_extended and middle_extended and ring_curled and pinky_curled
This can then be integrated into the main loop similarly to the pinch gesture method.
3. Visual Feedback for Scrolling
Providing visual feedback helps users understand when the system recognizes their scroll gesture:
# Inside the main loop, when scroll gesture is detected:
if is_scroll_gesture:
# Draw a visual indicator for active scrolling
cv2.circle(image, (50, 50), 20, (0, 255, 0), -1) # Green circle when scrolling
cv2.putText(image, f"Scrolling {'UP' if scroll_amount < 0 else 'DOWN'}",
(75, 50), cv2.FONT_HERSHEY_SIMPLEX, 0.7, (0, 255, 0), 2)
Adjustable Mouse Sensitivity
Different users have different preferences for cursor speed and precision. Let’s implement adjustable sensitivity controls:
1. Adding Sensitivity Settings
First, we’ll define sensitivity parameters that can be adjusted:
# Mouse movement sensitivity settings
mouse_sensitivity = 1.0 # Default value
sensitivity_min = 0.2 # Minimum allowed sensitivity
sensitivity_max = 3.0 # Maximum allowed sensitivity
sensitivity_step = 0.1 # Increment/decrement step
2. Applying Sensitivity to Mouse Movement
We need to modify our mouse movement logic to incorporate the sensitivity setting:
# Inside the main loop, when calculating cursor position:
wrist = landmarks[mp_hands.HandLandmark.WRIST]
# Get raw coordinates
raw_x = wrist.x * screen_width
raw_y = wrist.y * screen_height
# Calculate center of screen
center_x = screen_width / 2
center_y = screen_height / 2
# Apply sensitivity to the distance from center
offset_x = (raw_x - center_x) * mouse_sensitivity
offset_y = (raw_y - center_y) * mouse_sensitivity
# Calculate final position
x = int(center_x + offset_x)
y = int(center_y + offset_y)
# Apply smoothing for stable cursor movement
prev_x = prev_x + (x - prev_x) / smoothing
prev_y = prev_y + (y - prev_y) / smoothing
# Move the mouse
pyautogui.moveTo(prev_x, prev_y)
This approach:
- Calculates the cursor position relative to the center of the screen
- Applies the sensitivity factor to the offset from center
- Ensures that low sensitivity gives fine control, while high sensitivity allows rapid movement across the screen
3. Gesture-Based Sensitivity Adjustment
Now we’ll implement gestures to adjust sensitivity on-the-fly:
# Global variables for tracking the last sensitivity adjustment
last_sensitivity_change_time = 0
sensitivity_change_cooldown = 1.0 # Seconds between adjustments
def detect_increase_sensitivity_gesture(landmarks):
"""Detect gesture for increasing sensitivity (pinky and thumb pinch)"""
thumb_tip = landmarks[mp_hands.HandLandmark.THUMB_TIP]
pinky_tip = landmarks[mp_hands.HandLandmark.PINKY_TIP]
distance = np.sqrt((thumb_tip.x - pinky_tip.x)**2 + (thumb_tip.y - pinky_tip.y)**2)
return distance < 0.07
def detect_decrease_sensitivity_gesture(landmarks):
"""Detect gesture for decreasing sensitivity (thumb touching wrist)"""
thumb_tip = landmarks[mp_hands.HandLandmark.THUMB_TIP]
wrist = landmarks[mp_hands.HandLandmark.WRIST]
distance = np.sqrt((thumb_tip.x - wrist.x)**2 + (thumb_tip.y - wrist.y)**2)
return distance < 0.12
# In the main loop:
# Check for sensitivity adjustment gestures
current_time = time.time()
if current_time - last_sensitivity_change_time > sensitivity_change_cooldown:
if detect_increase_sensitivity_gesture(landmarks):
mouse_sensitivity = min(mouse_sensitivity + sensitivity_step, sensitivity_max)
print(f"Sensitivity increased to: {mouse_sensitivity:.1f}")
last_sensitivity_change_time = current_time
elif detect_decrease_sensitivity_gesture(landmarks):
mouse_sensitivity = max(mouse_sensitivity - sensitivity_step, sensitivity_min)
print(f"Sensitivity decreased to: {mouse_sensitivity:.1f}")
last_sensitivity_change_time = current_time
4. On-Screen Sensitivity Display
To help users understand the current sensitivity level, we can display it on the screen:
# Inside the main loop, after handling sensitivity adjustments:
# Display current sensitivity on screen
cv2.putText(image, f"Sensitivity: {mouse_sensitivity:.1f}",
(10, image.shape[0] - 20), cv2.FONT_HERSHEY_SIMPLEX,
0.7, (0, 255, 0), 2)
5. UI Controls for Sensitivity Adjustment
For a more user-friendly experience, we can add GUI controls using OpenCV:
# Create a sensitivity slider using OpenCV
def create_control_window():
cv2.namedWindow('Mouse Controls')
cv2.createTrackbar('Sensitivity', 'Mouse Controls',
int(mouse_sensitivity * 10),
int(sensitivity_max * 10),
on_sensitivity_change)
cv2.createTrackbar('Scroll Speed', 'Mouse Controls',
int(scroll_sensitivity * 10),
int(sensitivity_max * 10),
on_scroll_sensitivity_change)
def on_sensitivity_change(value):
global mouse_sensitivity
mouse_sensitivity = value / 10.0
def on_scroll_sensitivity_change(value):
global scroll_sensitivity
scroll_sensitivity = value / 10.0
# Call at the beginning of your program
create_control_window()
6. Configuration File for Persistent Settings
To remember user preferences between sessions, we can save settings to a configuration file:
import json
import os
config_file = "gesture_mouse_config.json"
def save_settings():
"""Save current settings to a JSON file"""
settings = {
"mouse_sensitivity": mouse_sensitivity,
"scroll_sensitivity": scroll_sensitivity,
"smoothing": smoothing
}
with open(config_file, 'w') as f:
json.dump(settings, f)
print("Settings saved!")
def load_settings():
"""Load settings from a JSON file if it exists"""
global mouse_sensitivity, scroll_sensitivity, smoothing
if os.path.exists(config_file):
try:
with open(config_file, 'r') as f:
settings = json.load(f)
mouse_sensitivity = settings.get("mouse_sensitivity", mouse_sensitivity)
scroll_sensitivity = settings.get("scroll_sensitivity", scroll_sensitivity)
smoothing = settings.get("smoothing", smoothing)
print("Settings loaded!")
except:
print("Error loading settings. Using defaults.")
# Load settings at startup
load_settings()
# Add keyboard event to save settings:
# (inside the main loop)
key = cv2.waitKey(1) & 0xFF
if key == ord('s'):
save_settings()
Technical Challenges and Solutions
Challenge 1: Hand Detection Stability
Problem: Hand detection can be inconsistent under varying lighting conditions or when the hand moves quickly.
Solution: Multiple approaches can improve stability:
- Adjust MediaPipe confidence thresholds based on your environment
- Implement background removal techniques to isolate the hand
- Use temporal filtering to reject spurious detections
Challenge 2: Gesture Recognition Accuracy
Problem: Distinguishing intentional gestures from natural hand movements.
Solution:
- Define clear gesture thresholds
- Implement gesture “holding” requirements (e.g., maintain a gesture for 300ms)
- Add visual feedback to help users understand when gestures are recognized
Challenge 3: Cursor Stability
Problem: Direct mapping of hand position to cursor coordinates can result in jittery movement.
Solution:
- Implement motion smoothing algorithms (like our weighted average approach)
- Use Kalman filtering for more sophisticated motion prediction
- Create a “deadzone” where small hand movements don’t affect the cursor
Challenge 4: Fatigue and Ergonomics
Problem: Holding the hand in mid-air causes user fatigue over time.
Solution:
- Implement a “clutch” mechanism that enables/disables control
- Design gestures that allow for natural hand positions
- Consider relative positioning rather than absolute positioning
Challenge 5: Scroll Precision
Problem: Scrolling can be too sensitive or jerky with direct hand movement mapping.
Solution:
- Implement non-linear scroll response curves
- Add “scroll momentum” for smoother continuous scrolling
- Provide visual feedback about scroll speed and direction
# Non-linear scroll response curve
def apply_scroll_curve(movement):
"""Apply a non-linear curve to make small movements more precise"""
# Square the movement but keep the sign
sign = 1 if movement >= 0 else -1
magnitude = abs(movement)
# Apply curve: square for values > 0.1, linear for smaller values
if magnitude > 0.1:
result = sign * ((magnitude - 0.1) ** 2) * 2 + (sign * 0.1)
else:
result = sign * magnitude
return result
Advanced Features and Improvements
Enhancing Mouse Movement
For more precise control, we can improve the mapping between hand position and cursor movement:
# Define a region of interest in the camera's field of view
roi_left = 0.2
roi_right = 0.8
roi_top = 0.2
roi_bottom = 0.8
# Map the hand position within this region to screen coordinates
def map_to_screen(x, y):
screen_x = screen_width * (x - roi_left) / (roi_right - roi_left)
screen_y = screen_height * (y - roi_top) / (roi_bottom - roi_top)
return max(0, min(screen_width, screen_x)), max(0, min(screen_height, screen_y))
This approach creates a smaller “active area” within the camera’s view, allowing for more precise movements.
Implementing Additional Gestures
Beyond basic clicking, we can add more complex interactions:
- Scroll wheel emulation:
def detect_scroll_gesture(landmarks):
thumb_tip = landmarks[mp_hands.HandLandmark.THUMB_TIP]
index_tip = landmarks[mp_hands.HandLandmark.INDEX_FINGER_TIP]
# Calculate pinch distance
distance = ((thumb_tip.x - index_tip.x)**2 + (thumb_tip.y - index_tip.y)**2)**0.5
# If pinching, track vertical movement for scrolling
if distance < pinch_threshold:
return (index_tip.y - prev_index_y) * scroll_sensitivity
return 0
- Drag and drop:
# Track index finger extension status
index_extended = landmarks[mp_hands.HandLandmark.INDEX_FINGER_TIP].y < landmarks[mp_hands.HandLandmark.INDEX_FINGER_PIP].y
# If status changes from extended to not extended while moving, start drag
if prev_index_extended and not index_extended:
pyautogui.mouseDown()
elif not prev_index_extended and index_extended:
pyautogui.mouseUp()
- Gesture-based shortcuts:
# Detect specific finger configurations
if all_fingers_extended(landmarks):
# Perform action, like opening task manager
pyautogui.hotkey('ctrl', 'shift', 'esc')
Calibration System
A calibration system improves accuracy across different users and environments:
def calibrate():
calibration_points = [(0.1, 0.1), (0.9, 0.1), (0.9, 0.9), (0.1, 0.9)]
user_points = []
for point in calibration_points:
# Prompt user to place hand at this position
# Record actual hand position
user_points.append((wrist.x, wrist.y))
# Create transformation matrix
transformation = calculate_transformation(calibration_points, user_points)
return transformation
Performance Optimization
To ensure smooth operation, several optimizations are critical:
1. Frame Rate Management
Processing every frame can be computationally expensive. We can reduce the processing load:
# Process only every n frames
if frame_count % process_every_n_frames == 0:
# Process hand detection and tracking
else:
# Use the previous result
2. Resolution Scaling
Lower resolution processing can significantly improve performance:
# Scale down the image for processing
process_scale = 0.5
small_frame = cv2.resize(image, (0, 0), fx=process_scale, fy=process_scale)
# Process the smaller image
results = hands.process(small_frame)
# Scale coordinates back up when using them
x = int(landmark.x / process_scale)
y = int(landmark.y / process_scale)
3. Multi-threading
Separating video capture from processing improves responsiveness:
def capture_thread():
while running:
ret, frame = cap.read()
if ret:
frame_queue.put(frame)
def process_thread():
while running:
if not frame_queue.empty():
frame = frame_queue.get()
# Process the frame
Real-World Applications
Gesture control systems have numerous practical applications beyond cursor control:
- Accessibility: Enables computer use for people with mobility impairments
- Medical Environments: Allows for touchless interaction in sterile settings
- Presentations: Facilitates natural interaction with slides and content
- Gaming: Creates immersive control experiences without specialized hardware
- Smart Home Control: Enables intuitive interaction with IoT devices
- Virtual Reality: Provides hand tracking for more realistic VR experiences
Challenges and Future Directions
While powerful, gesture control systems face several ongoing challenges:
Technical Limitations
- Occlusion: Fingers may be hidden from the camera’s view
- Background Complexity: Busy environments can confuse hand detection
- Lighting Sensitivity: Performance varies with lighting conditions
- Camera Limitations: Low frame rates or resolution affect tracking quality
Future Research Directions
- Multi-modal Integration: Combining gestures with voice commands or eye tracking
- Context-aware Gestures: Adapting to different applications automatically
- Personalized Gestures: Learning user-specific gesture patterns
- Transfer Learning: Applying knowledge from one gesture domain to another
- Edge Processing: Moving computations to specialized hardware for better performance
Conclusion
Computer vision-based gesture control represents a significant step forward in human-computer interaction, offering a more natural and intuitive way to control computers. By leveraging libraries like MediaPipe and OpenCV, developers can now create sophisticated gesture recognition systems with relatively modest technical requirements.
Our gesture-controlled mouse system demonstrates the core principles of this technology, with additional features like scrolling and adjustable sensitivity making it truly practical for everyday use. The accessibility and customizability of such systems highlight the exciting possibilities at the intersection of computer vision, machine learning, and human-computer interaction.
Whether for accessibility, specialized environments, or simply for the joy of a more natural interaction, gesture control systems are poised to become an increasingly common part of our digital interfaces.
Code Repository
The complete implementation of the gesture-controlled mouse system described in this blog is available on GitHub at {https://github.com/tejask0512/Hand_Gesture_Mouse_Computer_Vision} . The code is extensively commented to help you understand each component and customize it for your specific needs.
References and Further Reading
- MediaPipe Hands: https://google.github.io/mediapipe/solutions/hands.html
- OpenCV Documentation: https://docs.opencv.org/
- PyAutoGUI Documentation: https://pyautogui.readthedocs.io/
- “Hand Gesture Recognition: A Literature Review” – S. S. Rautaray and A. Agrawal
- “Vision Based Hand Gesture Recognition for Human Computer Interaction” – Pavlovic et al.
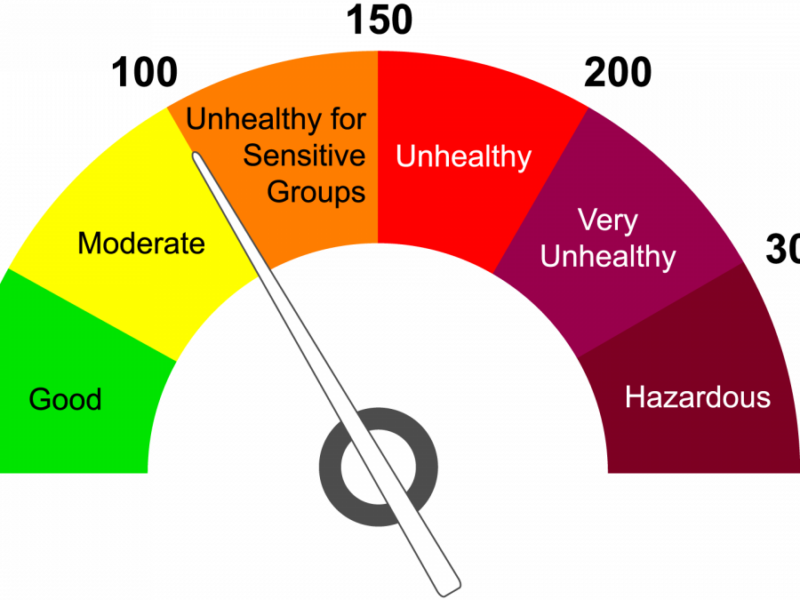
Mapping Air Quality Index: A Deep Dive into the AQI Google Maps Project
In an era where environmental concerns increasingly shape public policy and personal health decisions, access to real-time air quality data has never been more crucial. The AQI Google Maps project represents an innovative approach to environmental monitoring, combining Google Maps’ familiar interface with critical air quality metrics. This open-source initiative transforms complex environmental data into an accessible visualization tool that can benefit researchers, policymakers, and everyday citizens concerned about the air they breathe.
What is the AQI Google Maps Project?
The AQI (Air Quality Index) Google Maps project is an open-source web application that integrates air quality data with Google Maps to provide a visual representation of air pollution levels across different locations. Developed by Tejas K (GitHub: tejask0512), this project leverages modern web technologies and public APIs to create an interactive map where users can view air quality conditions with intuitive color-coded markers.
Technical Architecture
The project employs a straightforward yet effective technical stack:
- Frontend: HTML, CSS, JavaScript
- APIs: Google Maps API for mapping functionality, Air Quality APIs for pollution data
- Data Visualization: Custom markers and color-coding system
The core functionality revolves around fetching air quality data based on geographic coordinates and rendering this information as color-coded markers on the Google Maps interface. The colors transition from green (good air quality) through yellow and orange to red and purple (hazardous air quality), providing an immediate visual understanding of conditions in different areas.
Deep Dive into AQI Analysis
Understanding the Air Quality Index
The Air Quality Index is a standardized indicator developed by environmental agencies to communicate how polluted the air is and what associated health effects might be. The AQI Google Maps project implements this complex calculation system and presents it in an accessible format.
The AQI typically accounts for multiple pollutants:
Pollutant | Source | Health Impact |
---|---|---|
PM2.5 (Fine Particulate Matter) | Combustion engines, forest fires, industrial processes | Can penetrate deep into lungs and bloodstream |
PM10 (Coarse Particulate Matter) | Dust, pollen, mold | Respiratory irritation, asthma exacerbation |
O3 (Ozone) | Created by chemical reactions between NOx and VOCs | Lung damage, respiratory issues |
NO2 (Nitrogen Dioxide) | Vehicles, power plants | Respiratory inflammation |
SO2 (Sulfur Dioxide) | Fossil fuel combustion, industrial processes | Respiratory issues, contributes to acid rain |
CO (Carbon Monoxide) | Incomplete combustion | Reduces oxygen delivery in bloodstream |
The project likely calculates an overall AQI based on the highest concentration of any single pollutant, following the EPA’s approach where:
- 0-50 (Green): Good air quality with minimal health concerns
- 51-100 (Yellow): Moderate air quality; unusually sensitive individuals may experience issues
- 101-150 (Orange): Unhealthy for sensitive groups
- 151-200 (Red): Unhealthy for all groups
- 201-300 (Purple): Very unhealthy; may trigger health alerts
- 301+ (Maroon): Hazardous; serious health effects for entire population
The technical implementation likely includes conversion formulas to normalize different pollutant measurements to the same 0-500 AQI scale.
Real-time Data Processing
A key technical achievement of the project is its ability to process real-time air quality data. This involves:
- API Integration: Connecting to air quality data providers through RESTful APIs
- Data Parsing: Extracting relevant metrics from JSON/XML responses
- Coordinate Mapping: Associating pollution data with precise geographic coordinates
- Temporal Synchronization: Managing data freshness and update frequencies
The project handles these operations seamlessly in the background, presenting users with up-to-date information without exposing the complexity of the underlying data acquisition process.
Report Generation Capabilities
One of the project’s valuable features is its ability to generate comprehensive air quality reports. These reports serve multiple purposes:
Types of Reports Generated
- Location-specific Snapshots: Detailed breakdowns of current air quality at selected points
- Comparative Analysis: Contrasting air quality across multiple locations
- Temporal Reports: Tracking air quality changes over time (hourly, daily, weekly)
- Pollutant-specific Reports: Focusing on individual contaminants like PM2.5 or O3
Report Components
The reporting system likely includes:
- Statistical Summaries: Min/max/mean values for AQI metrics
- Health Impact Assessments: Explanations of potential health effects based on current readings
- Visualizations: Charts and graphs depicting pollution trends
- Contextual Information: Weather conditions that may influence readings
- Actionable Recommendations: Suggested activities based on air quality levels
Technical Implementation of Reporting
From a development perspective, the reporting functionality demonstrates sophisticated data processing:
// Conceptual example of report generation logic
function generateAQIReport(locationData, timeframe) {
const reportData = {
location: locationData.name,
coordinates: locationData.coordinates,
timestamp: new Date(),
metrics: {
overall: calculateOverallAQI(locationData.pollutants),
individual: locationData.pollutants,
trends: analyzeTrends(locationData.history, timeframe)
},
healthImplications: assessHealthImpact(calculateOverallAQI(locationData.pollutants)),
recommendations: generateRecommendations(calculateOverallAQI(locationData.pollutants))
};
return formatReport(reportData, preferredFormat);
}
This functionality transforms raw data into actionable intelligence, making the project valuable beyond simple visualization.
AQI and Location Coordinate Data for Machine Learning
Perhaps the most forward-looking aspect of the project is its potential for generating valuable datasets for machine learning applications. The combination of precise geolocation data with corresponding air quality metrics creates numerous possibilities for advanced environmental analysis.
Data Generation for ML Models
The project effectively creates a continuous stream of structured data points with these key attributes:
- Geographic Coordinates: Latitude and longitude
- Temporal Information: Timestamps for each measurement
- Multiple Pollutant Metrics: PM2.5, PM10, O3, NO2, SO2, CO values
- Calculated AQI: Overall air quality index
- Contextual Metadata: Potentially including weather conditions, urban density, etc.
This multi-dimensional dataset serves as excellent training data for various machine learning models.
Potential ML Applications
With sufficient data collection over time, the following machine learning approaches become possible:
1. Predictive Modeling
Machine learning algorithms can be trained to forecast air quality based on historical patterns:
- Time Series Forecasting: Using techniques like ARIMA, LSTM networks, or Prophet to predict AQI values hours or days in advance
- Multivariate Prediction: Incorporating weather forecasts, traffic patterns, and seasonal factors to improve accuracy
- Anomaly Detection: Identifying unusual pollution events that deviate from expected patterns
# Conceptual example of LSTM model for AQI prediction
from keras.models import Sequential
from keras.layers import LSTM, Dense
def build_aqi_prediction_model(lookback_window):
model = Sequential()
model.add(LSTM(50, activation='relu', input_shape=(lookback_window, n_features)))
model.add(Dense(1))
model.compile(optimizer='adam', loss='mse')
return model
# Train with historical AQI data from project
model = build_aqi_prediction_model(24) # 24-hour lookback window
model.fit(X_train, y_train, epochs=100, validation_split=0.2)
2. Spatial Analysis and Interpolation
The geospatial nature of the data enables sophisticated spatial modeling:
- Kriging/Gaussian Process Regression: Estimating pollution levels between measurement points
- Spatial Autocorrelation: Analyzing how pollution levels at one location influence nearby areas
- Hotspot Identification: Using clustering algorithms to detect persistent pollution sources
# Conceptual example of spatial interpolation
from sklearn.gaussian_process import GaussianProcessRegressor
from sklearn.gaussian_process.kernels import RBF, WhiteKernel
def interpolate_aqi_surface(known_points, known_values, grid_points):
# Define kernel - distance matters for pollution spread
kernel = RBF(length_scale=1.0) + WhiteKernel(noise_level=0.1)
gpr = GaussianProcessRegressor(kernel=kernel)
# Train on known AQI points
gpr.fit(known_points, known_values)
# Predict AQI at all grid points
predicted_values = gpr.predict(grid_points)
return predicted_values
3. Causal Analysis
Advanced machine learning techniques can help identify pollution drivers:
- Causal Inference Models: Determining the impact of traffic changes, industrial activities, or policy interventions on air quality
- Counterfactual Analysis: Estimating what air quality would be under different conditions
- Attribution Modeling: Quantifying the contribution of different sources to overall pollution levels
4. Computer Vision Integration
The project’s map-based approach opens possibilities for combining with visual data:
- Satellite Imagery Analysis: Correlating visible pollution (smog, industrial activity) with measured AQI
- Traffic Density Estimation: Using traffic camera feeds to predict localized pollution spikes
- Urban Development Impact: Analyzing how changes in urban landscapes affect air quality patterns
Implementation Considerations for ML Integration
To fully realize the machine learning potential, the project could implement:
- Data Export APIs: Programmatic access to historical AQI and coordinate data
- Standardized Dataset Generation: Creating properly formatted, cleaned datasets ready for ML models
- Feature Engineering Utilities: Tools to extract temporal patterns, spatial relationships, and other derived features
- Model Integration Endpoints: APIs that allow trained models to feed predictions back into the visualization system
// Conceptual implementation of data export for ML
function exportTrainingData(startDate, endDate, region, format='csv') {
const dataPoints = fetchHistoricalData(startDate, endDate, region);
// Process for ML readiness
const mlReadyData = dataPoints.map(point => ({
timestamp: point.timestamp,
lat: point.coordinates.lat,
lng: point.coordinates.lng,
pm25: point.pollutants.pm25,
pm10: point.pollutants.pm10,
o3: point.pollutants.o3,
no2: point.pollutants.no2,
so2: point.pollutants.so2,
co: point.pollutants.co,
aqi: point.aqi,
// Derived features
hour_of_day: new Date(point.timestamp).getHours(),
day_of_week: new Date(point.timestamp).getDay(),
is_weekend: [0, 6].includes(new Date(point.timestamp).getDay()),
season: calculateSeason(point.timestamp)
}));
return formatDataForExport(mlReadyData, format);
}
Key Features and Capabilities
The project demonstrates several notable features:
- Real-time air quality visualization: Displays current AQI values at selected locations
- Interactive map interface: Users can navigate, zoom, and click on markers to view detailed information
- Color-coded AQI indicators: Intuitive visual representation of pollution levels
- Customizable markers: Location-specific information about air quality conditions
- Responsive design: Functions across various device types and screen sizes
Environmental and Health Significance
The importance of this project extends far beyond its technical implementation. Here’s why such tools matter:
Public Health Impact
Air pollution is directly linked to numerous health problems, including respiratory diseases, cardiovascular issues, and even neurological disorders. According to the World Health Organization, air pollution causes approximately 7 million premature deaths annually worldwide. By making air quality data more accessible, this project empowers individuals to:
- Make informed decisions about outdoor activities
- Understand when to take protective measures (like wearing masks or staying indoors)
- Recognize patterns in local air quality that might affect their health
Environmental Awareness
Environmental literacy begins with awareness. When people can visually connect with environmental data, they’re more likely to:
- Understand the scope and severity of air pollution issues
- Recognize temporal and spatial patterns in air quality
- Connect human activities with environmental outcomes
- Support policies aimed at improving air quality
Research and Policy Applications
For researchers and policymakers, visualized air quality data offers valuable insights:
- Identifying pollution hotspots that require intervention
- Evaluating the effectiveness of environmental regulations
- Planning urban development with air quality considerations
- Allocating resources for environmental monitoring and mitigation
Case Study: Urban Planning and Environmental Justice
The AQI Google Maps project provides a powerful tool for addressing environmental justice concerns. By visualizing pollution patterns across different neighborhoods, it can reveal disparities in air quality that often correlate with socioeconomic factors.
Data-Driven Environmental Justice
Researchers can use the generated datasets to:
- Identify Disproportionate Impacts: Quantify differences in air quality across neighborhoods with varying income levels or racial demographics
- Temporal Justice Analysis: Determine if certain communities bear the burden of poor air quality during specific times (e.g., industrial activity hours)
- Policy Effectiveness: Measure how environmental regulations impact different communities
Practical Application Example
Consider a city planning department using the AQI Google Maps project to assess the impact of a proposed industrial development:
- Establish baseline air quality readings across all affected neighborhoods
- Use predictive modeling (with the ML techniques described above) to estimate pollution changes
- Generate reports showing projected AQI impacts on different communities
- Adjust development plans to minimize disproportionate impacts on vulnerable populations
This data-driven approach promotes equitable development and environmental protection.
The Future of Environmental Data Integration
The AQI Google Maps project represents an important step toward more integrated environmental monitoring. Future development could include:
Data Fusion Opportunities
- Cross-Pollutant Analysis: Investigating relationships between different pollutants
- Multi-Environmental Factor Integration: Combining air quality with noise pollution, water quality, and urban heat island effects
- Health Data Correlation: Connecting real-time AQI with emergency room visits for respiratory issues
Technical Evolution
- Edge Computing Integration: Processing air quality data from low-cost sensors at the edge
- Blockchain for Data Integrity: Ensuring the provenance and authenticity of environmental measurements
- Federated Learning: Enabling distributed model training across multiple air quality monitoring networks
Conclusion
The AQI Google Maps project represents an important intersection of environmental monitoring, data visualization, and public information. Its ability to generate structured air quality data associated with precise geographic coordinates creates a foundation for sophisticated analysis and machine learning applications.
By democratizing access to environmental data and creating opportunities for advanced computational analysis, this project contributes to both public awareness and scientific advancement. The potential for machine learning integration further elevates its significance, enabling predictive capabilities and deeper insights into pollution patterns.
As we continue to face environmental challenges, projects like this demonstrate how technology can be leveraged not just for convenience or entertainment, but for creating a more informed and environmentally conscious society. The combination of visual accessibility with data generation for machine learning represents a powerful approach to environmental monitoring that can drive both individual awareness and systemic change.
This blog post analyzes the AQI Google Maps project developed by Tejas K. The project is open-source and available for contributions on GitHub.
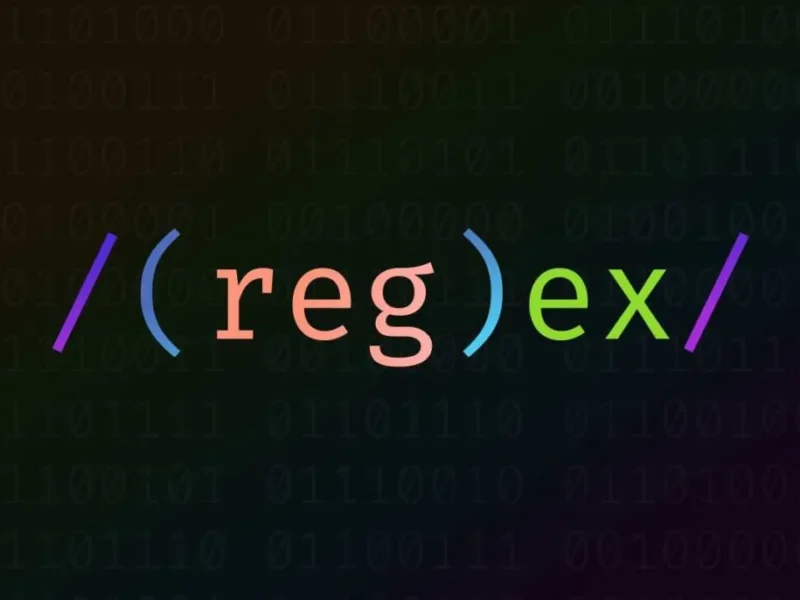
RegEx Mastery: Unlocking Structured Data From Unstructured Text
A comprehensive guide to advanced regular expressions for data mining and extraction
Introduction
In today’s data-driven world, the ability to efficiently extract structured information from unstructured text is invaluable. While many sophisticated NLP and machine learning tools exist for this purpose, regular expressions (regex) remain one of the most powerful and flexible tools in a data scientist’s toolkit. This blog explores advanced regex techniques implemented in the “Advance-Regex-For-Data-Mining-Extraction” project by Tejas K., demonstrating how carefully crafted patterns can transform raw text into actionable insights.
What Makes Regex Essential for Text Mining?
Regular expressions provide a concise, pattern-based approach to text processing that is:
- Language-agnostic: Works across programming languages and text processing tools
- Highly efficient: Once optimized, regex patterns can process large volumes of text quickly
- Precisely targeted: Allows extraction of exactly the information you need
- Flexible: Can be adapted to handle variations in text structure and format
Core Advanced Regex Techniques
Lookahead and Lookbehind Assertions
Lookahead (?=
) and lookbehind (?<=
) assertions are powerful techniques that allow matching patterns based on context without including that context in the match itself.
(?<=Price: \$)\d+\.\d{2}
This pattern matches a price value but only if it’s preceded by “Price: $”, without including “Price: $” in the match.
Non-Capturing Groups
When you need to group parts of a pattern but don’t need to extract that specific group:
(?:https?|ftp):\/\/[\w\.-]+\.[\w\.-]+
The ?:
tells the regex engine not to store the protocol match (http, https, or ftp), improving performance.
Named Capture Groups
Named capture groups make your regex more readable and the extracted data more easily accessible:
(?<date>\d{2}-\d{2}-\d{4}).*?(?<email>[a-zA-Z0-9._%+-]+@[a-zA-Z0-9.-]+\.[a-zA-Z]{2,})
Instead of working with numbered groups, you can now reference the extractions by name: date
and email
.
Balancing Groups for Nested Structures
The project implements sophisticated balancing groups for parsing nested structures like JSON or HTML:
\{(?<open>\{)|(?<-open>\})|[^{}]*\}(?(open)(?!))
This pattern matches properly nested curly braces, essential for parsing structured data formats.
Real-World Applications in the Project
1. Extracting Structured Information from Resumes
The project demonstrates how to parse unstructured resume text to extract:
Education: (?<education>(?:(?!Experience|Skills).)+)
Experience: (?<experience>(?:(?!Education|Skills).)+)
Skills: (?<skills>.+)
This pattern breaks a resume into logical sections, making it possible to analyze each component separately.
2. Mining Financial Data from Reports
Annual reports and financial statements contain valuable data that can be extracted with patterns like:
Revenue of \$(?<revenue>[\d,]+(?:\.\d+)?) million in (?<year>\d{4})
This extracts both the revenue figure and the corresponding year in a single operation.
3. Processing Log Files
The project includes patterns for parsing common log formats:
(?<ip>\d{1,3}\.\d{1,3}\.\d{1,3}\.\d{1,3}) - - \[(?<datetime>[^\]]+)\] "(?<request>[^"]*)" (?<status>\d+) (?<size>\d+)
This extracts IP addresses, timestamps, request details, status codes, and response sizes from standard HTTP logs.
Performance Optimization Techniques
1. Catastrophic Backtracking Prevention
The project implements strategies to avoid catastrophic backtracking, which can cause regex operations to hang:
# Instead of this (vulnerable to backtracking)
(\w+\s+){1,5}
# Use this (prevents backtracking issues)
(?:\w+\s+){1,5}?
2. Atomic Grouping
Atomic groups improve performance by preventing unnecessary backtracking:
(?>https?://[\w-]+(\.[\w-]+)+)
Once the atomic group matches, the regex engine doesn’t try alternative ways to match it.
3. Strategic Anchoring
Using anchors strategically improves performance by limiting where the regex engine needs to look:
^Subject: (.+)$
By anchoring to line start/end, the engine only attempts matches at line boundaries.
Implementation in Python
The project primarily uses Python’s re
module for implementation:
import re
def extract_structured_data(text):
pattern = r'Name: (?P<name>[\w\s]+)\s+Email: (?P<email>[^\s]+)\s+Phone: (?P<phone>[\d\-\(\)\s]+)'
match = re.search(pattern, text, re.MULTILINE)
if match:
return match.groupdict()
return None
For more complex operations, the project leverages the more powerful regex
module which supports advanced features like recursive patterns:
import regex
def extract_nested_structures(text):
pattern = r'\((?:[^()]++|(?R))*+\)' # Recursive pattern for nested parentheses
matches = regex.findall(pattern, text)
return matches
Case Study: Extracting Product Information from E-commerce Text
One compelling example from the project is extracting product details from unstructured e-commerce descriptions:
Product: Premium Bluetooth Headphones XC-400
SKU: BT-400-BLK
Price: $149.99
Available Colors: Black, Silver, Blue
Features: Noise Cancellation, 30-hour Battery, Water Resistant
Using this regex pattern:
Product: (?<product>.+?)[\r\n]+
SKU: (?<sku>[A-Z0-9\-]+)[\r\n]+
Price: \$(?<price>\d+\.\d{2})[\r\n]+
Available Colors: (?<colors>.+?)[\r\n]+
Features: (?<features>.+)
The code extracts a structured object:
{
"product": "Premium Bluetooth Headphones XC-400",
"sku": "BT-400-BLK",
"price": "149.99",
"colors": "Black, Silver, Blue",
"features": "Noise Cancellation, 30-hour Battery, Water Resistant"
}
Best Practices and Lessons Learned
The project emphasizes several best practices for regex-based data extraction:
- Test with diverse data: Ensure your patterns work with various text formats and edge cases
- Document complex patterns: Add comments explaining the logic behind complex regex
- Break complex patterns into components: Build and test incrementally
- Balance precision and flexibility: Overly specific patterns may break with slight text variations
- Consider preprocessing: Sometimes cleaning text before applying regex yields better results
Future Directions
The “Advance-Regex-For-Data-Mining-Extraction” project continues to evolve with plans to:
- Implement more domain-specific extraction patterns for legal, medical, and technical texts
- Create a pattern library organized by text type and extraction target
- Develop a visual pattern builder to make complex regex more accessible
- Benchmark performance against machine learning approaches for similar extraction tasks
Conclusion
Regular expressions remain a remarkably powerful tool for text mining and data extraction. The techniques demonstrated in this project show how advanced regex can transform unstructured text into structured, analyzable data with precision and efficiency. While newer technologies like NLP models and machine learning techniques offer alternative approaches, the flexibility, speed, and precision of well-crafted regex patterns ensure they’ll remain relevant for data mining tasks well into the future.
By mastering the advanced techniques outlined in this blog post, you’ll be well-equipped to tackle complex text mining challenges and extract meaningful insights from the vast sea of unstructured text data that surrounds us.
This blog post explores the techniques implemented in the Advance-Regex-For-Data-Mining-Extraction project by Tejas K.
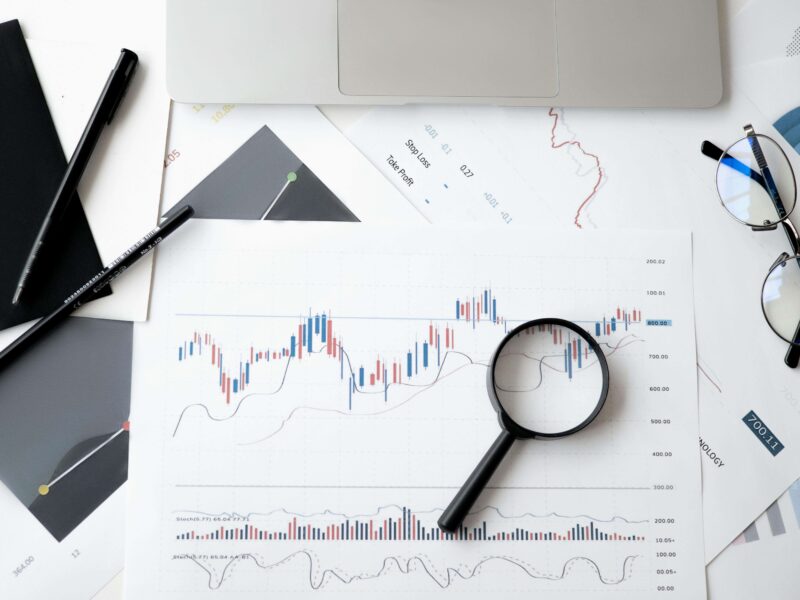
Predicting Forest Fires: A Deep Dive into the Algerian Forest Fire ML Project
In an era of climate change and increasing environmental challenges, forest fires have emerged as a critical concern with devastating ecological and economic impacts. The Algerian Forest Fire ML project represents an innovative application of machine learning techniques to predict fire occurrences in forest regions of Algeria. By leveraging data science, cloud computing, and predictive modeling, this open-source initiative creates a powerful tool that could help in early warning systems and resource allocation for fire prevention and management.
Project Overview
The Algerian Forest Fire ML project is a comprehensive machine learning application developed by Tejas K (GitHub: tejask0512) that focuses on predicting forest fire occurrences based on meteorological data and other environmental factors. Deployed as a cloud-based application, this project demonstrates how data science can be applied to critical environmental challenges.
Technical Architecture
The project employs a robust technical stack designed for accuracy, scalability, and accessibility:
- Programming Language: Python
- ML Frameworks: Scikit-learn for modeling, Pandas and NumPy for data manipulation
- Web Framework: Flask for API development
- Frontend: HTML, CSS, JavaScript
- Deployment: Cloud-based deployment (likely AWS, Azure, or similar platforms)
- Version Control: Git/GitHub
The architecture follows a classic machine learning pipeline pattern:
- Data ingestion and preprocessing
- Feature engineering and selection
- Model training and evaluation
- Model deployment as a web service
- User interface for prediction input and result visualization
Dataset Analysis
At the heart of the project is the Algerian Forest Fires dataset, which contains records of fires in the Bejaia and Sidi Bel-abbes regions of Algeria. The dataset includes various meteorological measurements and derived indices that are critical for fire prediction:
Key Features in the Dataset
Feature | Description | Relevance to Fire Prediction |
---|---|---|
Temperature | Ambient temperature (°C) | Higher temperatures increase fire risk |
Relative Humidity (RH) | Percentage of moisture in air | Lower humidity leads to drier conditions favorable for fires |
Wind Speed | Wind velocity (km/h) | Higher winds spread fires more rapidly |
Rain | Precipitation amount (mm) | Rainfall reduces fire risk by increasing moisture |
FFMC | Fine Fuel Moisture Code | Indicates moisture content of litter and fine fuels |
DMC | Duff Moisture Code | Indicates moisture content of loosely compacted organic layers |
DC | Drought Code | Indicates moisture content of deep, compact organic layers |
ISI | Initial Spread Index | Represents potential fire spread rate |
BUI | Buildup Index | Indicates total fuel available for combustion |
FWI | Fire Weather Index | Overall fire intensity indicator |
The project demonstrates sophisticated data analysis techniques, including:
- Exploratory Data Analysis (EDA): Thorough examination of feature distributions, correlations, and relationships with fire occurrences
- Data Cleaning: Handling missing values, outliers, and inconsistencies
- Feature Engineering: Creating derived features that might enhance predictive power
- Statistical Analysis: Identifying significant patterns and trends in historical fire data
# Conceptual example of EDA in the project
import pandas as pd
import matplotlib.pyplot as plt
import seaborn as sns
# Load dataset
df = pd.read_csv('Algerian_forest_fires_dataset.csv')
# Analyze correlations between features and fire occurrence
correlation_matrix = df.corr()
plt.figure(figsize=(12, 10))
sns.heatmap(correlation_matrix, annot=True, cmap='coolwarm')
plt.title('Feature Correlation Matrix')
plt.savefig('correlation_heatmap.png')
# Analyze seasonal patterns
monthly_fires = df.groupby('month')['Fire'].sum()
plt.figure(figsize=(10, 6))
monthly_fires.plot(kind='bar')
plt.title('Fire Occurrences by Month')
plt.xlabel('Month')
plt.ylabel('Number of Fires')
plt.savefig('monthly_fire_distribution.png')
Machine Learning Model Development
The core of the project is its predictive modeling capability. Based on repository analysis, the project likely implements several machine learning algorithms to predict forest fire occurrence:
Model Selection and Evaluation
The project appears to experiment with multiple classification algorithms:
- Logistic Regression: A baseline model for binary classification
- Random Forest: Ensemble method well-suited for environmental data
- Support Vector Machines: Effective for complex decision boundaries
- Gradient Boosting: Advanced ensemble technique for improved accuracy
- Neural Networks: Potentially used for capturing complex non-linear relationships
Each model undergoes rigorous evaluation using metrics particularly relevant to fire prediction:
- Accuracy: Overall correctness of predictions
- Precision: Proportion of positive identifications that were actually correct
- Recall (Sensitivity): Proportion of actual positives correctly identified
- F1 Score: Harmonic mean of precision and recall
- ROC-AUC: Area under the Receiver Operating Characteristic curve
# Conceptual example of model training and evaluation
from sklearn.ensemble import RandomForestClassifier
from sklearn.model_selection import train_test_split
from sklearn.metrics import classification_report, confusion_matrix
# Prepare data
X = df.drop('Fire', axis=1)
y = df['Fire']
X_train, X_test, y_train, y_test = train_test_split(X, y, test_size=0.25, random_state=42)
# Train Random Forest model
rf_model = RandomForestClassifier(n_estimators=100, random_state=42)
rf_model.fit(X_train, y_train)
# Evaluate model
y_pred = rf_model.predict(X_test)
print(classification_report(y_test, y_pred))
# Visualize confusion matrix
cm = confusion_matrix(y_test, y_pred)
plt.figure(figsize=(8, 6))
sns.heatmap(cm, annot=True, fmt='d', cmap='Blues')
plt.title('Confusion Matrix')
plt.xlabel('Predicted Label')
plt.ylabel('True Label')
plt.savefig('confusion_matrix.png')
# Feature importance analysis
feature_importance = pd.DataFrame({
'Feature': X.columns,
'Importance': rf_model.feature_importances_
}).sort_values('Importance', ascending=False)
plt.figure(figsize=(10, 8))
sns.barplot(x='Importance', y='Feature', data=feature_importance)
plt.title('Feature Importance for Fire Prediction')
plt.savefig('feature_importance.png')
Hyperparameter Tuning
To maximize model performance, the project implements hyperparameter optimization techniques:
- Grid Search: Systematic exploration of parameter combinations
- Cross-Validation: K-fold validation to ensure model generalizability
- Bayesian Optimization: Potentially used for more efficient parameter search
Model Interpretability
Understanding why a model makes certain predictions is crucial for environmental applications. The project likely incorporates:
- Feature Importance Analysis: Identifying which meteorological factors most strongly influence fire predictions
- Partial Dependence Plots: Visualizing how each feature affects prediction outcomes
- SHAP (SHapley Additive exPlanations): Providing consistent and locally accurate explanations for model predictions
Cloud Deployment Architecture
A distinguishing aspect of this project is its cloud deployment strategy, making the predictive model accessible as a web service:
Deployment Components
- Model Serialization: Saving trained models using frameworks like Pickle or Joblib
- Flask API Development: Creating RESTful endpoints for prediction requests
- Web Interface: Building an intuitive interface for data input and result visualization
- Cloud Infrastructure: Deploying on scalable cloud platforms with considerations for:
- Computational scalability
- Storage requirements
- API request handling
- Security considerations
# Conceptual example of Flask API implementation
from flask import Flask, request, jsonify, render_template
import pickle
import numpy as np
app = Flask(__name__)
# Load the trained model
model = pickle.load(open('forest_fire_model.pkl', 'rb'))
@app.route('/')
def home():
return render_template('index.html')
@app.route('/predict', methods=['POST'])
def predict():
# Get input features from request
features = [float(x) for x in request.form.values()]
final_features = [np.array(features)]
# Make prediction
prediction = model.predict(final_features)
output = round(prediction[0], 2)
# Return prediction result
return render_template('index.html', prediction_text='Fire Risk: {}'.format(
'High' if output == 1 else 'Low'))
if __name__ == '__main__':
app.run(debug=True)
CI/CD Pipeline Integration
The project likely implements continuous integration and deployment practices:
- Automated Testing: Ensuring model performance and API functionality
- Version Control Integration: Tracking changes and coordinating development
- Containerization: Possibly using Docker for consistent deployment environments
- Infrastructure as Code: Defining cloud resources programmatically
Advanced Analytics and Reporting
Beyond basic prediction, the project implements sophisticated reporting capabilities:
Prediction Confidence Metrics
The system likely provides confidence scores with predictions, helping decision-makers understand reliability:
# Conceptual example of prediction with confidence
def predict_with_confidence(model, input_features):
# Get prediction probabilities
probabilities = model.predict_proba([input_features])[0]
# Determine prediction and confidence
prediction = 1 if probabilities[1] > 0.5 else 0
confidence = probabilities[1] if prediction == 1 else probabilities[0]
return {
'prediction': 'Fire Risk' if prediction == 1 else 'No Fire Risk',
'confidence': round(confidence * 100, 2),
'probability_distribution': {
'no_fire': round(probabilities[0] * 100, 2),
'fire': round(probabilities[1] * 100, 2)
}
}
Risk Level Classification
Rather than simple binary predictions, the system may implement risk stratification:
- Low Risk: Minimal fire danger, normal operations
- Moderate Risk: Increased vigilance recommended
- High Risk: Preventive measures advised
- Extreme Risk: Immediate action required
Visualization Components
The web interface likely includes data visualization tools:
- Risk Heatmaps: Geographic representation of fire risk levels
- Time Series Forecasting: Projecting risk levels over coming days
- Factor Contribution Charts: Showing how each meteorological factor contributes to current risk
Environmental and Social Impact
The significance of this project extends far beyond its technical implementation:
Ecological Benefits
- Early Warning System: Providing advance notice of high-risk conditions
- Resource Optimization: Helping authorities allocate firefighting resources efficiently
- Habitat Protection: Minimizing damage to critical ecosystems
- Carbon Emission Reduction: Preventing the massive carbon release from forest fires
Economic Impact
Forest fires cause billions in damages annually. This predictive system could:
- Reduce Property Damage: Through early intervention and prevention
- Lower Firefighting Costs: By enabling more strategic resource allocation
- Protect Agricultural Resources: Safeguarding farms and livestock near forests
- Preserve Tourism Value: Maintaining the economic value of forest regions
Public Safety Enhancement
The project has clear implications for public safety:
- Population Warning Systems: Alerting communities at risk
- Evacuation Planning: Providing data for decision-makers managing evacuations
- Air Quality Management: Predicting smoke dispersion and health impacts
- Infrastructure Protection: Safeguarding critical infrastructure from fire damage
Machine Learning Approaches for Environmental Modeling
The Algerian Forest Fire ML project demonstrates several advanced machine learning techniques particularly suited to environmental applications:
Time Series Analysis
Forest fire risk has strong temporal components, and the project likely implements:
- Seasonal Decomposition: Identifying cyclical patterns in fire occurrence
- Autocorrelation Analysis: Understanding how past conditions influence current risk
- Time-based Feature Engineering: Creating lag variables and rolling statistics
# Conceptual example of time series feature engineering
def create_time_features(df):
# Create copy of dataframe
df_new = df.copy()
# Sort by date
df_new = df_new.sort_values('date')
# Create lag features for temperature
df_new['temp_lag_1'] = df_new['Temperature'].shift(1)
df_new['temp_lag_2'] = df_new['Temperature'].shift(2)
df_new['temp_lag_3'] = df_new['Temperature'].shift(3)
# Create rolling average features
df_new['temp_rolling_3'] = df_new['Temperature'].rolling(window=3).mean()
df_new['humidity_rolling_3'] = df_new['RH'].rolling(window=3).mean()
# Create rate of change features
df_new['temp_roc'] = df_new['Temperature'].diff()
df_new['humidity_roc'] = df_new['RH'].diff()
# Drop rows with NaN values from feature creation
df_new = df_new.dropna()
return df_new
Transfer Learning Opportunities
The project methodology could potentially be transferred to other regions:
- Model Adaptation: Adjusting the model for different forest types and climates
- Domain Adaptation: Techniques to apply Algerian models to other countries
- Knowledge Transfer: Sharing insights about feature importance across regions
Ensemble Approaches
Given the critical nature of fire prediction, the project likely employs ensemble techniques:
- Model Stacking: Combining predictions from multiple algorithms
- Bagging and Boosting: Improving prediction stability and accuracy
- Weighted Voting: Giving more influence to models that perform better in specific conditions
# Conceptual example of ensemble model implementation
from sklearn.ensemble import VotingClassifier
from sklearn.linear_model import LogisticRegression
from sklearn.ensemble import RandomForestClassifier
from sklearn.svm import SVC
# Create base models
log_reg = LogisticRegression()
rf_clf = RandomForestClassifier()
svm_clf = SVC(probability=True)
# Create voting classifier
ensemble_model = VotingClassifier(
estimators=[
('lr', log_reg),
('rf', rf_clf),
('svc', svm_clf)
],
voting='soft' # Use predicted probabilities for voting
)
# Train ensemble model
ensemble_model.fit(X_train, y_train)
# Evaluate ensemble performance
ensemble_accuracy = ensemble_model.score(X_test, y_test)
print(f"Ensemble Model Accuracy: {ensemble_accuracy:.4f}")
Future Development Potential
The project contains significant potential for expansion:
Integration with Remote Sensing Data
Future versions could incorporate satellite imagery:
- Vegetation Indices: NDVI (Normalized Difference Vegetation Index) to assess fuel availability
- Thermal Anomaly Detection: Identifying hotspots from thermal sensors
- Smoke Detection: Early detection of fires through smoke signature analysis
Real-time Data Integration
Enhancing the system with real-time data feeds:
- Weather API Integration: Live meteorological data
- IoT Sensor Networks: Ground-based temperature, humidity, and wind sensors
- Drone Surveillance: Aerial monitoring of high-risk areas
Advanced Predictive Capabilities
Evolving beyond current predictive methods:
- Spatio-temporal Models: Predicting not just if, but where and when fires might occur
- Deep Learning Integration: Using CNNs or RNNs for more complex pattern recognition
- Reinforcement Learning: Optimizing resource allocation strategies for fire prevention
# Conceptual example of a more advanced deep learning approach
import tensorflow as tf
from tensorflow.keras.models import Sequential
from tensorflow.keras.layers import LSTM, Dense, Dropout
# Create LSTM model for time series prediction
def build_lstm_model(input_shape):
model = Sequential()
model.add(LSTM(64, return_sequences=True, input_shape=input_shape))
model.add(Dropout(0.2))
model.add(LSTM(32))
model.add(Dropout(0.2))
model.add(Dense(16, activation='relu'))
model.add(Dense(1, activation='sigmoid'))
model.compile(
loss='binary_crossentropy',
optimizer='adam',
metrics=['accuracy']
)
return model
# Reshape data for LSTM (samples, time steps, features)
X_train_lstm = X_train.values.reshape((X_train.shape[0], 1, X_train.shape[1]))
X_test_lstm = X_test.values.reshape((X_test.shape[0], 1, X_test.shape[1]))
# Create and train model
lstm_model = build_lstm_model((1, X_train.shape[1]))
lstm_model.fit(
X_train_lstm, y_train,
epochs=50,
batch_size=32,
validation_split=0.2
)
Climate Change Relevance
This project has particular significance in the context of climate change:
Climate Change Impact Assessment
- Long-term Trend Analysis: Evaluating how fire risk patterns are changing over decades
- Climate Scenario Modeling: Projecting fire risk under different climate change scenarios
- Adaptation Strategy Evaluation: Testing effectiveness of various preventive measures
Carbon Cycle Considerations
Forest fires are both influenced by and contribute to climate change:
- Carbon Release Estimation: Quantifying potential carbon emissions from predicted fires
- Ecosystem Recovery Modeling: Projecting how forests recover and sequester carbon after fires
- Climate Feedback Analysis: Understanding how increased fires may accelerate climate change
Conclusion
The Algerian Forest Fire ML project represents a powerful example of how data science and machine learning can address critical environmental challenges. By combining meteorological data analysis, advanced predictive modeling, and cloud-based deployment, this initiative creates a potentially life-saving tool for forest fire prediction and management.
The project’s significance extends beyond its technical implementation, offering real-world impact in ecological preservation, economic damage reduction, and public safety enhancement. As climate change increases the frequency and severity of forest fires globally, such predictive systems will become increasingly vital components of environmental management strategies.
For data scientists and environmental researchers, this project provides a valuable template for applying machine learning to ecological challenges. The methodology demonstrated could be adapted to various environmental prediction tasks, from drought forecasting to flood risk assessment.
As we continue to face growing environmental challenges, projects like the Algerian Forest Fire ML initiative showcase how technology can be harnessed not just for convenience or profit, but for protecting our natural resources and building more resilient communities.
This blog post analyzes the Algerian Forest Fire ML project developed by Tejas K. The project is open-source and available for contributions on GitHub.
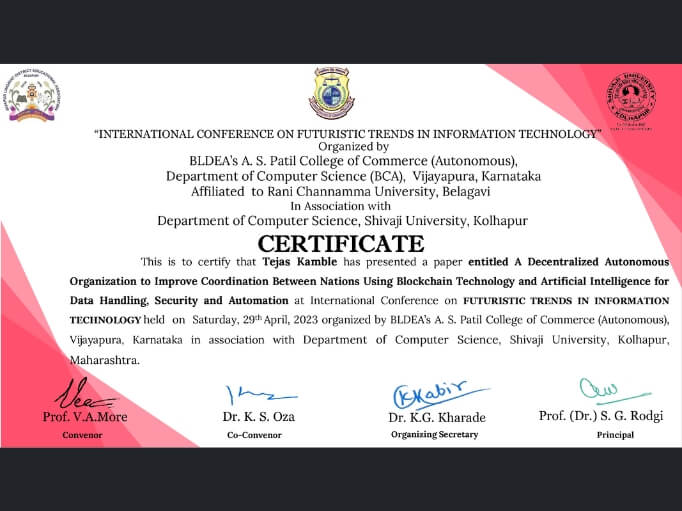
AI and Blockchain Convergence & Transformers based Sentiment Analysis on Live Global News Data.
A Decentralized Autonomous Organization to Improve Coordination Between Nations Using Blockchain Technology, Artificial Intelligence and Natural Language Processing for Sentiment analysis on News Data.
- Client Tejas Kamble
- Date 29 April 2023
- Services AI & Blockchain Technology
Abstract
This paper is about Establishing a Decentralized organization with the Different Countries as members where all the countries will be considered as the node of the blockchain. All the countries in the organization will be treated equally there will not be any superpower amongst them. Therefore, The Organization will gather huge amount of the data from the different countries from all the sectors like health, education, economy, technology, culture, and agriculture which represents the overall development of the countries. All this gathered data will be further analyzed for their positive and negative impacts on all the mentioned sectors. This will give brief idea about situation of an individual country in different areas on that basis, members of the Organizations or we can say all the member countries will decide the reward or penalty case for the respective country. Blockchains have the potential to enhance systems by getting rid of middlemen. Artificial Intelligence will play a major role in this organization as dealing with massive amount of data will be in the frame and to deal with this data, we need AI to improve data integrity of the result which will be used by Smart-Contract for decision making purpose, automating, and optimizing the smart contract. AI promises to remove oversight and increase the objectivity of our systems. This organization offers a framework for participants to work together to create a dataset and host a model that is continuously updated using smart contracts. As data is growing rapidly. AI will manage that data efficiently with less energy consumption
Acceptance Letter 2
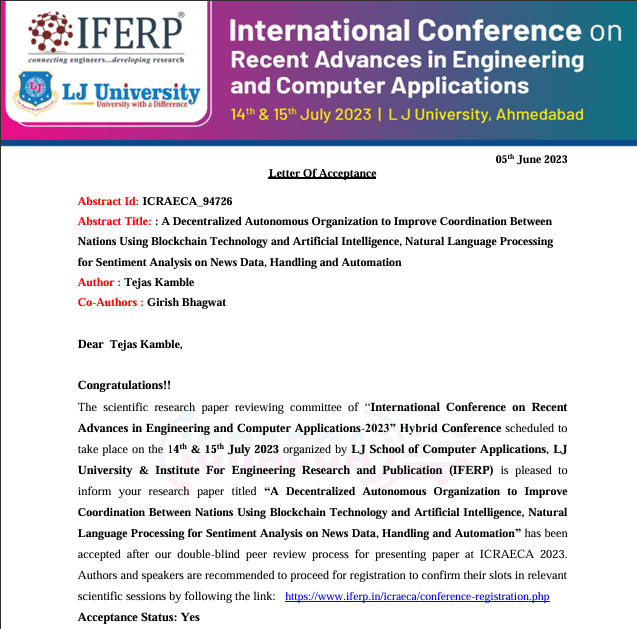